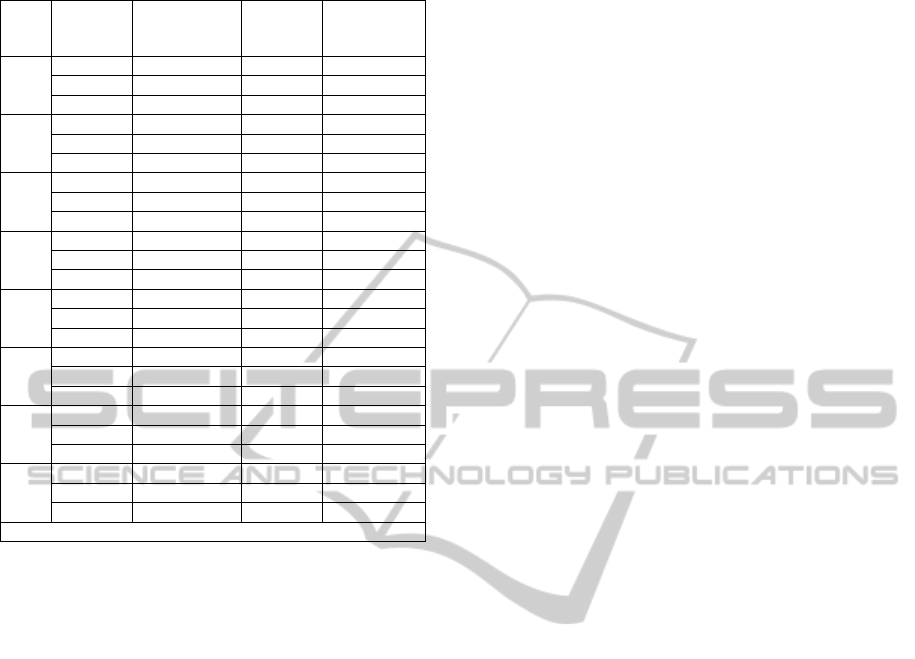
Table 3: Results obtained by HGA for instance 2.
Initial
pop.
Crossover
strategy
Average total
cost (
avg
TC )
Average
CPU time
(seconds)
% Deviation
A
SC 43,035.66* 455 17,374.58%
PC 128,479.62* 387 52,068.99%
RC 85,750.71* 795 34,718.97%
B
SC 393.67 501 59.85%
PC 393.45 407 59.76%
RC 382.21 826 55.19%
C
SC 43,109.16* 480 17,404.42%
PC 391.80 407 59.09%
RC 85,820.74* 821 34,747.41%
D
SC 271.29 570 10.16%
PC 262.67 378 6.65%
RC 246.28 807 0.00%
E
SC 290.57 491 17.99%
PC 288.28 382 17.06%
RC 279.24 813 13.38%
F
SC 279.20 485 13.37%
PC 280.46 377 13.88%
RC 259.47 803 5.36%
G
SC 85,755.44* 468 34,720.89%
PC 85,762.53* 391 34,723.77%
RC 43,040.22* 802 17,376.43%
H
SC 392.97 497 59.56%
PC 393.88 405 59.93%
RC 381.33 823 54.84%
*Solutions where flights were not all covered, resulting in penalty
4 CONCLUSIONS
This study treated the Crew Assignment Problem
(CAP), important part of the airlines operational
planning. A Hybrid Genetic Algorithm (HGA)
associated with a constructive heuristic and a local
search was developed. The HGA yielded feasible
and efficient solutions for the considered instances
with reduced CPU times (order of 8 to 14 minutes).
Elements of the GRASP metaheuristic combined
with a constructive heuristic led HGA to be more
robust and effective. The introduction of the local
search heuristic (LSH) proved to be a way to get
more effective solutions for the CAP. Besides, the
RC (random crossover) strategy proposed in this
study was more effective than other crossover
strategies (SC and PC) found in the literature.
ACKNOWLEDGEMENTS
The authors acknowledge CAPES (Coordenação de
Aperfeiçoamento de Pessoal de Nível Superior),
CNPq (Conselho Nacional de Desenvolvimento
Científico), and LPT/EPUSP (Laboratório de
Planejamento e Operação de Transportes da EPUSP)
for supporting this research.
REFERENCES
ANAC, 2011. Lei do aeronauta (7.183/74). Available at:
<http://www2.anac.gov.br/biblioteca/leis/lei7183%20.
pdf> [Accessed 4 May 2011].
Barnhart, C. et al., 2003. Airline crew scheduling. In: R.
W. Hall, ed.
Handbook of Transportation Science.
Boston: Kluwer Scientific Publishers, p. 1-48.
Clarke, G., Wright, J. W., 1964. Scheduling of vehicles
from a central depot to a number of delivery points.
Operations Research, 12, p. 568-581.
Feo, T. A., Resende, M. G. C., 1995. Greedy randomized
adaptive search procedures.
Journal of Global
Optimization
, 6, p. 109-133.
Gopalakrishnan, B., Johnson, E., 2005. Airline crew
scheduling: State-of-the-art.
Annals of Operations
Research
, 140 (2), p. 305-337.
ILOG, 2007.
CPLEX 11.0 User’s manual. France: ILOG.
Kohn, N., Karisch, S. E., 2004. Airline crew rostering:
Problem types, modeling and optimization.
Annals of
Operations Research
, 127, p. 223-257.
Lucic, P., Teodorovic, D., 2007. Metaheuristics approach
to the aircrew rostering problem.
Annals of Operations
Research
, 155, p. 311-338.
Matsumoto, M., Nishimura, T., 1998. Mersenne Twister:
A 623-dimensionally equidistributed uniform
pseudorandom number generator.
ACM Transactions
on Modeling and Computer Simulation
, 8 (1), p. 3-30.
Nemhauser, G. L., Wolsey, L. A., 1999. Integer and
combinatorial optimization. Wiley – Interscience.
SNA, 2011.
Convenção coletiva de trabalho - 2010/2012.
Available at: <http://www.aeronautas.org.br/images/
stories/convcol/cc_2010_2012.pdf> [Accessed 4 May
2011].
Souai, N., Teghem, J., 2009. Genetic algorithm based
approach for the integrated airline crew-pairing and
rostering problem.
European Journal of Operational
Research
, 199, p. 674-683.
Zeghal, F. M., Minoux, M., 2006. Modeling and solving a
crew assignment problem in air transportation.
European Journal of Operational Research, 175, p.
187-209.
A HYBRID GENETIC ALGORITHM FOR THE AIRLINE CREW ASSIGNMENT PROBLEM
195