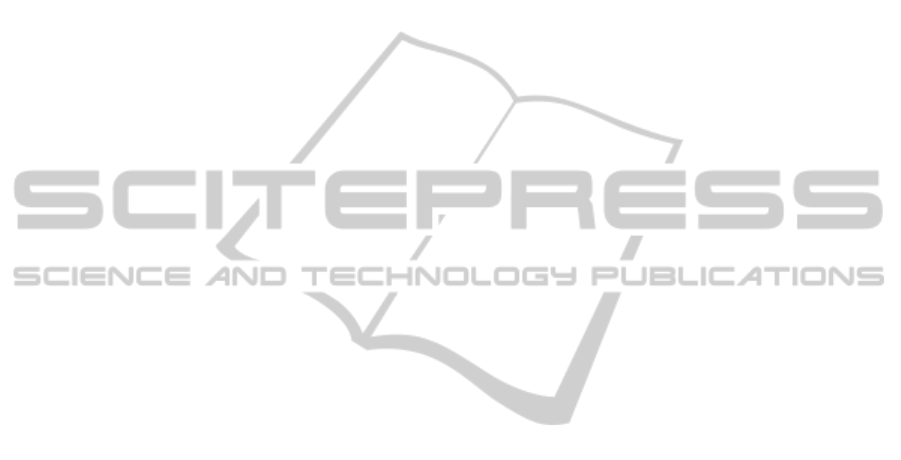
skewness of the observed streamflow series for each
of these time step discretizations. Out the three
performance criteria; (i) periodical mean, (ii)
periodical standard deviation and (iii) skewness
coefficient of the series, ANN was found to be
performing quite well for the periodical standard
deviation and skewness coefficient of the series,
while its performance for periodical mean, was also
found satisfactory and within acceptable limit. Based
on the above analysis, ANN can be regarded as a
competitive alternative method of computing
synthetic streamflow series having potential of better
performance as compared to Thomas-Fiering model.
REFERENCES
Ahmed J A, Sarma A K 2007. Artificial neural network
model for synthetic streamflow generation. Water
Resources Management 21(6):1015-1029
Govindaraju R S 2000. Artificial neural networks in
Hydrology. II: Hydrologic applications. Journal of
Hydrologic Engineering 5(2): 124-137
Bayazıt M 1988. Hidrolojik Modeller, I.T.U. rektorlugu,
Istanbul.
Birikundavyi S, Labib R, Trung H, Rousselle J 2002.
Performance of Neural Networks in Daily Streamflow
Forecasting. Journal of Hydrologic Engineering 7 (5):
392- 398
Box, G E P, Jenkins, G M 1976. Time Series Analysis
Forecasting and Control. San Francisco: Holden-Day
Burian J S, Durrans S R, Nix, S J, Pitt, R E 2001.Training
artificial neural networks to perform rainfall
disaggregation. Journal of Hydrologic Engineering
ASCE 6(1): 43–51
Chandramouli V, Raman H 2001. Multireservoir modeling
with dynamic programming and neural networks.
Journal of Water Resources Planning and
Management 127(2): 89-98
Chandramouli V, Deka P 2005. Neural Network Based
Decision Support Model for Optimal Reservoir
Operation. Water Ressources Management, 19: 447–
464
Diamantopoulou J M, Antonopoulos V Z, Papamichail D
M 2007. Cascade correlation artificial neural networks
for estimating missing monthly values of water quality
parameters in rivers. Water Resources Management
21:649–662
Govindaraju R S, Rao A R 2000. Artificial neural
networks in hydrology. Kluwer Academic Publishers,
Dordrecht
Hagan M T, Demuth H B, Beale M 1996. Neural network
design. PWS/KENT Publishing Co., Boston
Karunanithi N, Grenney W J, Whitley D, Bovee K 1994.
Neural networks for river flow prediction. Journal of
Computing in Civil Engineering ASCE 8 (2):201–220
Kumar D, Raju K, Sathish T 2004. River Flow Forecasting
Using Recurrent Neural Networks. Water Resources
Management, Kluwer Academic Publishers, 18: 143-
161
Loucks D P, Stedinger J R, Haith D A 1981. Water
resources system planning and analysis. Prentice Hall,
Englewood Cliffs, New Jersey
Maass A, Hufschmidt, M M., Dorfman J R, Thomas H A,
Marglin SA, Fair GM 1970. Design of water resources
systems. Harvard University Press, Cambridge
Ochoa-Rivera J C, Andreu J, García-Bartual R 2007.
Influence of Inflows Modeling on Management
Simulation of Water Resources System. Journal of
Water Resources Planning and Management 2:106–
116
Raman H, Sunilkumar N (1995) Multivariate modeling of
water resources time series using artificial neural
networks. Journal of Hydrological Sciences
40(2):145-163
Rumelhart D E, Hinton G E, Williams R J 1986. Learning
internal representations by error propagation,
Parallel distributed processing, Rumelhart DE and
McCleland JL (eds.) vol. 1, Chapter 8, Cambridge,
MA: MIT Press
Srinivasulu S, Jain A 2006. A comparative analysis of
training methods for artificial neural network rainfall–
runoff models. Applied Soft Computing 6: 295–306
Stedinger J R, Taylor, M R 1982. Synthetic streamflow
generation 1: Model verification and validation. Water
Resources Research 18(4): 909–918
Thomas H A, Fiering M B 1962. Mathematical synthesis
of streamflow sequences for the analysis of river
basins by simulation. In: Design of Water Resources
Systems, (Ed. by A. Maas et al.) Chapter 12 Harvard
University Press, Cambridge, Mass
Treiber B, Schultz G A 3/1976. Comparison of required
reservoir storages computed by the Thomas-Fiering
model and the 'Karlsruhe model' Type A and B.
Hydrological Sciences-Bulletin-des Sciences
Hydrologiques- XXI 1(3) 177-185
Yurekli K, Kurunc A. 2004. Performances of Stochastic
Approaches in Generating Low Streamflow Data for
Drought Analysis. Journal of Spatial Hydrology 5(1):
20-32
Zekai Z (6/1978) A mathematical model of monthly flow
sequences. Hydrological Sciences-Bulletin-des
Sciences Hydrologiques 23(2): 223-229
IMPORTANCE OF INPUT PARAMETER SELECTION FOR SYNTHETIC STREAMFLOW GENERATION OF
DIFFERENT TIME STEP USING ANN TECHNIQUES
217