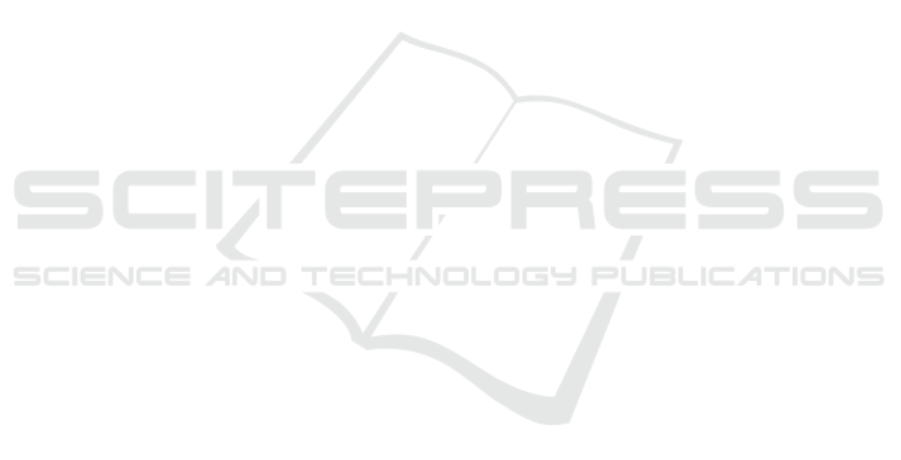
INDIVIDUALLY AND COLLECTIVELY TREATED NEURONS
AND ITS APPLICATION TO SOM
Ryotaro Kamimura
IT Education Center, Tokai University, 1117 Kitakaname, Hiratsuka, Kanagawa 258-1292, Japan
Keywords:
Individually treated neurons, Collectively treated nerons, Information-theoretic learning, Free enrgy, SOM.
Abstract:
In this paper, we propose a new type of information-theoretic method to interact individually treated neurons
with collectively treated neurons. The interaction is determined by the interaction parameter α. As the param-
eter α is increased, the effect of collectiveness is larger. On the other hand, when the parameter α is smaller,
the effect of individuality becomes dominant. We applied this method to the self-organizing maps in which
much attention has been paid to the collectiveness of neurons. This biased attention has, in our view, shown
difficulty in interpreting final SOM knowledge. We conducted an preliminary experiment in which the Iono-
sphere data from the machine learning database was analyzed. Experimental results confirmed that improved
performance could be obtained by controlling the interaction of individuality with collectiveness. In particular,
the trustworthiness and continuity are gradually increased by making the parameter α larger. In addition, the
class boundaries become sharper by using the interaction.
1 INTRODUCTION
Neurons in neural networks have been treated indi-
vidually or collectively in different learning methods.
No attempts have been made to examine the interac-
tion of individuality with collectiveness. In this paper,
we postulate that neurons should be treated individu-
ally and collectively and these two types of neurons
should interact with each other to have special effect
for neural learning. We, in particular, focus upon the
self-organizing maps (SOM) (Kohonen, 1988), (Ko-
honen, 1995). Because only the collectiveness of neu-
rons has been taken into account in the SOM, ignoring
the properties of individual treated neurons. Thus, it
is easy to demonstrate the effect of interaction using
the SOM.
The SOM is a well-known technique for the vec-
tor quantization and the vector projection from high
dimensional input spaces into low dimensional out-
put spaces. However, it is hard to interpret final SOM
knowledge from simple visual inspection. Thus,
many different types of visualization techniques have
been proposed, for example, the U-matrix and its vari-
ants (Ultsch, 2003b), (Ultsch, 2003a) visualization of
component planes (Vesanto, 1999), linear and non-
linear dimensionality reduction methods such as the
principal component analysis (PCA) (Bishop, 1995),
Sammon Map (Sammon, 1969) and many non-linear
methods (Joshua B. Tenenbaum and Langford, 2000),
(Roweis and Saul, 2000), (Demartines and Herault,
1997), and the responses to data samples (Vesanto,
1999). Recently, more advanced visualization tech-
niques were proposed such as gradient field and bor-
derline visualization techniques (Georg Polzlbauer
and Rauber, 2006), the connectivity matrix of proto-
type vectors (Tasdemir and Merenyi, 2009) and the
gradient-based SOM matrix (Costa, 2010) and so on.
Even using these visualization techniques, it re-
mains to be difficult to interpret final SOM knowl-
edge. The detection of class or cluster boundaries is,
in particular, a serious problem. If neurons on both
sides of class boundaries should behave differently, it
is easy to find the boundaries with some visualization
techniques. However, cooperation processes in SOM
diminishes the effect of the boundaries, because the
cooperation processes aim to increase continuity over
the output space. Intuitively, the continuity is contra-
dictory to the boundaries. In the proposed method,
the individuality as well as collectiveness of neurons
is introduced. The introduction of individuality is re-
lated to the more explicit detection of class boundaries
by reducing collectiveness.
24
Kamimura R..
INDIVIDUALLY AND COLLECTIVELY TREATED NEURONS AND ITS APPLICATION TO SOM.
DOI: 10.5220/0003677300240030
In Proceedings of the International Conference on Neural Computation Theory and Applications (NCTA-2011), pages 24-30
ISBN: 978-989-8425-84-3
Copyright
c
2011 SCITEPRESS (Science and Technology Publications, Lda.)