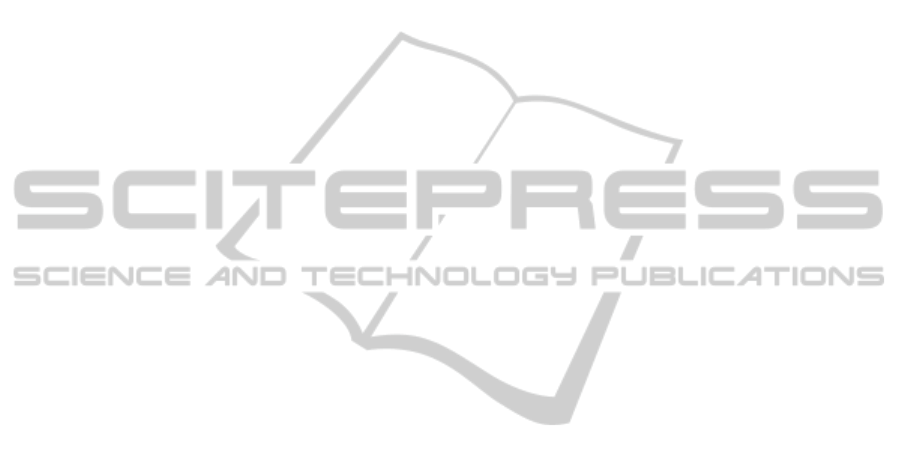
sion and GO semantic similarity. IEEE/ACM Trans.
Comput. Biology Bioinform, 2(4):330–338.
Ding, C. and Peng, H. (2003). Minimum redundancy fea-
ture selection from microarray gene expression data.
In Bioinformatics Conference, 2003. CSB 2003. Pro-
ceedings of the 2003 IEEE, pages 523 – 528.
Duan, K.-B., Rajapakse, J., Wang, H., and Azuaje, F.
(2005). Multiple svm-rfe for gene selection in cancer
classification with expression data. NanoBioscience,
IEEE Transactions on, 4(3):228 –234.
Dudoit, S., Fridlyand, J., and Speed, T. P. (2002). Compar-
ison of discrimination methods for the classification
of tumors using gene expression data. Journal of the
American Statistical Association, 97(457):77.
Efron, B., Tibshirani, R., Storey, J. D., and Tusher, V.
(2001). Empirical Bayes Analysis of a Microarray
Experiment. Journal of the American Statistical As-
sociation, 96(456):1151–1160.
Fox, R. and Dimmic, M. (2006). A two-sample bayesian
t-test for microarray data. BMC Bioinformatics,
7(1):126.
Furey, T. S., Cristianini, N., Duffy, N., Bednarski, D. W.,
Schummer, M., and Haussler, D. (2000). Support vec-
tor machine classification and validation of cancer tis-
sue samples using microarray expression data. Bioin-
formatics, 16(10):906–914.
Golub, T. R., Slonim, D. K., Tamayo, P., Huard, C., Gaasen-
beek, M., Mesirov, J. P., Coller, H., Loh, M. L., Down-
ing, J. R., Caligiuri, M. A., Bloomfield, C. D., and
Lander, E. S. (1999). Molecular Classification of Can-
cer: Class Discovery and Class Prediction by Gene
Expression Monitoring. Science, 286(5439):531–537.
Jafari, P. and Azuaje, F. (2006). An assessment of recently
published gene expression data analyses: reporting
experimental design and statistical factors. BMC Med-
ical Informatics and Decision Making, 6(1):27.
Kumar, P. V., Vinodh, K., An, M., and Elia, P. (2001). The
gene ontology consortium: Creating the gene ontol-
ogy resource: design and implementation. Genome
Res, pages 1425–1433.
Leung, Y. and Hung, Y. (2010). A multiple-filter-multiple-
wrapper approach to gene selection and microarray
data classification. Computational Biology and Bioin-
formatics, IEEE/ACM Transactions on, 7(1):108 –
117.
Li, L., Weinberg, C. R., Darden, T. A., and Pedersen, L. G.
(2001). Gene selection for sample classification based
on gene expression data: study of sensitivity to choice
of parameters of the GA/KNN method. Bioinformat-
ics, 17(12):1131–1142.
Pan, W. (2003). On the use of permutation in and the
performance of a class of nonparametric methods to
detect differential gene expression. Bioinformatics,
19(11):1333–1340.
Papachristoudis, G., Diplaris, S., and Mitkas, P. A. (2010).
Sofocles: Feature filtering for microarray classifica-
tion based on gene ontology. Journal of Biomedical
Informatics, 43(1):1 – 14.
Paul, T. K. and Iba, H. (2009). Prediction of cancer class
with majority voting genetic programming classifier
using gene expression data. Computational Biol-
ogy and Bioinformatics, IEEE/ACM Transactions on,
6(2):353–367.
Pesquita, C., Faria, D., Falco, A. O., Lord, P., and Couto,
F. M. (2009). Semantic similarity in biomedical on-
tologies. PLoS Comput Biol, 5(7):e1000443.
Resnik, P. (1995). Using information content to evaluate
semantic similarity in a taxonomy. In Proceedings of
the 14th international joint conference on Artificial in-
telligence - Volume 1, pages 448–453, San Francisco,
CA, USA. Morgan Kaufmann Publishers Inc.
Saeys, Y., Inza, I. n., and Larra
˜
naga, P. (2007). A review of
feature selection techniques in bioinformatics. Bioin-
formatics (Oxford, England), 23(19):2507–2517.
Saraswathi, S., Sundaram, S., Sundararajan, N., Zimmer-
mann, M., and Nilsen-Hamilton, M. (2011). Icga-pso-
elm approach for accurate multiclass cancer classifica-
tion resulting in reduced gene sets in which genes en-
coding secreted proteins are highly represented. Com-
putational Biology and Bioinformatics, IEEE/ACM
Transactions on, 8(2):452–463.
Seco, N., Veale, T., and Hayes, J. (2004). An intrinsic
information content metric for semantic similarity in
wordnet. In de M
´
antaras, R. L. and Saitta, L., editors,
ECAI, pages 1089–1090. IOS Press.
Speed, T. P., editor (2003). Statistical Analysis of Gene Ex-
pression Microarray Data. Chapman and Hall.
Statnikov, A., Aliferis, C. F., Tsamardinos, I., Hardin, D.,
and Levy, S. (2005). A comprehensive evaluation
of multicategory classification methods for microar-
ray gene expression cancer diagnosis. Bioinformatics,
21(5):631–643.
Thomas, J. G., Olson, J. M., Tapscott, S. J., and Zhao,
L. P. (2001). An Efficient and Robust Statistical Mod-
eling Approach to Discover Differentially Expressed
Genes Using Genomic Expression Profiles. Genome
Research, 11(7):1227–1236.
Wang, L., Chu, F., and Xie, W. (2007). Accurate
cancer classification using expressions ofvery few
genes. IEEE/ACM Trans. Comput. Biology Bioinform,
4(1):40–53.
Wang, Y., Tetko, I. V., Hall, M. A., Frank, E., Facius, A.,
Mayer, K. F., and Mewes, H. W. (2005). Gene selec-
tion from microarray data for cancer classification–a
machine learning approach. Computational Biology
and Chemistry, 29(1):37 – 46.
Xu, T., Du, L., and Zhou, Y. (2008). Evaluation of GO-
based functional similarity measures using S. cere-
visiae protein interaction and expression profile data.
BMC Bioinformatics.
Yeoh, E.-J., Ross, M. E., Shurtleff, S. A., Williams, W., Pa-
tel, D., Mahfouz, R., Behm, F. G., Raimondi, S. C.,
Relling, M. V., Patel, A., Cheng, C., Campana, D.,
Wilkins, D., Zhou, X., Li, J., Liu, H., Pui, C.-H.,
Evans, W. E., Naeve, C., Wong, L., and Downing, J. R.
(2002). Classification, subtype discovery, and pre-
diction of outcome in pediatric acute lymphoblastic
leukemia by gene expression profiling. Cancer Cell,
1(2):133 – 143.
KDIR 2011 - International Conference on Knowledge Discovery and Information Retrieval
302