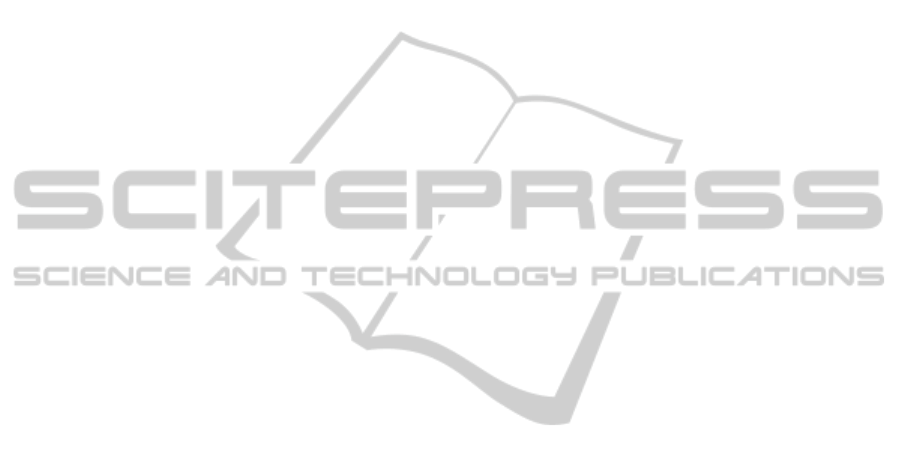
operations without the requirement for a drastic re-
structuring of the system.
3.3 Reasoning & Inference
Using an ontology model to combine condition mon-
itoring data with railway operations provides the po-
tential for autonomous reasoning, removing the ne-
cessity for human operators to spend large amounts
of time cross-referencing and checking raw data in
order to determine and describe vehicle/infrastructure
faults. This is likely to be a key benefit to railway op-
erators, and an aspect of the system which will be de-
veloped in depth. A reasoner will allow, for example,
the association between a vehicle’s geographic loca-
tion and an RCM fault value to be done automatically.
This is one of the key benefits of using an ontology-
based system to integrate data across the railway, and
knowledge inferred through reasoning techniques is
likely to be of great value to railway operators and
other stakeholders.
4 A WILD ONTOLOGY
Initially, the development of a new ontology system
to address the data integration issues discussed above
will aim to capture informationfrom the Network Rail
WILD axle load monitoring system and combine it
with train running data (such as vehicle locations and
descriptions). It is proposed that such an ontology
include knowledge of several aspects of the railway
domain, as follows:
• Railway geography and network layout, in order
to locate vehicles on track and RCM sensors. In-
vensys Rail’s WestCAD system tracks train lo-
cations in discrete ’bays’ along the track, based
on data from railway track circuits or axel coun-
ters; the WestCAD data, or even track circuit data
could be used to locate trains in an ontology.
• Railway vehicle knowledge. Train headcodes
contain limited information about vehicle class
(freight, passenger, high speed services); this in-
formation should be represented as such, with
the possibility of being expanded upon using data
available from other systems (in-cab diagnostics,
train operator data).
• RCM knowledge such as WILD raw data, passing
vehicle type, fault conditions, equipment charac-
teristics, and equipment geography.
With these aspects correctly modelled, it is possible
that rules created in an ontology reasoner will allow
WILD data to be correlated to railway vehicles, and
could also associate vehicle types with common line
faults (for instance train bogie designs that are less
reliable than others). Train speed and weight as ac-
quired by the WILD system will also form part of
the ontology, allowing speed profiling of services over
long periods of time to be performed. Creation of this
data model will allow the retrieval of WILD infor-
mation with regards to specific trains, automatically,
and will thus save the expense of needing human re-
sources to verify all incoming RCM data. The even-
tual use of this system will add value to existing IRG
products, and continued development of the ontology
system should allow greater utilisation of the technol-
ogy in the railway domain.
4.1 Future Development
It is important that the WILD system as described
above be modelled in such a way that further develop-
ment is possible, in order that many more sub-systems
can be included and further knowledge inferred. It
is the project’s aim to use this ontology application
as a basis for a larger railway systems ontology over
the next two years, showing that further streamlining
of railway data can be achieved across control, op-
erations, and maintenance of the network. Further
work will involve the inclusion of other railway sys-
tems data in the ontology, such as recorded vehicle
’black box’ data (many trains record speed, location,
and diagnostics information as they travel), and com-
prehensive timetable information. With the addition
of these data sets, an ontology system will be able to
perform further reasoning in order to contribute new
knowledge to railway stakeholders.
REFERENCES
AEA Technology (2008). Wheelchex user guide.
Langer, G., Lewis, R., and Roberts, C. (2008). Ontology
driven railway rcm data integration. In Railway Con-
dition Monitoring, 2008 4th IET International Confer-
ence on.
Lewis, R., Fuchs, F., Pirker, M., Roberts, C., and Langer, G.
(2006). Using ontology to integrate railway condition
monitoring data. In Railway Condition Monitoring,
2006. The Institution of Engineering and Technology
International Conference on, pages 149 –155.
Network Rail (2010). Catalogue of railway code systems.
Office of Rail Regulations (2010). National rail trends
2009-10 yearbook.
Ollier, B. D. (2006). Intelligent infrastructure the business
challenge. In Railway Condition Monitoring, 2006.
The Institution of Engineering and Technology Inter-
national Conference on, pages 1 –6.
KEOD 2011 - International Conference on Knowledge Engineering and Ontology Development
444