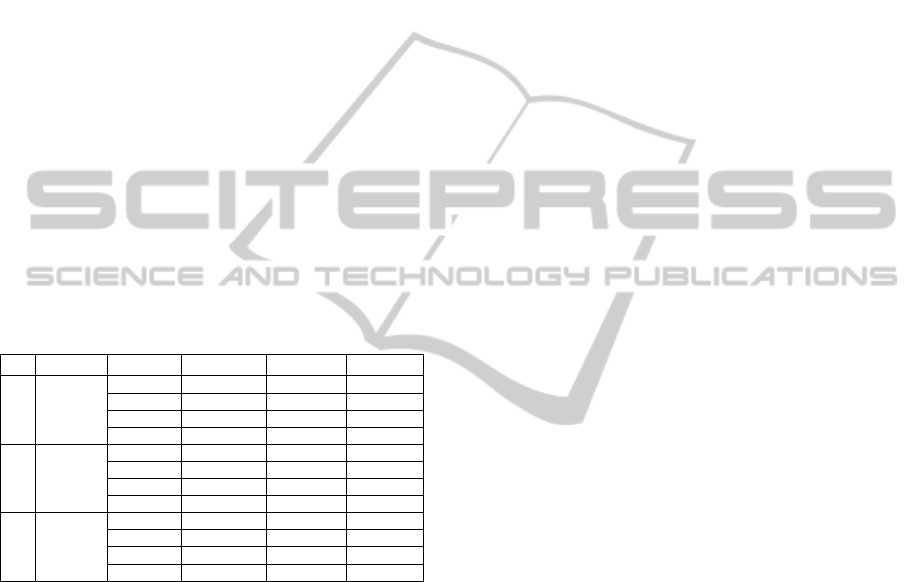
version rather than a single node version. Here, the
structure is composed of three nodes as shown in
figure 1. The main advantages are to reduce the total
number of rules of the system, as the rule set of each
node is smallest and also to enhance the recognition
rate. Table 1 shows the learning rates (LR), the
generalisation rates (GR), the number of features
(#Param.) and the number of generated rules
(#Rules) obtained for five methods.
Despite the proposed method provided better
results considering separately each node, the
recognition rates obtained by SFFS, SBFS and the
proposed method (PM) are comparable. Scores
between 97% and 98% are reached for the
generalization step considering the whole database.
The expert selection (EXP) gives rise to a rate
around 96%. The difference is insignificant.
However, the number of parameters extracted by
our method is the lowest assuming the better
interpretability of the model. The numbers of rules
are carried out using expert (EXP: 3775 rules) and
the Proposed Method (PM: 1375 rules). Other
methods lead to a rule number higher than 350 000.
Table 1: Recognition rates and Generated rules.
EXP SFFS SBSF PM
Node 0
LR 96.4% 96.% 96,00% 96.4%
GR 90.59% 91.38% 91.39% 90.59%
#Param 4 2 2 4
#Rules 625
25 25
625
Node 1
LR 78.771% 94.97% 90.50% 78.77%
GR 72.236% 77.64% 74.69% 72.24%
#Param
5
9 8
5
#Rules 3125 1953125 390625 3125
Node 2
LR 100.% 100.% 100.% 100.%
GR 96.818%
97.27% 97.27%
96.82%
#Param 2 3 3 2
#Rules
25
125 125
25
4 CONCLUSIONS
An enhancement of a Fuzzy Rule Iterative Feature
Selection method has been presented. It allows the
decreasing of learning time processing while
focusing on relevant samples. A suitable set of
features is obtained. Then, FRIFS method is adapted
to handle with a hierarchical fuzzy inference system.
Industrial real-data tests show the efficiency of the
proposed method. The recognition rate is similar to
other methods but the number of features
significantly decreases and thus the number of rules
too. Actually, the extension of our model to provide
selection of parameters per class is under
consideration.
REFERENCES
Abdulhady, M., Abbas, H., Nassar, S., 2005. Performance
of neural classifiers for fabric faults classification. In
proc. IEEE International Joint Conference on Neural
Networks (IJCNN '05), Montreal, Canada, 1995-2000.
Bombardier, V., Schmitt, E., 2010. Fuzzy rule classifier:
Capability for generalization in wood color
recognition. In Eng. Appli. of Artificial Intelligence,
v23, 978-988.
Grabisch, M., Nicolas, J. M., 1994. Classification by fuzzy
integral - performance and tests. In Fuzzy Sets and
Systems, v65, 255-271.
Grabisch, M., 1995. The application of fuzzy integral in
multicriteria decision making. In Europ. journal of
operational research, v89, 445-456.
Kudo, M., Sklansky, J., 2000. Comparison of algorithms
that select features for pattern classifiers. In Pattern
Recognition, v 33, 25–41.
Murino, V., Bicego, M., Rossi, I. A., 2004. Statistical
classification of raw textile defects. In Proc. of the
17th Int. Conf. on Pattern Recognition (ICPR’04),
Cambridge, UK, 311- 314.
Murofushi, T., Soneda, S. 1993. Techniques for reading
fuzzy measures(iii): interaction index. In proc. 9th
Fuzzy System Symposium, Sapporo, Japan, 693-696.
Pudil, P., Novovicova, J., Kittler, J., 1994. Floating search
methods in feature selection. In Pattern Recognition
Letters, v15, 1119–1125.
Rendek, J., Wendling, L. 2006. Extraction of Consistent
Subsets of Descriptors using Choquet Integral. In
Proc. 18th Int. Conf. on Pattern Recognition, Hong
Kong, 208-211.
Shapley, L., 1953. A value for n-person games.
Contributions to the Theory of Games. In Annals of
Mathematics Studies. Khun, H., Tucker A., Princeton
University Press 307-317.
Schmitt, E., Bombardier, V., Wendling, L., 2008.
Improving Fuzzy Rule Classifier by Extracting
Suitable Features from Capacities with Respect to the
Choquet Integral. In IEEE trans. On System, man and
cybernetics v38-5 1195-1206.
Yang, X., Pang, G., Yung, N., 2002. Fabric defect
classification using wavelet frames and minimum
classification error training. In 37th IAS Industry
Application Conference, Pittsburgh, USA, 290–296.
Zhang, H., Sun, G. 2002. Feature selection using Tabu
Search method. In Pattern Recognition, v 35 701–711.
FCTA 2011 - International Conference on Fuzzy Computation Theory and Applications
496