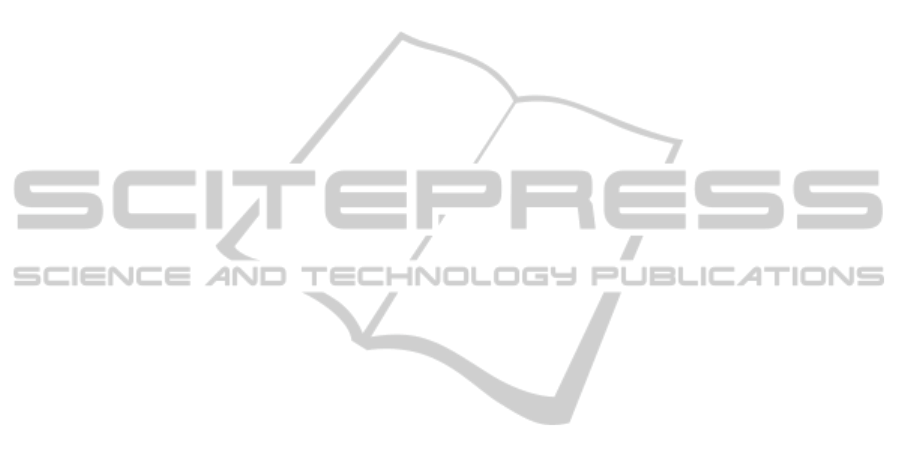
classification of normal subjects and epileptic pa-
tients were done with the accuracy of 100%, only if
when the number of features for classification M and
the size of dictionary N are properly set.
Although the proposed method has shown good
performance on the EEG signal classification, there
still remain some problems to be solved. The speed
is relatively slow and the selection of dictionary size
and number of features is a key point to classifica-
tion accuracy. So how to speed up the sparse repre-
sentation calculation and how to automatically de-
termine the size of dictionary and the number of
features suitable to EEG classification are our future
work.
ACKNOWLEDGEMENTS
The authors wish to thank the anonymous reviewers
for their useful suggestions and comments on the
paper. And the authors also would like to express
their sincere thanks to Ms. Xiuling Zhou for her
many useful suggestions.
The research work described in this paper was
fully supported by the grants from the National
Natural Science Foundation of China, (Project No.
90820010, 60911130513) Prof. Guo is the author to
whom the correspondence should be addressed, his
E-Mail address is pguo@ieee.org
REFERENCES
Nigam, V., & Graupe, D., 2004. A neural-network-based
detection of epilepsy. In Neurological Research,
26(1), 55–60.
Srinivasan, V., Eswaran, C., & Sriraam, N., 2005. Artifi-
cial neural network basedepileptic detection using
time-domain and frequency-domain features. In Jour-
nal of Medical Systems.
Güler, N., Übeyli, E. & Güler, I., 2005. Recurrent neural
networks employing Lyapunov exponents for EEG
signals classification. In Expert Systems with Applica-
tions, Subasi, A. ,2005-A. Automatic recognition of
alertness level from EEG byusing neural network and
wavelet coefficients. In Expert Systems with Applica-
tions, Subasi, A.,2005-B. Epileptic seizure detection
using dynamic wavelet network. In Expert Systems
with Applications.
Subasi, A., 2006.Automatic detection of epileptic seizure
using dynamic fuzzy neural networks. In Expert Sys-
tems with Applications.
Subasi, A., 2007. Application of adaptive neuro-fuzzy
inference system for epileptic seizure detection using
wavelet feature extraction, In Computers in Biology
and Medicine.
Subasi, A., 2007. EEG signal classification using wavelet
feature extraction and a mixture of expert model, In
Expert Systems with Applications 32 (4) (2007)1084–
1093.
Übeyli, E., 2009. Combined neural network model em-
ploying wavelet coefficientsfor EEG signals classifica-
tion. In Digital Signal Processing.
Ocak, H., 2009. Automatic detection of epileptic seizures
in EEG using discretewavelet transform and approxi-
mate entropy. In Expert Systems with Applications.
Wright, J., Yang, A. Y., Ganesh, A., Sastry, S. S. and Ma,
Y., 2009.Robust Face Recognition via Sparse Repre-
sentation, In IEEE Transactions on Pattern Analysis
and Machine Intelligence(PAMI).
Huang, K., and Aviyente, S., 2007. “Sparse representation
for signal classification,” In NIPS.
Richard, G., Baraniuk. Compressive Sensing., 2007. In
IEEE Signal Processing Magazine [118] July.
Zhang, Ch., Yin, Zh., Chen, X. D., and Xiao, M. X., 2005.
Signal overcomplete representation and sparse decom-
position based on redundant dictionaries, In Chinese
Science Bulletin.
Aharon, M. Elad, M. Bruckstein, A.M., 2006. The K-
SVD: an algorithm for designing Overcomplete Dic-
tionaries for Sparse Representation, In signal Process-
ing, IEEE.
Mairal, J. Bach, F. Ponce, J. and Sapiro, G., 2009. Online
dictionary learning for sparse coding, In ICML.
Mallat, S. and Zhang, Z., 1993. Matching pursuits with
time-frequency dictionaries, In IEEE Transactions on
Signal Processing.
Mallat, S. Davis, G. and Zhang, Z., 1994. Adaptive time-
frequency decompositions, In SPIE Journal of Optical
ngineering.
Donoho, D. and Huo, X., 2001. Uncertainty principles and
ideal atomic decomposition, In
IEEE Trans.on Infor-
mation Theory .
Guo, L., et al., 2011. Automatic feature extraction using
genetic programming: An application to epileptic EEG
classification. In Expert Systems with Applications
(2011).
Andrzejak, R., Lehnertz, K., Mormann, F., Rieke, C.,
David, P., & Elger, C., 2001. Indications of nonlinear
deterministic and finite-dimensional structures in
timeseries of brain electrical activity: Dependence on
recording region and brainstate.IN Physical Review E.
Christopher M. Bishop, 2006 Pattern Recognition and
Machine Learning, Springer Science+Business Media,
Turkey.
EPILEPTIC ELECTROENCEPHALOGRAM SIGNAL CLASSIFICATION BASED ON SPARSE REPRESENTATION
23