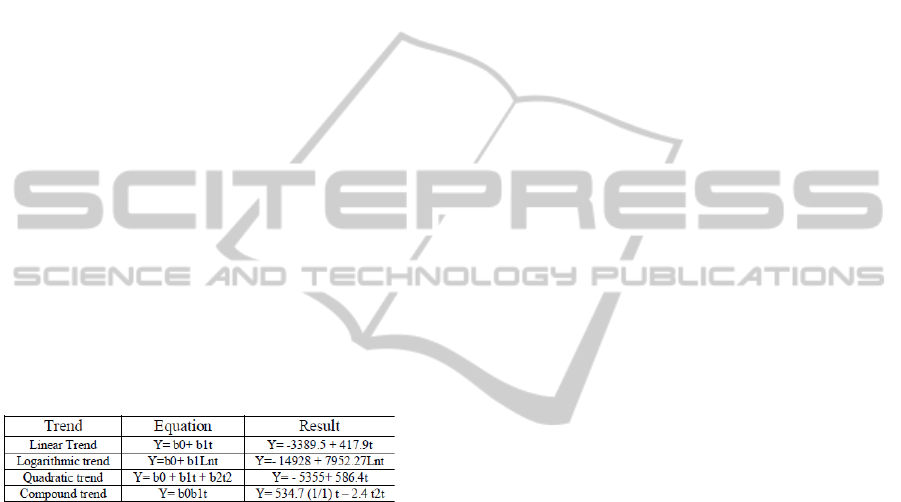
their error with α= 0.7 and β= 0.45 in this method.
Like other smoothing methods, parameters are
computed by trial and error.
Custom Smoothing with Damped Trend:
Forecasting by Damped trend is done using three
parameters: α,β and δ. The smoothing coefficients
are equal to α= 0.1,β= 0.1 and δ= 0.1. By using
these parameters the sum of square of the errors are
at a minimum.
The detailed results of errors are illustrated in
Table 5.
2. Forecasting by using Trend Analysis Method:
Different trends are analyzed in trend analysis as
follow: 1.Linear Trend, 2.Logarithmic Trend,
3.Inverse Trend, 4.Quadratic Trend, 5.Cubic trend,
6.Power Trend, 7.Compound Trend, 8.S- curve
Trend, 9.Logistic Trend, 10.Growth Trend,
11.Exponential Trend
For finding the best trend, all above trends are
formulated. The best trends are selected by
considering their R2 and MSE. ANOVA (analysis of
variance) results confirm that linear trend,
logarithmic trend, quadratic trend, and compound
trend are the most suitable trends. Equations of
selected trends are listed in Table 1:
Table 1: Most suitable Trend Equations.
The detailed error results are illustrated in Table 5.
3. Forecasting by using Box- jenkins Method: In
Box- Jenkins models (ARIMA), the following
analyses for statistical modeling were carried out:
1. Determination of normality and stationary of data.
2. Using Box-Cox conversion for normalizing data and
using differentiation for stationary data.
3. Computing auto-correlation coefficients, charts, and
studying partial auto-correlation coefficients.
According to this statistical modeling [ARIMA (1, 1,
1)], parameter p equals 1, parameter q equals 1, and
parameter d equals 1. The error results of this model
and their amounts are illustrated in Table 5.
4. Econometrics Causality Methods: In these
models, the behavior of affected data is studied. The
forecasting is done by formulating the dependent
variable using the effects of independent variables.
The variables and abbreviations used in causal
modeling are shown as follow:
OP
t
= OPEC oil demand during time t.
PR
t
= Oil price during time t.
GDP= Gross Domestic Product of countries
which are OPEC oil consumers (OECD).
OE
t
= Demand for other kind-s of energy
during time t.
VAI= Added Value for industrial parts for
countries which are OPEC oil consumers.
The causal models that are obtained are illustrated in
Table 2. This Table shows the equations and also
their analysis.
In the first model, oil demand has a significant
relationship with oil price, GDP and also with other
substitution energies demand (OE). In model (2) the
relationships are logarithmic and independent
variables which have been inputted in the model are
GDP, OE and also VAE. The relationship shows the
price elasticity and also revenue elasticity with oil
demand. Model (3) is a hybrid model consisting
ARIMA and regression model. Like model (3),
model (4) is a hybrid model with combination of
MA (1). Model (5) is a long term oil demand model
with the delay demand which has been inputted in
the model. The demand’s data sets which have been
imported in the model are belonging to previous
year (one year delay). This model (5) is not
considered in combining methods, because of its
correlation within its inputted variables.
In all models p-value<=.05, Determination
coefficient R
2
and adjusted R
2
are approximately
equal to 0 .9., Durbin Watson statistics equal to 2,
and all p-values are significant for variables and
constant quantity. The error results of causal
methods are shown in Table 5.
Step 2: Forecasting Oil Demand by Neural
Networking Method: The supervised back
propagation is widely used for time series
forecasting. Therefore we decided to choose this
well-known method for forecasting OPEC oil
demand. Consequently, normalizing data, training
data and weighting the network’s inputs have been
done. Topology is selected based on continuous
changes, especially changes in the amount of the
hidden layer's neurons. The best Neural Network
Model is (5, 15,1) in which internal layer is with 15
neurons, and one output of oil demand is obtained.
Functions of middle layer are considered as sigmoid
function and transfer function is considered as linear
function. The result of errors of Neural Network
Model is shown in Table 5. The topology is similar
to combined ANN model with different numbers of
neurons and the input layers.
Step 3: Combining Individual Forecasting
Method: In this step, combining individual forecas-
DEVELOPING COMBINED FORECASTING MODELS IN OIL INDUSTRY - A Case Study in Opec Oil Demand
207