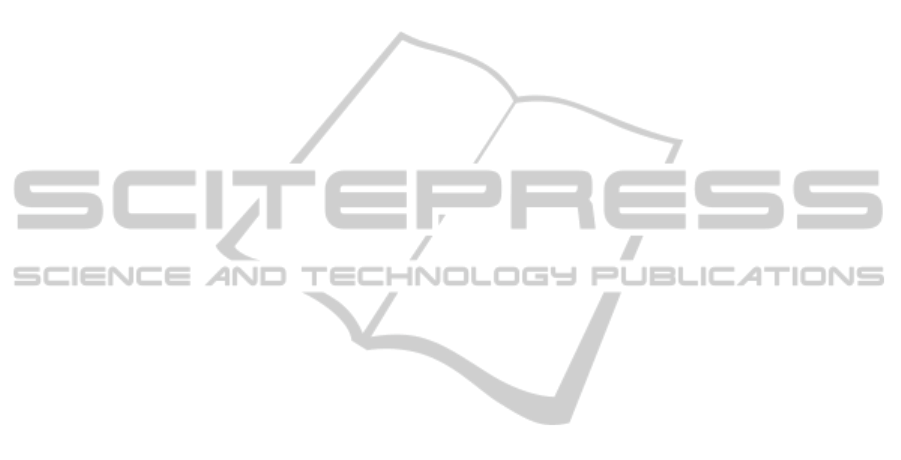
benchmark constrained nonlinear programming prob-
lems have been considered. These problems have also
been solved with the stochastic ranking and the feasi-
bility and dominance rules techniques and compari-
son has been made based on their performance pro-
files. It is shown that m-CDE with the global com-
petitive ranking, based on a fixed value of P
f
, is rel-
atively better than the other two techniques. A com-
parison has also been made with other results from the
literature: the adaptive penalty-based differential evo-
lution, the stochastic ranking based on an evolution
strategy, and the global competitive ranking based on
an evolution strategy. It is shown that m-CDE is rather
competitive when compared with the other solution
methods. Future developments will focus on the ex-
tension of the m-CDE to problems with mixed integer
variables.
ACKNOWLEDGEMENTS
This work is supported by FCT (Fundac¸˜ao para a
Ciˆencia e a Tecnologia) and Ciˆencia 2007, Portugal.
We thank two anonymous referees for their valuable
comments to improve this paper.
REFERENCES
Ali, M. M., Khompatraporn, C. and Zabinsky, Z. B. (2005).
A numerical evaluation of several stochastic algo-
rithms on selected continuous global optimization test
problems. Journal of Global Optimization, 31, 635–
672.
Barbosa, H. J. C. and Lemonge, A. C. C. (2003). A new
adaptive penalty scheme for genetic algorithms. Infor-
mation Sciences, 156, 215–251.
Brest, J., Greiner, S., Boˇskovi´c, B., Mernik, M. and
ˇ
Zumer,
V. (2006). Self-adapting control parameters in differ-
ential evolution: a comparative study on numerical
benchmark problems. IEEE Transactions on Evolu-
tionary Computation, 10, 646–657.
Coello Coello, C. A. (2000). Constraint-handling using an
evolutionary multiobjective optimization technique.
Civil Engineering and Environmental Systems, 17,
319–346.
Coello Coello, C. A. and Cort´es, N. C. (2004). Hybridiz-
ing a genetic algorithm with an artificial immune sys-
tem for global optimization. Engineering Optimiza-
tion, 36, 607–634.
Deb, K. (2000). An efficient constraint handling method
for genetic algorithms. Computer Methods in Applied
Mechanics and Engineering, 186, 311–338.
Dolan, E. D. and Mor´e, J. J. (2002). Benchmarking opti-
mization software with performance profiles. Mathe-
matical Programming, 91, 201–213.
Dong, Y., Tang, J., Xu, B. and Wang, D. (2005). An appli-
cation of swarm optimization to nonlinear program-
ming. Computers & Mathematics with Applications,
49, 1655–1668.
Fletcher, R. and Leyffer, S. (2002). Nonlinear programming
without a penalty function. Mathematical Program-
ming, 91, 239–269.
Fourer, R., Gay, D. M. and Kernighan, B. W. (1993). AMPL:
A modelling language for matematical programming.
Boyd & Fraser Publishing Co.: Massachusets.
Hedar, A. R. and Fukushima, M. (2006). Derivative-free fil-
ter simulated annealing method for constrained con-
tinuous global optimization. Journal of Global Opti-
mization, 35, 521–549.
Holland, J. H. (1975). Adaptation in Natural and Artificial
Systems. University of Michigan Press: Ann Arbor.
Kaelo, P. and Ali, M. M. (2006). A numerical study of some
modified differencial evolution algorithms. European
Journal of Operational Research, 169, 1176–1184.
Ray, T. and Tai, K. (2001). An evolutionary algorithm with
a multilevel pairing strategy for single and multiob-
jective optimization. Foundations of Computing and
Decision Sciences, 26, 75–98.
Ray, T. and Liew, K. M. (2003). Society and civilization:
An optimization algorithm based on the simulation of
social behavior. IEEE Transactions on Evolutionary
Computation, 7, 386–396.
Rocha, A. M. A. C. and Fernandes, E.M.G.P. (2008). Feasi-
bility and dominance rules in the electromagnetism-
like algorithm for constrained global optimization.
In Gervasi et al. (Eds.), Computational Science and
Its Applications: Lecture Notes in Computer Science
(vol. 5073, pp. 768–783). Springer: Heidelberg.
Rocha, A. M. A. C. and Fernandes, E.M.G.P. (2009). Self
adaptive penalties in the electromagnetism-like algo-
rithm for constrained global optimization problems. In
Proceedings of the 8th World Congress on Structural
and Multidisciplinary Optimization (pp. 1–10).
Runarsson, T. P. and Yao, X. (2000). Stochastic ranking for
constrained evolutionary optimization. IEEE Transac-
tions on Evolutionary Computation, 4, 284–294.
Runarsson, T.P. and Yao, X. (2003). Constrained evolution-
ary optimization – the penalty function approach. In
Sarker et al. (Eds.), Evolutionary Optimization: Inter-
national Series in Operations Research and Manage-
ment Science (vol. 48, pp. 87–113), Springer: New
York.
Silva, E. K., Barbosa, H. J. C. and Lemonge, A. C. C.
(2011). An adaptive constraint handling technique for
differential evolution with dynamic use of variants in
engineering optimization. Optimization and Engineer-
ing, 12, 31–54.
Storn, R. and Price, K. (1997). Differential evolution – a
simple and efficient heuristic for global optimization
over continuous spaces. Journal of Global Optimiza-
tion, 11, 341–359.
Vaz, A. I. F. and Vicente, L. N. (2007). A particle swarm
pattern search method for bound constrained global
optimization. Journal of Global Optimization, 39,
197–219.
Zahara, E. and Hu, C.-H. (2008). Solving constrained op-
timization problems with hybrid particle swarm opti-
mization. Engineering Optimization, 40, 1031–1049.
GLOBAL COMPETITIVE RANKING FOR CONSTRAINTS HANDLING WITH MODIFIED DIFFERENTIAL
EVOLUTION
51