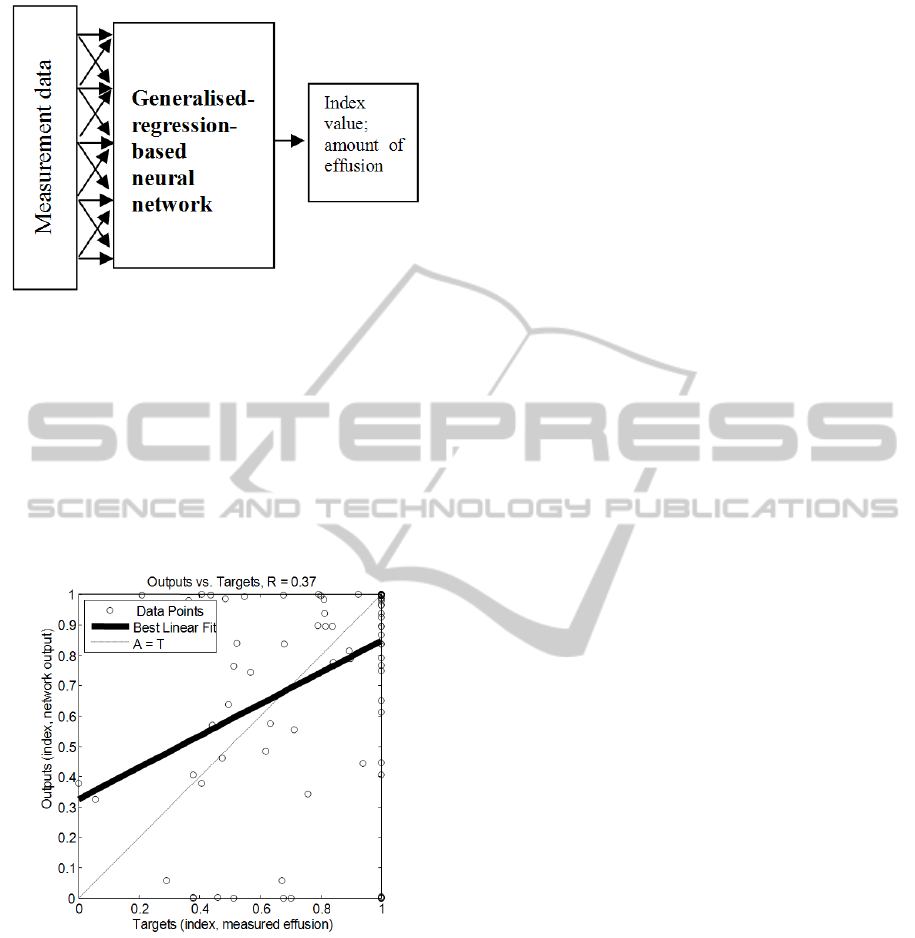
Figure 3: Neural network structure.
5 RESULTS
The neural network training and validation were
done with Matlab® software (Mathworks Inc,
Natick, USA). The correlation between the neural
network output and the amount of effusion as index
values in the ear is shown in Fig. 4.
Figure 4: Results of the neural network validation
(R=0.37, p < 0.001).
Fig. 4 shows that the neural network index and the
index of the measured amount of effusion correlate
statistically significantly (R = 0.37, p < 0.001).
6 DISCUSSION
AND CONCLUSIONS
The validation of the neural network analysis shows
that the correlation between the neural network
output and the measured amount of effusion in the
ear is clear, even though the correlation value is not
very high due to the preliminary characteristics of
the study. The result is in line with previous studies
(Linden et al., 2006; Chianese et al., 2007; Walsh et
al., 1998; Block et al., 1999; Teele and Teele, 1984)
and illustrates that the essential characteristic
features needed to estimate the amount of effusion in
the ear can be found from the AR measurement data.
In future studies the amount of data will be
increased in order to get higher correlations with
smaller deviations in single measurements. The idea
in this further development is to iteratively increase
the training data of the neural network of the
analysis system.
The data used in this investigation included a
number of unsuccessful measurements, 29 ears in
total. Technical problems which occurred during the
preliminary data collection were carefully
investigated and related improvements to the data
collection procedure were implemented in
subsequent measurements.
It should be kept in mind that in this study the
output of the network was an index with a linear
relationship to the amount of effusion only. Hence,
for example the pressure status of the ear was not
taken into account, which may account for part of
the deviations between the network output and the
determined ear status in the case of healthy ears with
no effusion at all. In order to improve the accuracy
of the measurements, further studies will more
extensively take into account the features of the ear,
related anthropometric characteristics and the middle
ear pressure. Also, the simplified linear index-based
approach used in this study may decrease the
correlation value between the neural network output
and the measured amount of effusion; application of
an appropriate nonlinear index would be better.
To conclude, the preliminary results of this
study were encouraging. The applied neural network
method has the capability to estimate the amount of
effusion in the ear at a statistical level, as shown
with the data set presented in this study. After
collection of the data set published in this study, the
number of data sets was increased essentially in the
next phase of the research project. In this phase an
improved measurement procedure was applied,
which substantially increased the repeatability and
noise tolerance of the measurements. The results of
those measurements will be published soon,
including evaluations about sensitivity and speficity
of the system in diagnostic application. Further, an
especially interesting application of the presented
measurement system is the possibility to use it at
PRELIMINARY RESULTS OF CLINICAL TESTS OF A NEW NEURAL-NETWORK-BASED OTITIS MEDIA
ANALYSIS SYSTEM
367