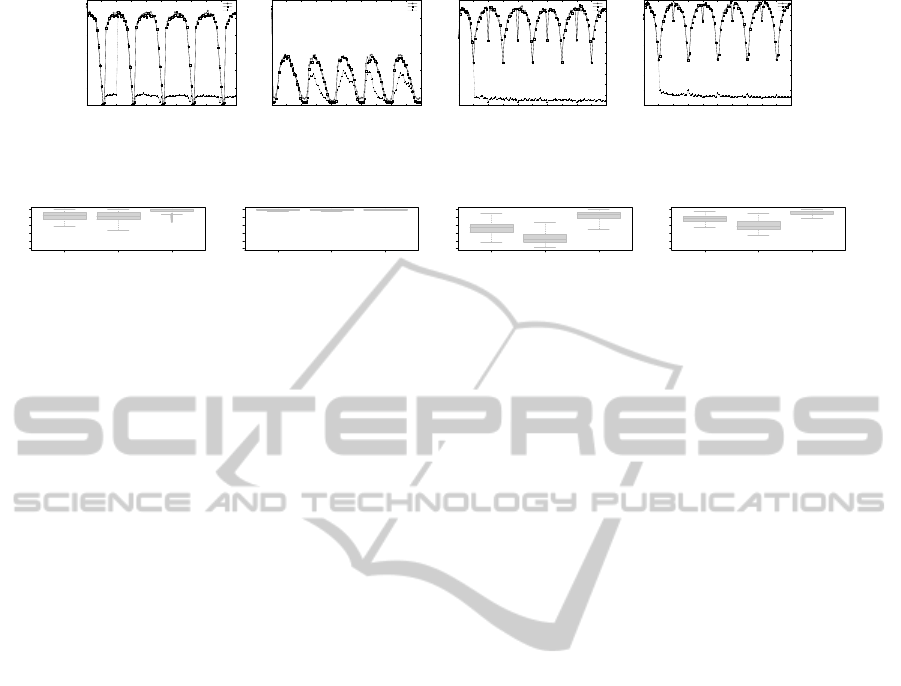
0
10
20
30
40
50
60
0 10 20 30 40 50 60 70 80 90 100
Number of generations
Changes
DNSGA-II-A
DNSGA-II-B
DNSGA-II-MEMORY
(a) FDA1
0
5
10
15
20
25
30
0 10 20 30 40 50 60 70 80 90 100
Number of generations
Changes
DNSGA-II-A
DNSGA-II-B
DNSGA-II-MEMORY
(b) FDA2
0
20
40
60
80
100
120
140
160
180
200
0 10 20 30 40 50 60 70 80 90 100
Number of generations
Changes
DNSGA-II-A
DNSGA-II-B
DNSGA-II-MEMORY
(c) DZDT2
0
20
40
60
80
100
120
140
0 10 20 30 40 50 60 70 80 90 100
Number of generations
Changes
DNSGA-II-A
DNSGA-II-B
DNSGA-II-MEMORY
(d) DZDT3
Figure 1: Number of generations required for approximate at the P F at a IGD of 0.01. Problem FDA1.
●
●
●
●
●
●
●
●
●
●
●
●
●
●
●
●
●
●
●
●
●
●
●
●
●
●
●
●
●
●
●
●
●
●
●
●
●
●
●
●
●
●
●
●
●
●
●
●
●
●
●
●
●
●
●
●
●
●
●
●
●
●
●
●
●
●
●
●
●
●
●
●
●
●
●●
●
●
●
●
●
●
●
●
●
●
●
●
●
●
●
●
●
●
●
●
●
●
●
●
●
●
●
●
●
●
●
●
●
●
●
●
●
●
●
●
●
●
●
●
●
●
●
●
●
●
●
●
●
●
●
●
●
●
●
●
●
●
●
●
●
●
●
●
●
●
●
●
●
●
●
●
●
●
●
●
●
●
●
●
●
●
●
●
●
●
●
●
●
●
●
●
●
●
●
●
●
●
●
●
●
●
●
●
●
●
●
●
●
●
●
●
●
●
●
●
●
●
●
●
●
●
●
●
●
●
●
●
●
●
●
●
●
●
●
●
●
●
●
●
●
●
●
●
●
●
●
●
●
●
●
●
●
●
●
●
●
●
●
●
●
●
●
●
●
●
●
●
●
●
●
●
●
●
●
●
●
●
●
●
●
●
●
●
●
●
●
●
●
●
●
●
●
●
●
●
●
●
●
●
●
●
DNSGA−II−A DNSGA−II−B DNSGA−II−MEMORY
0.0 0.2 0.4 0.6 0.8 1.0
Hipervolume ratio
Algoritmh
Hipervolume ratio
(a) FDA1
●
●
●
●
●
●
●
●
●
●
●
●
●
●
●
●
●
●
●
●
●
●
●
●
●
●
●
●
●
●
●
●
●
●
●
●
●
●
●
●
●
●
●
●
●
●
●
●
●
●
●
●
●
●
●
●
●
●
●
●
●
●
●
●
●
●
●
●
DNSGA−II−A DNSGA−II−B DNSGA−II−MEMORY
0.0 0.2 0.4 0.6 0.8 1.0
Hipervolume ratio
Algoritmh
Hipervolume ratio
(b) FDA2
●
●
●
●
●
●
●
●
●
●
●
●
●
●
●
●
●
●
●
●
●
●
●
●
●
●
●
●
●
●
●
●
●
●
●
●
●
●
●
●
●
●
●
●
●
●
●
●
●
●
●
●
●
●
●
●
●
●
●
●
●
●
●
DNSGA−II−A DNSGA−II−B DNSGA−II−MEMORY
0.0 0.2 0.4 0.6 0.8 1.0
Hipervolume ratio
Algoritmh
Hipervolume ratio
(c) DZDT2
●
●
●
●
●
●
●
●
●
●
●
●
●
●
●
●
●
●
●
●
●
●
●
●
●
●
●
●
●
●
●
●
●
●
●
●
●
●
●
●
●
●
●
●
●
●
●
●
●
●
●
●
●
●
●
●
●
●
●
●
●
●
●
●
●
●
●
●
●
●
●
●
●
●
●
●
●
●
●
●
●
●
●
●
●
●
●
●
●
●
●
●
●
●
●
●
●
●
●
●
●
●
●
●
●
●
●
●
●
●
●
●
●
●
●
●
●
●
●
●
●
●
●
●
●
●
●
●
●
●
DNSGA−II−A DNSGA−II−B DNSGA−II−MEMORY
0.0 0.2 0.4 0.6 0.8 1.0
Hipervolume ratio
Algoritmh
Hipervolume ratio
(d) DZDT3
Figure 2: Boxplots of 100 executions for FDA1, FDA2D, DZDT2 and DZDT3 during 100 changes.
FDA1 (the algorithm DNSGA-II-LTM could stabilize
its behavior over the time).
In FDA2 the three algorithm presented a good be-
havior, but again, the DNSGA-II-LTM reduced the
number of generations needed to follow the P F at
a IGD of 0.01 (see Figure 1(b)).
Since results from IGD indicate that the pro-
posed modification could outperform NSGA-II-A and
NSGA-II-B, we decided to perform a second experi-
ment. For this new experiment, we decided to mea-
sure the hypervolume ratio during 100 changes using
the three approaches (ours, the DNSGA-II-A and the
DNSGA-II-B). In order to present such results in a
friendly-comparison way, we decided to present them
as box-plot graphics which are shown in Figure 2.
From box-plots, we can see that the DNSGA-II-
LTM could reach to a hypervolume value close to
1.0 in most of the problems. The anomalous results
shown also in boxplots are due to the start of the
optimization process the algorithm had not sufficient
knowledge in memory and therefore, it was more dif-
ficult to follow the movement of the optimum. But
when the algorithm gained enough knowledge, the al-
gorithm could reach the P F most of the time.
5 CONCLUSIONS
According at the obtained result we can conclude that
the use of a long-term memory in dynamic multiob-
jective evolutionary algorithms reduces the number
of fitness function evaluations needed to optimize a
DMOP. It should be clear that in order to use a long-
term memory-based approach is necessary to use a
method to reduce the amount of information of each
environment to be stored in order to avoid saturation
of the memory. The proposed approach to generate
solutions from two points (in a connected region in
the parameter space) can be used to store only two so-
lutions at each change of the environment. It should
be noted that this method was only tested for prob-
lems with two objective functions. However, we are
planning to explore more dimensions in the objective
space in the future.
ACKNOWLEDGEMENTS
The first author gratefully acknowledges support from
CONACyT through project 105060. Also, this re-
search was partially funded by project number 51623
from “Fondo Mixto Conacyt-Gobierno del Estado
de Tamaulipas”. We would like to thank to Fondo
Mixto de Fomento a la Investigaci
´
on cient
´
ıfica y
Tecnol
´
ogica CONACyT - Gobierno del Estado de
Tamaulipas for their support to publish this paper.
REFERENCES
Bingul, Z. (2007). Adaptive Genetic Algorithms Applied to
Dynamic Multi-Objective Problems. Appl. Soft Com-
put., 7(3):791–799.
Branke, J. (1999). Memory enhanced evolutionary al-
gorithms for changing optimization problems. In
Congress on Evolutionary Computation CEC99,
pages 1875–1882. IEEE.
Branke, J., Kaussler, T., Schmidt, C., and Schmeck., H.
(2000). A multi-population approach to dynamic opti-
mization problems. In Adaptive Computing in Design
and Manufacturing, pages 299–307.
Deb, K., N., U. B. R., and Karthik, S. (2006). Dynamic
multi-objective optimization and decision-making us-
ing modified NSGA-II: A case study on hydro-thermal
power scheduling. In EMO, pages 803–817.
Farina, M., Deb, K., and Amato, P. (2003). Dynamic Mul-
tiobjective Optimization Problems: Test Cases, Ap-
proximation, and Applications. In Fonseca, C. M.,
Fleming, P. J., Zitzler, E., Deb, K., and Thiele, L., edi-
tors, Evolutionary Multi-Criterion Optimization. Sec-
ECTA 2011 - International Conference on Evolutionary Computation Theory and Applications
336