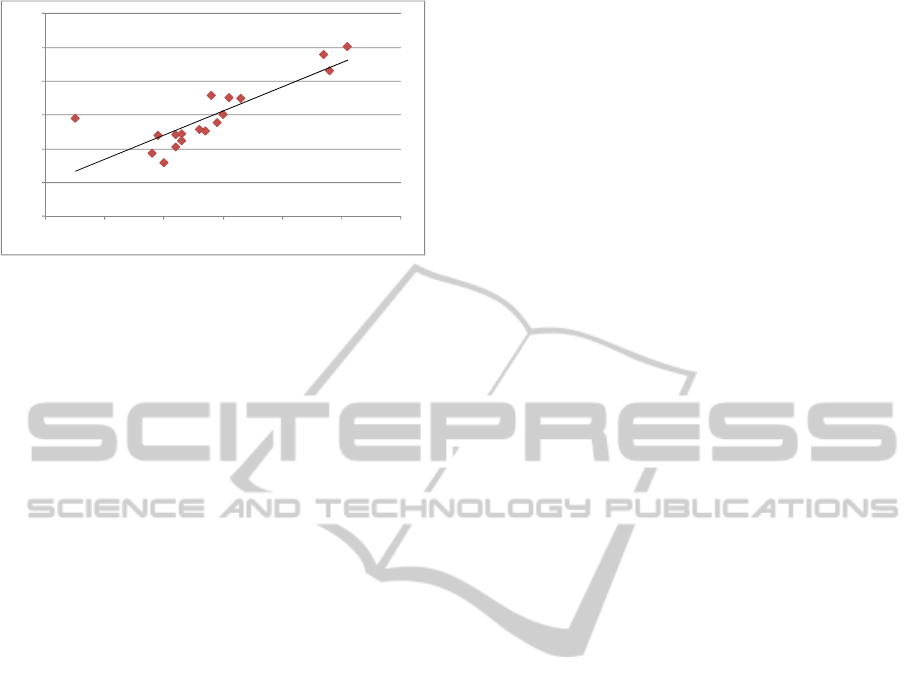
R²=0,70
4,0
5,0
6,0
7,0
8,0
9,0
10,0
4,0 5,0 6,0 7,0 8,0 9,0 10,0
Figure 4: Correlation between experimental data and
simulated data (neural network output) for R
a
.
5 CONCLUSIONS
The proposed hybrid approach based on Artificial
Neural Networks and Taguchi methodology was
used for AWJM mean kerf width and surface
roughness modelling purpose.
The Taguchi approach was used in order to
optimize the experimental effort whitout loosing the
prediction accuracy of the ANN model.
The acquired results indicate that the proposed
modelling approach could be effectively used to
predict the kerf geometry and the surface roughness
in AWJM, thus supporting the decision making
during process planning.
ACKNOWLEDGEMENTS
The authors wish to thank Sielman S.A., Volos,
Greece, for AWJM of the specimens. TRIP steels
were provided by Laboratory of Materials, Dept. of
Mechanical Engineering, University of Thessaly.
REFERENCES
Wang, J. & Wong, W. (1999). Study of abrasive waterjet
cutting of metallic coated sheet steels. International
Journal of Machine Tools & Manufacture, 39, pp. 855-
870.
Demuth, H. & Beale, M. (2001). Neural networks toolbox
for use with Matlab, User’s guide. The MathWorks,
Inc.
Gudimetla, P., Wang, J. & Wong, W. (2002). Kerf
formation analysis in the abrasive waterjet cutting of
industrial ceramics. Journal of Materials Processing
Technology, 128 (1-3), pp. 123-129.
Hascalik, A., Ulas, C. & Gurun, H. (2007). Effect of
traverse speed on abrasive waterjet machining of Ti-
6Al-4V alloy. Materials & Design, 28(6), pp. 1953-
1957.
Jegaraj, J. J. R. & Babu, N. R. (2007). A soft computing
approach for controlling the quality of cut with
abrasive waterjet cutting system experiencing orifice
and focusing tube wear. Journal of Materials
Processing Technology , 185 (1-3), pp. 217-227.
Kechagias, J. (2007). Investigation of LOM process
quality using design of experiments approach. Rapid
Prototyping Journal, 13(5), 316-323.
Kechagias, J., Pappas, M., Karagiannis, S., Petropoulos,
G., Iakovakis, V. & Maropoulos, S. (2010). An ANN
approach on the optimization of the cutting parameters
during CNC plasma-arc cutting. In: Proceedings of
ESDA2010: 10
th
Biennial ASME Conference on
Engineering Systems Design and Analysis, 12-14 July
2010, Istanbul, Turkey.
Momber, A. W. & Kovacevic, R. (1997). Principles of
Abrasive Water Jet Machining. Springer.
Olson, G. B. & Azrin, M. (1978). Transformation behavior
of TRIP steels. Metallurgical and Materials
Transactions A, 9(5), pp. 713-721.
Pappas, M., Kechagias, J., Iakovakis, V. & Maropoulos, S.
(2011). Surface roughness modelling and optimization
in CNC end milling using Taguchi design and Neural
Networks. In: Proceedings of the 3rd International
Conference on Agents and Artificial Intelligence, pp,
595-598.
Petropoulos, G., Tsolakis, N.; Vaxevanidis, N. &
Antoniadis, A. (2009) Topographic description of
abrasive waterjet machined surfaces. In: Proc. 2
nd
European Conference on Tribology -ECOTRIB 2009,
7-10 June 2009, Pisa, Italy, pp. 309-314.
Valicek, J. et al. (2007). Experimental analysis of
irregularities of metallic surfaces generated by
abrasive waterjet. International Journal of Machine
Tools & Manufacture, 47(11), pp. 1786-1790.
Vaxevanidis, N. M., Markopoulos A. & Petropoulos G.
(2010). Artificial Neural Network Modeling of
Surface Quality Characteristics in Abrasive Water Jet
Machining of Trip Steel Sheet. In: J.P. Davim (ed.)
Artificial Intelligence in Manufacturing Research.
Nova Science Publishers, Inc, pp 79-99.
NCTA 2011 - International Conference on Neural Computation Theory and Applications
380