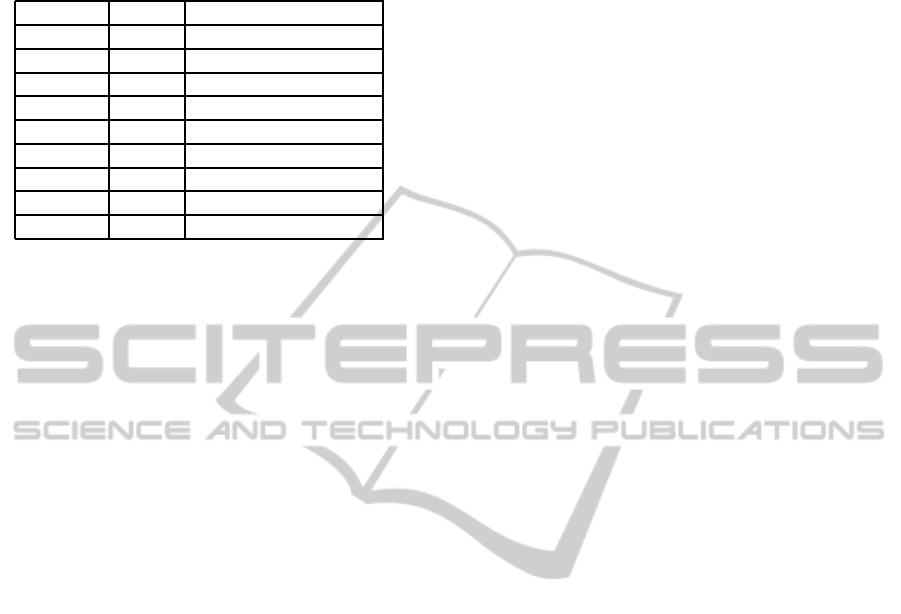
Table 5: Performance of HCHIRSIM algorithm in terms of
precision, recall, lexical overlap, ontological improvement
and ontological loss for λ = 1.
λ = 1 MeSH Documents collection
Selected 881 528
Rejected 463 256
Total 1344 784
LR 59,93%
LP 67,35%
F
β
63,42%
LO 57,89%
OI 2,04%
OL 42,11%
5.2 Discussion
Taking λ = 1 corresponds to the Chir-Statistic tech-
nique which is an improved version of the chi-statistic
test (Resnik, 1999). While taking λ = 0 corre-
sponds to the mutual information dependency mea-
sure (Church and Hanks, 1990). Instead of only
exploiting mutual information measure or only us-
ing statistic technique or sequential method given by
(Djaanfar et al., 2010) which is time consuming, we
use a hybrid measure. The performance evaluation
is conducted by setting different weights. The ex-
perimental results are presented in tables 1-5. In
general, the hybrid method yields better performance
than statistics-based or linguistics-based. More-
over, the performance of the hybrid method for the
weight λ = 0.5 (LR = 93, 87%, LP = 89, 02%, F
β
=
91, 38%, LO = 86, 83%, OI = 7, 04%, OL = 13, 17%)
is much higher than that of the other weights.
6 CONCLUSIONS
This paper presented a hybrid method combining sta-
tistical and semantic approaches for automating the
ontology construction process by retrieving and ex-
tracting data from Web resources. The obtained algo-
rithm called HCHIRSIM can be adapted to any do-
main ontology learning from the Web. The exper-
iments show that our hybrid approach outperforms
both purely statistical and purely semantic relation-
ships among concepts approaches. The successful
evaluation of our method with different values of
the weighting parameter shows that the proposed ap-
proach can effectively construct a cancer domain on-
tology from unstructured text documents.
REFERENCES
Brun, A., Smaili, K., and Haton, J.-P. (2002). Wsim : une
mthode de dtection de thme fonde sur la similarit entre
mots. In 9me conf. fran. TALN’2002, Nancy, France.
Budanitsky, A. (1999). Lexical semantic relatedness and its
application in natural language processing. In Tech-
nical Report CSRG-390. Computer Systems Research
Group, University of Toronto.
Church, K. and Hanks, P. (1990). Word association norms,
mutual information, and lexicograph. Computational
Linguistics, 16(1).
Craven, M., Dipasquo, D., Freitag, D., McCallum, A.,
Mitchell, T., Nigam, K., and Slattery (2000). Learn-
ing to construct knowledge bases from the world wide
web. Artificial Intelligence, 118(1):69–113.
Croft, B. and Ponte, J. (1998). A language modeling ap-
proach to information retrieval. In 21st International
Conference on Research and Development in Informa-
tion Retrieval.
Dagan, I., Lee, L., and Pereira, F. C. N. (1999). Similarity-
based models of word co-occurrence probabilities.
Machine Learning, 34:43–69.
Djaanfar, A. S., Frikh, B., and Ouhbi, B. (2010). A do-
main ontology learning from the web. In M. Saadi et
al. (eds), Studies in Comp. Intel., Vol(315), 201-208,.
Springer-Verlag.
Etzioni, O., Cafarella, M., Downey, D., Popescu, A.,
Shaked, T., Soderland, S., , Weld, D., and Yates, A.
(2005). Unsupervised named-entity extraction from
the web: an experimental study. Artificial Intelligence,
165(1):91–134.
Fotzo, H. and Gallinari, P. (2004). Learning generaliza-
tion/specialization relations between concepts appli-
cation for automatically building thematic document
hierarchies. In The 7th International Conference
on Computer-Assisted Information Retrieval (RIAO).
RIAO Vaucluse, France.
Frikh, B., Djaanfar, A. S., and Ouhbi, B. (2009). An in-
telligent surfer model combining web contents and
links based on simultaneous multiple-term query. In
The seventh ACS/IEEE International Conference on
Computer Systems and Applications (AICCSA-2009),
IEEE Computer Society.
Fuhr, N. (1992). Probabilistic models in information re-
trieval. The Computer Journal, 35(3):243–255.
Li, Y., Luo, C., and Chung, S. M. (2008). Text clustering
with feature selection by using statistical data knowl-
edge and data engineering. IEEE Transactions on
Know and Data Eng., 20(5):641–651.
Maedche, A., Pekar, V., and Staab, S. (2002). Ontology
learning part one-on discovering taxonomic relations
from the web. Springer-Verlag.
Maedche, A. and Staab, S. (2002). Measuring similarity be-
tween ontologies. In European Conference on Knowl-
edge Acquisition and Management (EKAW), Madrid,
Spain.
Mesh (2010). Medical Subject Headings. National Library
of Medicine’s controlled vocabulary thesaurus.
A HYBRID METHOD FOR DOMAIN ONTOLOGY CONSTRUCTION FROM THE WEB
291