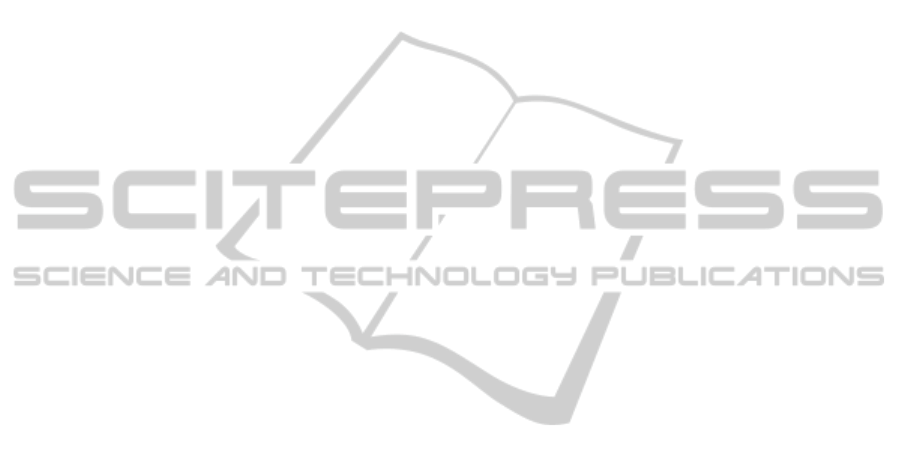
4 FUTURE WORK
The next stage is the implementation of a monitoring
system based on the described AIS method. The
direct outcomes are to achieve a proof of a “non-
self” and “self” set in the used acceleration data set
and a dynamic adaptation in immature and mature
detectors. A dynamic variation in the monitoring can
be achieved by mutating detectors at specific time
intervals (lifespan). The mutation process can be
based on different method like random generation or
more advanced clonal algorithms. Therefore further
research will look into the following areas:
• Possible use of data pre-processing
• Generation of detector
• Lifespan of detector
• Matching of detector and data instance
• Uncertainty after a mature detector is
triggered
The authors believe that research in these areas will
lead to an improved recognition in fall detection.
The research outcome will be compared against the
earlier results using FFT and neural networks.
ACKNOWLEDGEMENTS
This work is supported by the University of
Portsmouth under the Higher Education Innovation
Fund (HEIF 4) and performed by the Digital
Wellbeing Research Group at the same University.
REFERENCES
Yixin Cai, Mo-Yuen Chow, Wenbin Lu, & Lexin Li;.
(2010). Evaluation of distribution fault diagnosis
algorithms using ROC curves. Power and Energy
Society General Meeting, 2010 IEEE , 1 - 6.
Virone. (2008). Monitoring activity patterns and trends of
older adults. Engineering in Medicine and Biology
Society, 2008. EMBS 2008. 30th Annual International
Conference of the IEEE , 2071 - 2074.
Bersch, S., Chislett, C., Azzi, D., & Khusainov, R. (2011,
Jan 1). Activity detection using frequency analysis and
off-the-shelf devices: fall detection from accelero-
meter data. eprints.port.ac.uk .
Bersini, H., & Carneiro, J. (2006). Artificial Immune
Systems: 5th International Conference, ICARIS 2006,
Oeiras, Portugal, September 4-6, 2006, Proceedings
(Lecture Notes in Computer Science).
Boylan, G. (2011, Jan 1). EEG monitoring in the neonatal
intensive care unit: A critical juncture. Clinical
neurophysiology: official journal of the … .
Broek, G., Cavallo, F., Odetti, L., & Wehrmann, C. (2009,
Sep 22). Ambient Assisted Living Roadmap. 1-120.
Cagnoni, S., Matrella, G., Mordonini, M., Sassi, F., &
Ascari, L. (2009). Sensor Fusion-Oriented Fall
Detection for Assistive Technologies Applications.
Intelligent Systems Design and Applications, 2009.
ISDA '09. Ninth International Conference on , 673 -
678.
Commission, E., Economic, D.-G., & Affairs, F. (2009).
Ageing Report 2009.
Elbert, D., Storf, H., Eisenbarth, M., Ünalan, Ö., &
Schmitt, M. (2011). An approach for detecting
deviations in daily routine for long-term behavior
analysis. Workshop: Orange Alerts - Behaviour
Modeling and Health of older people in their homes
(AAL 2011). Dublin.
Franco, C., Demongeot, J., Villemazet, C., & Vuillerme,
N. (2010). Behavioral Telemonitoring of the Elderly at
Home: Detection of Nycthemeral Rhythms Drifts from
Location Data. Advanced Information Networking and
Applications Workshops (WAINA), 2010 IEEE 24th
International Conference on , 759 - 766.
Golovko, V., Komar, M., & Sachenko, A. (2010).
Principles of neural network artificial immune system
design to detect attacks on computers. Modern
Problems of Radio Engineering, Telecommunications
and Computer Science (TCSET), 2010 International
Conference on , 237 - 237.
Hofmeyr, S. (2000, Jan 1). Architecture for an artificial
immune system. Evolutionary Computation .
Jui-Yu Wu;. (2010). Computational Intelligence-Based
Intelligent Business Intelligence System: Concept and
Framework. Computer and Network Technology
(ICCNT), 2010 Second International Conference on ,
334 - 338.
Monekosso, D., & Remagnino, P. (2010). Behavior
Analysis for Assisted Living. Automation Science and
Engineering … .
Polat, K. (2008, Jan 1). Artificial immune recognition
system with fuzzy resource allocation mechanism
classifier, principal component analysis and FFT
method based new hybrid automated …. Expert
Systems with Applications .
Polat, K. (2008, Jan 1). Computer aided medical diagnosis
system based on principal component analysis and
artificial immune recognition system classifier
algorithm. Expert Systems with Applications .
Sim, K., Yap, G.-E., Phua, C., Biswas, J., Phyo Wai, A.,
Tolstikov, A., et al. (2010). Improving the accuracy of
erroneous-plan recognition system for Activities of
Daily Living. e-Health Networking Applications and
Services (Healthcom), 2010 12th IEEE International
Conference on , 28 - 35.
Storf, H., Becker, M., & Riedl, M. (2009). Rule-based
activity recognition framework: Challenges, technique
and learning. Pervasive Computing Technologies for
Healthcare, 2009. PervasiveHealth 2009. 3rd Interna-
tional Conference on, 1 - 7.
Timmis, J. (2007, Jan 1). Artificial immune systems—
today and tomorrow. Natural Computing.
FALL DETECTION USING BIOLOGIALLY INSPIRED MONITORING - Artificial Immune System in the Area of
Assisted Living
323