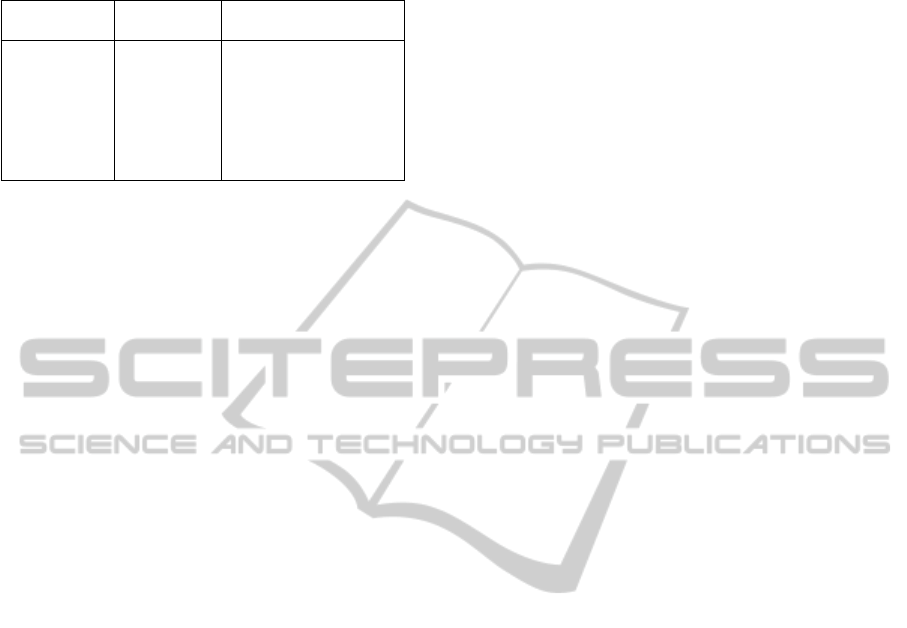
Table 6: Hypernyms obtained and WordNet hypernym for
the group of terms related with term plant.
Term Hypernym WordNet
obtained hypernym
plant organism organism, being
park plant tract, piece of land
garden park vegetation
region park location
safari garden expedition, travel
environment garden geographical area
vegetation plant collection,aggregation
5 CONCLUSIONS
This paper describes an approach to discover hyper-
nyms. The use of the related information in web
queries seems a good approximation for narrowing
the search results. This kind of queries is the most
concrete and indicates that 1) there is a relation be-
tween terms and 2) the terms and their hypernym are
in the same context. The method can be applied to
any domain knowledge. WordNet seems to be lim-
ited because it does not nouns with more than one
term and it only includes some proper nouns. The
obtained results can be improved resolving ambigu-
ous terms. Adding new lexical patterns to queries and
extending the search to Frequently Questions Blogs
and Wikipedia are good options to explore. The cre-
ated taxonomies are consistent with the input corpus.
This makes possible that taxonomies can be used on
applications where the structure of corpus content is
crucial. Finally, in the futher work will be consid-
ered additional experimentation and comparison with
other state of art approaches.
REFERENCES
Blohm, S. and Cimiano, P. (2007). Learning Patterns
from the Web-Evaluating the Evaluation Functions-
Extended Abstract. OTT’06, 1:101.
Burgun, A. and Bodenreider, O. (2001). Aspects of the tax-
onomic relation in the biomedical domain. In Pro-
ceedings of the international conference on Formal
Ontology in Information Systems-Volume 2001, page
233. ACM.
Caraballo, S. (1999). Automatic construction of a
hypernym-labeled noun hierarchy from text. In Pro-
ceedings of the 37th annual meeting of the Association
for Computational Linguistics, pages 120–126. Asso-
ciation for Computational Linguistics.
Cederberg, S. and Widdows, D. (2003). Using LSA and
noun coordination information to improve the preci-
sion and recall of automatic hyponymy extraction. In
Proceedings of the seventh conference on Natural lan-
guage learning at HLT-NAACL 2003-Volume 4, page
118. Association for Computational Linguistics.
Cicurel, L., Bloehdorn, S., and Cimiano, P. (2007). Cluster-
ing of polysemic words. Advances in Data Analysis,
pages 595–602.
Cimiano, P. and Staab, S. (2004). Learning by googling.
ACM SIGKDD explorations newsletter, 6(2):24–33.
Gruber, T. (1993). A translation approach to portable ontol-
ogy specifications. Knowledge acquisition, 5(2):199–
220.
Hearst, M. (1992). Automatic acquisition of hyponyms
from large text corpora. In Proceedings of the 14th
conference on Computational linguistics-Volume 2,
pages 539–545. Association for Computational Lin-
guistics.
Maedche, A. and Staab, S. (2001). Ontology learning
for the semantic web. Intelligent Systems, IEEE,
16(2):72–79.
Ortega-Mendoza, R., Villase
˜
nor-Pineda, L., and y G
´
omez,
M. M. (2007). Using lexical patterns for extracting
hyponyms from the web. MICAI 2007: Advances in
Artificial Intelligence, pages 904–911.
Pantel, P. (2003). Clustering by committee. PhD thesis,
University of Alberta.
Pantel, P. and Lin, D. (2002). Discovering word senses from
text. In Proceedings of the eighth ACM SIGKDD in-
ternational conference on Knowledge discovery and
data mining, pages 613–619. ACM.
Pantel, P., Ravichandran, D., and Hovy, E. (2004). Towards
terascale knowledge acquisition. In Proceedings of
the 20th international conference on Computational
Linguistics, page 771. Association for Computational
Linguistics.
Ritter, A., Soderland, S., and Etzioni, O. (2009). What is
this, anyway: Automatic hypernym discovery. In Pro-
ceedings of AAAI-09 Spring Symposium on Learning
by Reading and Learning to Read, pages 88–93.
S
´
anchez, D. (2009). Domain ontology learning from
the web. The Knowledge Engineering Review,
24(04):413–413.
Sang, E. (2007). Extracting hypernym pairs from the web.
In Proceedings of the 45th Annual Meeting of the
ACL on Interactive Poster and Demonstration Ses-
sions, pages 165–168. Association for Computational
Linguistics.
Schutz, A. and Buitelaar, P. (2005). Relext: A tool for re-
lation extraction from text in ontology extension. In
Gil, Y., Motta, E., Benjamins, V., and Musen, M., ed-
itors, The Semantic Web ISWC 2005, volume 3729 of
Lecture Notes in Computer Science, pages 593–606.
Springer Berlin / Heidelberg.
Snow, R., Jurafsky, D., and Ng, A. (2005). Learning
syntactic patterns for automatic hypernym discovery.
Advances in Neural Information Processing Systems,
17:1297–1304.
Turney, P. D. and Pantel, P. (2010). From frequency to
meaning: vector space models of semantics. J. Artif.
Int. Res., 37:141–188.
KEOD 2011 - International Conference on Knowledge Engineering and Ontology Development
278