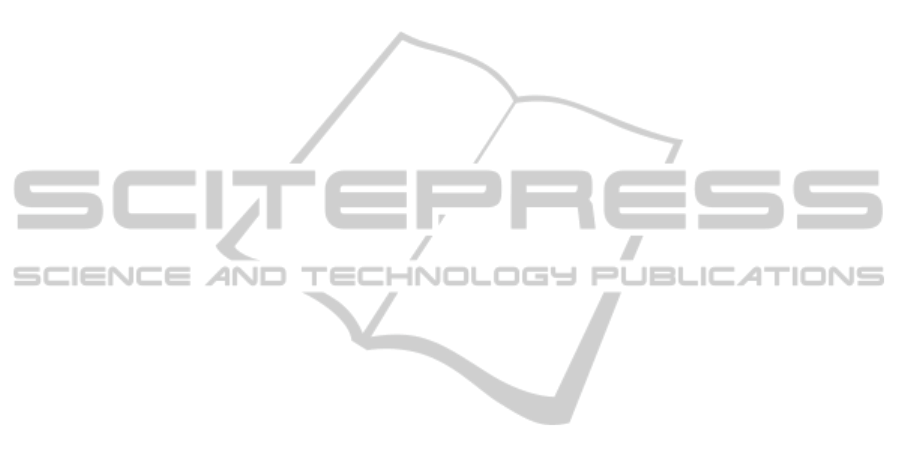
6 CONCLUSIONS
In the computer aided diagnosis, the image seg-
mentation is often used as a first step in an au-
tomated case analysis. As such, it benefits from
the fuzzy notion provided by the fuzzy connected-
ness. After 15 years since being announced the fuzzy
connectedness-based algorithms appeared in multiple
segmentation applications and programming environ-
ments (e.g. note the specified FC filters in the ITK –
Insight Toolkit – environment). The properly defined
connectedness between points within objects is help-
ful in achieving precise and reliable delineation of the
structures. Sometimes the FC analysis is sufficient as
a standalone segmentation method; other times it pro-
vides just the most accurate part of a larger process.
The spatial relations and fuzziness used in the seg-
mentation step permit better resemblance to the pro-
cessing performed by the human brain and perceptual
system. In our work the fuzzy connectedness proved
to be a powerfull tool; we can conclude, that its fuzzi-
ness improves flexibility of the segmentation process.
REFERENCES
Abrahams, J. M., Saha, P. K., Hurst, R. W., LeRoux,
P. D., and Udupa, J. K. (2002). Three-Dimensional
Bone-Free Rendering of the Cerebral Circulation Us-
ing Computed Tomographic Angiography and Fuzzy
Connectedness. Neurosurgery, 51:264–269.
Admasu, F., Al-Zubi, S., Toennies, K., Bodammer, N., and
Hinrichs, H. (2003). Segmentation of Multiple Scle-
rosis Lesions from MR Brain Images Using the Prin-
ciples of Fuzzy-Connectedness and Artificial Neuron
Networks. In International Conference on Image Pro-
cessing, ICIP 2003, volume 2, pages II 1081–1084.
Badura, P. and Pietka, E. (2007). Semi-Automatic Seed
Points Selection in Fuzzy Connectedness Approach
to Image Segmentation. In Computer Recognition
Systems 2 (Advances in Soft Computing), volume 45,
pages 679–686. Springer-Verlag.
Badura, P. and Pietka, E. (2008). Pre- and Postprocessing
Stages in Fuzzy Connectedness-Based Lung Nodule
CAD. In Information Technologies in Biomedicine
(Advances in Soft Computing), volume 47, pages 192–
199. Springer-Verlag.
Carvalho, B. M., Joe Gau, C., Herman, G. T., and
Yung Kong, T. (1999). Algorithms for Fuzzy Seg-
mentation. Pattern Analysis & Applications, 2:73–81.
Ciesielski, K. C., Udupa, J. K., Saha, P. K., and Zhuge, Y.
(2007). Iterative Relative Fuzzy Connectedness for
Multiple Objects with Multiple Seeds. Comput. Vis.
Image Underst., 107(3):160–182.
Czajkowska, J., Badura, P., and Pietka, E. (2010). 4D Seg-
mentation of Ewing’s Sarcoma in MR Images. In
Information Technologies in Biomedicine: Volume 2
(Advances in Intelligent and Soft Computing), vol-
ume 69, pages 91–100. Springer-Verlag.
Dehmeshki, J., Amin, H., Valdivieso, M., and Ye, X.
(2008). Segmentation of Pulmonary Nodules in Tho-
racic CT Scans: A Region Growing Approach. IEEE
Transactions on Medical Imaging, 27(4):467–480.
Gonzalez, R. and Woods, R. (2002). Digital Image Process-
ing. Prentice Hall, Upper Saddle River, NJ.
Herman, G. T. and Carvalho, B. M. (2001). Multiseeded
Segmentation Using Fuzzy Connectedness. IEEE
Transactions on Pattern Analysis and Machine Intel-
ligence, 23(5):460–474.
Kawa, J. and Pietka, E. (2008). Automated Fuzzy-
Connectedness-Based Segmentation in Extraction of
Multiple Sclerosis Lesions. In Information Technolo-
gies in Biomedicine (Advances in Soft Computing),
volume 47, pages 149–156. Springer-Verlag.
Moonis, G., Liu, J., Udupa, J. K., and Hackney, D. (2002).
Estimation of Tumor Volume Using Fuzzy Connect-
edness Segmentation of MRI. Am. J. Neuroradiol.,
23(3):356–363.
Perona, P. and Malik, J. (1990). Scale-Space and Edge De-
tection Using Anisotropic Diffusion. IEEE Transac-
tions on Pattern Analysis and Machine Intelligence,
12(7):629–639.
Saha, P. K. and Udupa, J. K. (2001). Fuzzy Connected Ob-
ject Delineation: Axiomatic Path Strength Definition
and the Case of Multiple Seeds. Computer Vision and
Image Understanding, 83(3):275–295.
Saha, P. K., Udupa, J. K., and Odhner, D. (2000). Scale-
Based Fuzzy Connected Image Segmentation: The-
ory, Algorithms, and Validation. Computer Vision and
Image Understanding, 77(9):145–174.
Tschirren, J., Hoffman, E. A., McLennan, G., and Sonka,
M. (2005). Intrathoracic Airway Trees: Segmentation
and Airway Morphology Analysis from Low-Dose
CT Scans. IEEE Transactions on Medical Imaging,
24(12):1529–1539.
Udupa, J. K., Odhner, D., and Eisenberg, H. C. (2001).
New Automatic Mode of Visualizing the Colon via
CT. In SPIE: Medical Imaging, volume 4319, pages
237–243, San Diego, CA.
Udupa, J. K., Saha, P., and Lotufo, R. (2002). Relative
Fuzzy Connectedness and Object Definition: Theory,
Algorithms, and Applications in Image Segmentation.
IEEE Transactions on Pattern Analysis and Machine
Intelligence, 24(11):1485–1500.
Udupa, J. K. and Samarasekera, S. (1996). Fuzzy Connect-
edness and Object Definition: Theory, Algorithms,
and Applications in Image Segmentation. Graphical
Models and Image Processing, 58(3):246–261.
Udupa, J. K., Wei, L., Samarasekera, S., Miki, Y., van
Buchem, M. A., and Grossman, R. I. (1997). Mul-
tiple Sclerosis Lesion Quantification Using Fuzzy-
Connectedness Principles. IEEE Transactions on
Medical Imaging, 16(5):598–609.
FCTA 2011 - International Conference on Fuzzy Computation Theory and Applications
492