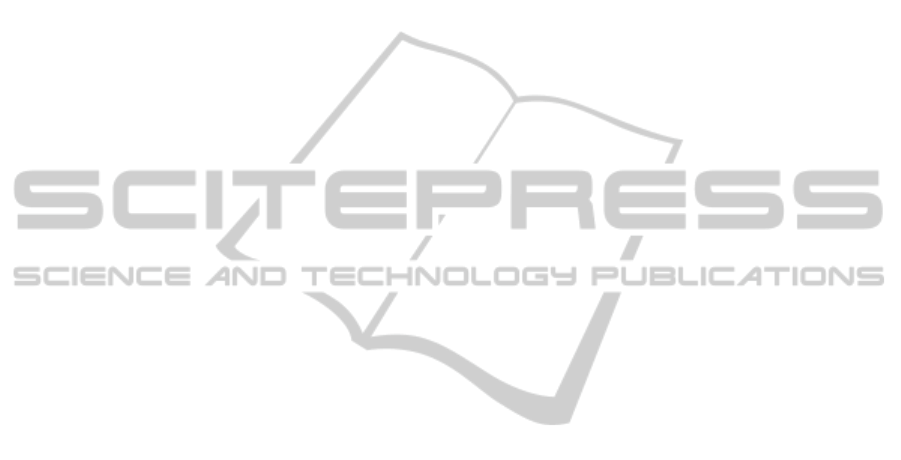
provided, with the reduced TC representation, quite
interesting rules characterizing subgroups of one drug
category versus another one. Complementary experi-
ments can now be carried out to identify rules specific
of a given category versus all other categories.
REFERENCES
Alcala-Fdez, J., Snchez, L., Garca, S., del Jesus, M., Ven-
tura, S., Garrell, J., Otero, J., Romero, C., Bacardit,
J., Rivas, V., Fernndez, J., and Herrera, F. (2009).
KEEL: a software tool to assess evolutionary algo-
rithms for data mining problems. Soft Computing -
A Fusion of Foundations, Methodologies and Appli-
cations, 13(3):307–318–318.
Benabderrahmane, S., Smail-Tabbone, M., Poch, O.,
Napoli, A., and Devignes, M. (2010). IntelliGO: a new
vector-based semantic similarity measure including
annotation origin. BMC Bioinformatics, 11(1):588.
Coulet, A., Smail-Tabbone, M., Benlian, P., Napoli, A., and
Devignes, M. (2008). Ontology-guided data prepa-
ration for discovering genotype-phenotype relation-
ships. BMC Bioinformatics, 9(Suppl 4):S3.
Dy, J. G. and Brodley, C. E. (2004). Feature selection for
unsupervised learning. J. Mach. Learn. Res., 5:845–
889.
Guyon, I. and Elisseeff, A. (2003). An introduction to
variable and feature selection. J. Mach. Learn. Res.,
3:1157–1182.
Han, J. and Kamber, M. (2001). Data Mining: Concepts
and Techniques. Morgan Kaufmann, San Francisco, 1
edition.
John, G. H., Kohavi, R., and Pfleger, K. (1994). Irrelevant
Features and the Subset Selection Problem. In In-
ternational Conference on Machine Learning, pages
121–129.
Kaytoue-Uberall, M., Duplessis, S., Kuznetsov, S. O., and
Napoli, A. (2009). Two fca-based methods for mining
gene expression data. In ICFCA, pages 251–266.
Kelley, L. A., Gardner, S. P., and Sutcliffe, M. J. (1996).
An automated approach for clustering an ensemble
of NMR-derived protein structures into conforma-
tionally related subfamilies. Protein Engineering,
9(11):1063 –1065.
Kim, Y., Street, W. N., and Menczer, F. (2000). Feature
selection in unsupervised learning via evolutionary
search. In Proceedings of the sixth ACM SIGKDD in-
ternational conference on Knowledge discovery and
data mining, pages 365–369, Boston, Massachusetts,
United States. ACM.
Knox, C., Law, V., Jewison, T., Liu, P., Ly, S., Frolkis, A.,
Pon, A., Banco, K., Mak, C., Neveu, V., Djoumbou,
Y., Eisner, R., Guo, A. C., and Wishart, D. S. (2011).
DrugBank 3.0: a comprehensive resource for Omics
research on drugs. Nucleic Acids Research, 39(suppl
1):D1035 –D1041.
Kohavi, R. and John, G. H. (1997). Wrappers for feature
subset selection. Artificial Intelligence, 97(1-2):273–
324.
Koller, D. and Sahami, M. (1996). Toward optimal feature
selection. In Saitta, L., editor, Proceedings of the Thir-
teenth International Conference on Machine Learning
(ICML), pages 284–292. Morgan Kaufmann Publish-
ers.
Kuhn, M., Campillos, M., Letunic, I., Jensen, L. J., and
Bork, P. (2010). A side effect resource to capture phe-
notypic effects of drugs. Mol Syst Biol, 6.
Kyriakopoulou, A. (2008). Text classification aided by clus-
tering: a literature review. In Tools in Artificial Intel-
ligence, chapter 14. Paula Fritzsche, intech edition.
Lavrac, N., Kavsek, B., Flach, P., and Todorovski, L.
(2004). Subgroup discovery with CN2-SD. J. Mach.
Learn. Res., 5:153–188.
Leva, A. D., Berchi, R., Pescarmona, G., and Sonnessa,
M. (2005). Analysis and prototyping of biological
systems: the abstract biological process model. In-
ternational Journal of Information and Technology,
3(4):216–224.
MedDRA (2007). Meddra maintenance and support ser-
vices organization. introductory guide, meddra ver-
sion 10.1.
Messai, N., Devignes, M.-D., Napoli, A., and Sma
¨
ıl-
Tabbone, M. (2008). Many-valued concept lattices
for conceptual clustering and information retrieval. In
ECAI, pages 127–131.
Pakhomov, S. S., Hemingway, H., Weston, S. A., Jacob-
sen, S. J., Rodeheffer, R., and Roger, V. L. (2007).
Epidemiology of angina pectoris: Role of natural lan-
guage processing of the medical record. American
Heart Journal, 153(4):666–673.
Slonim, N. and Tishby, N. (2000). Document clustering
using word clusters via the information bottleneck
method. In Proceedings of the 23rd annual interna-
tional ACM SIGIR conference on Research and de-
velopment in information retrieval, pages 208–215,
Athens, Greece. ACM.
Szathmary, L., Napoli, A., and Kuznetsov, S. O. (2007).
Zart: A multifunctional itemset mining algorithm. In
CLA, pages 26–37.
Ward, J. H. (1963). Hierarchical grouping to optimize
an objective function. Journal of the American Sta-
tistical Association, 58(301):236–244. ArticleType:
research-article / Full publication date: Mar., 1963 /
Copyright 1963 American Statistical Association.
Witten, I. H., Frank, E., and Hall, M. A. (2011). Data
Mining: Practical Machine Learning Tools and Tech-
niques. Morgan Kaufmann, Burlington, MA, 3 edi-
tion.
KDIR 2011 - International Conference on Knowledge Discovery and Information Retrieval
276