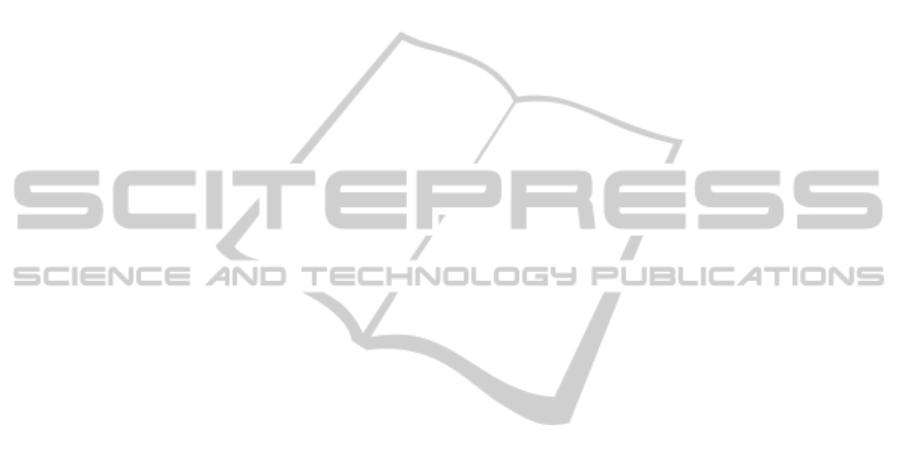
rated (Figure 2), and where application of s
Lev´y
solu-
tions in the population improved the results, that is,
obtained error decreased (Figure 3).
For each of the benchmark instances there were
obtained 32 values of oe which are presented in a
graphicalform as a surface generated for different val-
ues of the number of s
Lev´y
solutions (that is, ind
SαS
)
and α. The benchmark instances in the Figures are
ordered in the same way as in Table 2.
7 CONCLUSIONS
In this paper we introduce hybrid structure of popula-
tion in differential evolution algorithm jDE and study
its properties when applied to dynamic optimization
tasks. This is a case of a kind of technology trans-
fer where promising mechanisms form one heuris-
tic approach appear to be useful in another. The re-
sults show that mutation operator using random vari-
ates based on α-stable distribution, that is, the oper-
ator where both fine local modification and macro-
jumps can appear, improves the values of oe. Lack
of improvement appeared in two cases, that is, for
the DCBG with F4 (Rastrigin components) which is a
very difficult landscape looking like a hedgehog (Fig-
ure 1, the graph in the second row on the left) and for
the MPB sc.2 with ten cones. In both cases macro-
mutation most probably introduces unnecessary noise
rather than a chance for exploration of a new promis-
ing area. Difficulty of the former benchmark instance
is confirmed by extremely high values of error ob-
tained for all three types of change.
In the remaining cases the influence of s
Lev´y
solu-
tion presence is positive, however, it must be stressed
that the number of these solutions should be small. In
most cases the best results were obtained for just one
piece. The exception is the DCBG with F5 (Grievank
components) which was the easiest landscape for the
algorithm, easier even than the landscape build of the
spheres. In the case of the DCBG with F5 the higher
number of s
Lev´y
, the smaller value of oe.
Finally, it is worth to stress that the different com-
plexity of the tested instances shows also that when
we take them as a suite of tests and evaluate over-
all gain of the algorithm we need to apply different
weight for the successes obtained for each of the in-
stances. Simply, an improvement of efficiency ob-
tained for DCBG with Grievank components havedif-
ferent significance than the same improvement for,
e.g., DCBG with Ackley components.
REFERENCES
Branke, J. (1999). Memory enhanced evolutionary algo-
rithm for changing optimization problems. In Proc. of
the Congr. on Evolutionary Computation, volume 3,
pages 1875–1882. IEEE Press, Piscataway, NJ.
Brest, J., Greiner, S., Boskovic, B., Mernik, M., and Zumer,
V. (2006). Self-adapting control parameters in dif-
ferential evolution: A comparative study on numeri-
cal benchmark problems. IEEE Trans. Evol. Comput.,
10(6):646–657.
Brest, J., Zamuda, A., Boskovic, B., Maucec, M. S., and
Zumer, V. (2009). Dynamic optimization using self-
adaptive differential evolution. In IEEE Congr. on
Evolutionary Computation, pages 415–422. IEEE.
Feokistov, V. (2006). Differential Evolution, In Search of
Solutions, volume 5 of Optimization and Its Applica-
tions. Springer.
Li, C. and Yang, S. (2008). A generalized approach to con-
struct benchmark problems for dynamic optimization.
In Simulated Evolution and Learning, SEAL, volume
5361 of LNCS, pages 391–400. Springer.
Liang, J. J., Suganthan, P. N., and Deb, K. (2005). Novel
composition test functions for numerical global opti-
mization. In IEEE Swarm Intelligence Symposium,
pages 68–75, Pasadena, CA, USA.
Price, K. V. (1994). Genetic annealing. Dr. Dobb’s Journal,
pages 127–132.
Price, K. V., Storn, R. M., and Lampinen, J. A. (2005). Dif-
ferential Evolution, A Practical Approach to Global
Optimization. Natural Computing Series. Springer.
Salomon, R. (1996). Reevaluating genetic algorithm perfor-
mance under coordinate rotation of benchmark func-
tions. BioSystems, 39(3):263–278.
Suganthan, P. N., Hansen, N., Liang, J. J., Deb, K., Chen,
Y.-P., Auger, A., and Tiwari, S. (2005). Problem defi-
nitions and evaluation criteria for the cec 2005 special
session on real-parameter optimization. Technical re-
port, Nanyang Technological University, Singapore.
Trojanowski, K. (2009). Properties of quantum particles in
multi-swarms for dynamic optimization. Fundamenta
Informaticae, 95(2-3):349–380.
HYBRID RULES OF PERTURBATION IN DIFFERENTIAL EVOLUTION FOR DYNAMIC OPTIMIZATION
41