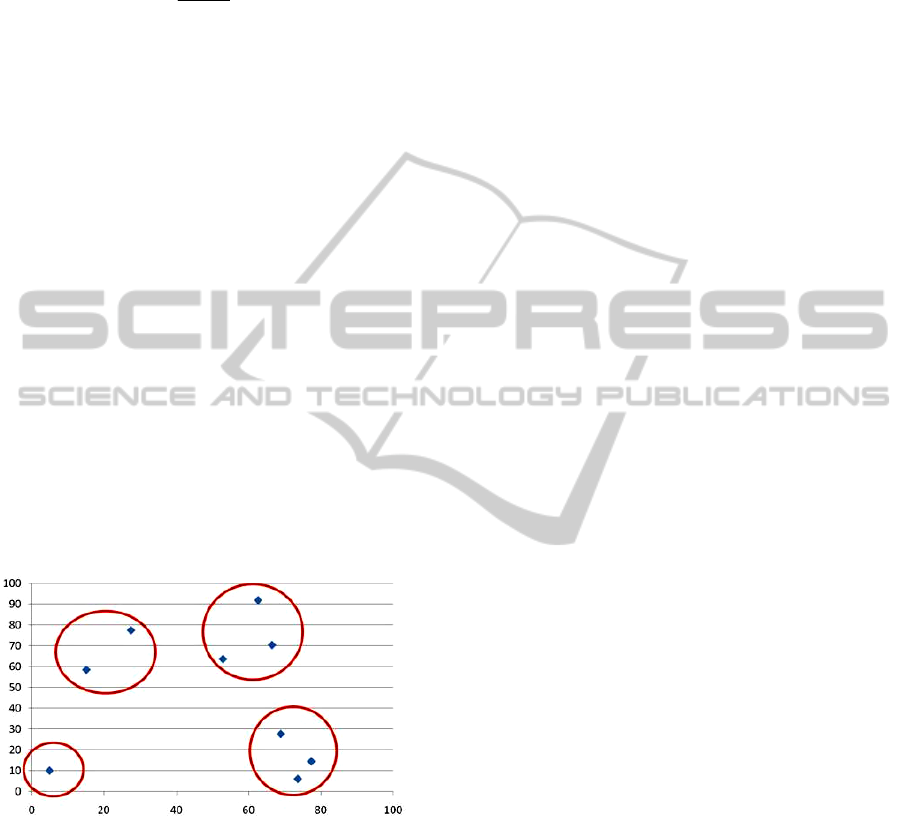
component and the deviations for Training design
models from it (2) and (3).
n
M
M
n
i
i
avg
∑
=
=
0
(2)
iavg
d
i
MMM −=
(3)
The final evaluation of models is the sum of
deviations of each model totals from the average (4).
d
i
d
i
d
i
d
i
d
i
UOTME +++=
(4)
Setting in advance the thresholds (t) the final
scores for two models E
i
and E
j
are considered the
same if their difference is bellow threshold.
tEE
d
j
d
i
<− ||
(5)
The final result of comparison of multiple user
training design models is clustered of similar models
depending on thresholds neighbourhood (Figure 5).
There are two cases:
One of the clusters dominates – in this case we
choose its aggregated training model for the
whole learning group.
None of the clusters is dominant – then we split
the group on subgroups corresponding to
clusters and perform aggregated training model
for each cluster individually.
Figure 5: Clustered space to four training design models.
4 CONCLUSIONS
In this paper we present work in progress. On the
current stage of the project we need to tune the
linguistic variables values and to test different
membership functions and inference engine
techniques for generating output values. The final
decision which of them fits best to our domain
representation would be made on the testing results
base. Further research steps will be followed by
testing and validating the model with available data
for already passed teachers’ trainings. On the results
base, the model will be refined. In order to approve
the proposed approach applicability into practice, the
prototype of the build fuzzy system will be tested
with teachers and instructional designers of teachers’
trainings.
REFERENCES
Cachia, R., Ferrari, A., Ala-Mutka K., Punie Y., 2010.
Creative Learning and Innovative Teaching: Final
Report on the Study on Creativity and Innovation in
Education in EU Member States, http://ftp.jrc.es/
EURdoc/JRC62370.pdf, Institute for Prospective
Technological Studies, last visited 27/05/2011.
Guskey, T. R., 2010. Evaluating Professional
Development, Corwin Press
Mishra, P., Koehler J. M, 2006. Technological
Pedagogical Content Knowledge: A Framework for
Teacher Knowledge, Teachers College Record,
Volume 108, Number 6, June 2006, pp. 1017–1054
Pratt, D., Ainley, J., Kent, P., Levinson, R., Yogui, C., and
Kapadia, R. (in press). Informal inferential reasoning
as a contextualised risk-based enterprise. To appear in
Mathematical Thinking and Learning
Stefanova E., Boytcheva S., 2010. Theoretical Framework
of Open Virtual Worlds for Professional Development,
In proceeding of S3T’2010 conference, Varna,
Bulgaria, 11-12 September 2010, pp.79-83
Stefanova E., Boytcheva S., 2011. Teachers’ Training
Design Model and Decision Support System, Springer
proceeding of S3T’2011 conference, Burgas, Bulgaria,
1-3 September 2011, pp.192-196
Stoyanov, S. & Kirschner, P. A., 2004. Expert concept
mapping method for defining the characteristics of
adaptive e-learning: ALFANET project case.
Educational Technology Research and Development,
52, 2, 41–56
Trochim, W., 1989. An introduction to concept mapping
for planning and evaluation, Evaluation and Program
Planning 12, 1–16
Wopereis, I., Kirschner, P. A., Paas, F., Stoyanov, S.,
Hendriks, M., 2005. Failure and success factors of
educational ICT projects: a group concept mapping
approach, British Journal of Educational Technology,
36, (4) 2005, 681–684
Zadeh L. A., 1965. Fuzzy Set, Information and control, v.
8, pp.338-353
Zadeh L. A., 1973. Outline of new approach to the
analysis of complex systems and decision processes,
IEEE Transactions on systems, man, and cybernetics,
vol. SMC-3, No.1, January 1973, pp.28-44
FCTA 2011 - International Conference on Fuzzy Computation Theory and Applications
508