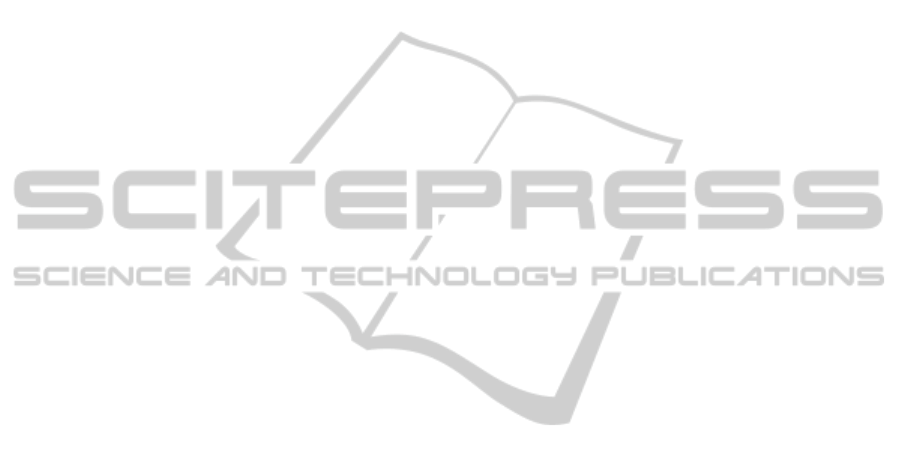
KLIM_L estimator achieves higher classification
accuracy than LDA, RDA, LOOC and KLIM
estimators on COIL-20 data set. In the future work,
the kernel method combined with these
regularization discriminant methods will be studied
for small sample problem with high dimension and
the selection of kernel parameters will be
investigated under some criterion.
ACKNOWLEDGEMENTS
The research work described in this paper was fully
supported by the grants from the National Natural
Science Foundation of China (Project No.
90820010, 60911130513). Prof. Guo is the author to
whom the correspondence should be addressed, his
e-mail address is pguo@ieee.org
REFERENCES
Bishop, C. M., 2007. Pattern recognition and machine
learning, Springer-Verlag New York, Inc. Secaucus,
NJ, USA.
Everitt, B. S., Hand, D., 1981. Finite Mixture
Distributions, Chapman and Hall, London.
Friedman, J. H., 1989. Regularized discriminant analysis,
Journal of the American Statistical Association, vol.
84, no. 405, 165–175.
Hoffbeck, J. P. and Landgrebe, D. A., 1996. Covariance
matrix estimation and classification with limited
training data, IEEE Transactions on Pattern Analysis
and Machine Intelligence, vol. 18, no. 7, 763–767.
Schafer, J. and Strimmer, K., 2005. A shrinkage approach
to large-scale covariance matrix estimation and
implications for functional genomics, Statistical
Applications in Genetics and Molecular Biology, vol.
4, no. 1.
Srivastava, S., Gupta, M. R., Frigyik, B. A., 2007.
Bayesian quadratic discriminant analysis, J. Mach.
Learning Res. 8, 1277
–1305.
Bickel, P. J. and Levina, E., 2008. Regularized estimation
of large covariance matrices, Annals of Statistics, vol.
36, no. 1, 199–227.
Friedman, J., Hastie, T., and Tibshirani, R., 2008. Sparse
inverse covariance estimation with the graphical lasso,
Biostatistics, vol. 9, no. 3, 432–441.
Cao, G., Bachega, L. R., Bouman, C. A., 2011. The Sparse
Matrix Transform for Covariance Estimation and
Analysis of High Dimensional Signals. IEEE
Transactions on Image Processing, Volume 20, Issue
3, 625 – 640.
Rivals, I., Personnaz, L., 1999. On cross validation for
model selection, Neural Comput. 11,863
–870.
Guo, P., Jia, Y., and Lyu, M. R., 2008. A study of
regularized Gaussian classifier in high-dimension
small sample set case based on MDL principle with
application to spectrum recognition, Pattern
Recognition, Vol. 41, 2842~2854.
Redner, R. A., Walker, H. F., 1984. Mixture densities,
maximum likelihood and the EM algorithm, SIAM
Rev. 26, 195
–239.
Aeberhard, S., Coomans, de Vel, D., O., 1994.
Comparative analysis of statistical pattern recognition
methods in high dimensional settings, Pattern
Recognition 27 (8), 1065
–1077.
Rissanen, J., 1978. Modeling by shortest data description,
Automatica 14, 465
–471.
Barron, A., Rissanen, J., Yu, B., 1998. The minimum
description length principle in coding and modeling,
IEEE Trans. Inform. Theory 44 (6), 2743
–2760.
Kullback, S., 1959. Information Theory and Statistics,
Wiley, New York.
Nene, S. A., Nayar, S. K. and Murase, H., 1996. Columbia
Object Image library(COIL-20). Technical report
CUCS-005-96.
MULTI-REGULARIZATION PARAMETERS ESTIMATION FOR GAUSSIAN MIXTURE CLASSIFIER BASED ON
MDL PRINCIPLE
117