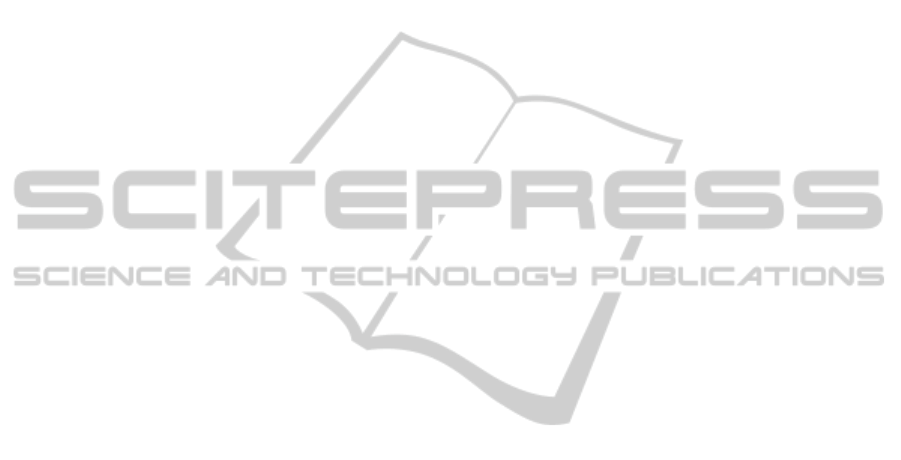
our framework. For further analysis of structured
data we choose to incorporate OLAP analysis as it is
a widely accepted technique for viewing data
alongside different perspectives (dimensions).
We conclude that this framework can already be
useful as a structured guide or reference, but leaving
ample room for improvement and the
implementation of new or existing techniques.
REFERENCES
Adomavicius, G., & Tuzhilin, A. (2002). Using data
mining methods to build customer profiles. Computer,
34(2), 74-82.
Anderson, P. (2007). What is web 2.0. Ideas, technologies
and implications for education, 60, 1-10.
Chung, W., Chen, H., & Numumaker Jr, J. F. (2003).
Business Intelligence Explorer: A knowledge map
framework for discovering business intelligence on the
Web. Proceedings of the 36th Annual Hawaii
International Conference on System Sciences (pp. 1-
10). Honolulu, Hawaii, USA: IEEE.
Ding, X., Liu, B., & Yu, P. S. (2008). A holistic lexicon-
based approach to opinion mining. Proceedings of the
international conference on Web search and web data
mining (pp. 231-240). Stanford, California, United
States of America: ACM.
Erétéo, G., Buffa, M., Gandon, F., Grohan, P., Leitzelman,
M., & Sander, P. (2008). A state of the art on social
network analysis and its applications on a semantic
web. Proceedings of the 7th International Semantic
Web Conference (pp. 1-6). Karlsruhe, Germany:
Citeseer.
Feldman, R., & Dagan, I. (1995). Knowledge discovery in
textual databases (KDT). Proceedings of the First
International Conference on Knowledge Discovery
and Data Mining (pp. 112-117). Montreal, Quebec,
Canada: ACM.
Feldman, R., & Sanger, J. (2007). The text mining
handbook: advanced approaches in analyzing
unstructured data. New York: Cambridge University
Press.
Heer, J., & Boyd, D. (2005). Vizster: Visualizing online
social networks. Proceedings of the 2005 IEEE
Symposium on Information Visualization (pp. 1-5).
Minneapolis, Minnesota, USA: IEEE Computer
Society.
Hu, M., & Liu, B. (2004). Mining and summarizing
customer reviews. Proceedings of the tenth ACM
SIGKDD international conference on Knowledge
discovery and data mining (pp. 168-177). ACM.
Inmon, W. H. (2002). Building the Data Warehouse (3rd
ed.). New York: Wiley.
Kleinberg, J. (2008). The convergence of social and
technological network. Communications of the ACM,
51(11), 66-72.
Kotsiantis, S., & Kanellopoulos, D. (2006). Association
rules mining: A recent overview. GESTS International
Transactions on Computer Science and Engineering,
32(1), 71-82.
Liu, B. (2007). Opinion Mining. In B. Lui, & B. Liu (Ed.),
Web data mining: Exploring hyperlinks, contents, and
usage data (pp. 411-447). Springer.
Moody, D. L., & Kortink, M. A. (2000). From enterprise
models to dimensional models: a methodology for data
warehouse and data mart design. Proceedings of the
Second International Workshop on Design and
Management. 28, pp. 1-12. Stockholm, Sweden:
Citeseer.
och Dag, N., Regnell, B., Gervasi, V., & Brinkkemper, S.
(2005). A linguistic-engineering approach to large-
scale requirements management. Software, IEEE,
22(1), 32-39.
Pang, B., & Lee, L. (2008). Opinion mining and sentiment
analysis. Foundations and Trends in Information
Retrieval, 2(1), 1-135.
Parmenter, D. (2007). Key Performance Indicators.
Hoboken, New Jersey, USA: John Wiley & Sons.
Sen, A., & Sinha, A. P. (2005). A comparison of data
warehousing methodologies.
Communications of the
ACM, 48(3), 79-84.
Willen, C. (2002). Airborne opportunities. Intelligent
Enterprise, 5(2), 11-12.
Yang, W. S., & Dia, J. B. (2008). Discovering cohesive
subgroups from social networks for targeted
advertising. Expert Systems with Applications, 34(3),
2029-2038.
Yang, W. S., Dia, J. B., Cheng, H. C., & Lin, H. T. (2006).
Mining social networks for targeted advertising.
Proceedings of the 39th Annual Hawaii International
Conference on System Sciences (pp. 1-10). Honolulu,
Hawaii, United States of America: IEEE.
LINGUISTIC ENGINEERING AND ITS APPLICABILITY TO BUSINESS INTELLIGENCE - Towards an Integrated
Framework
463