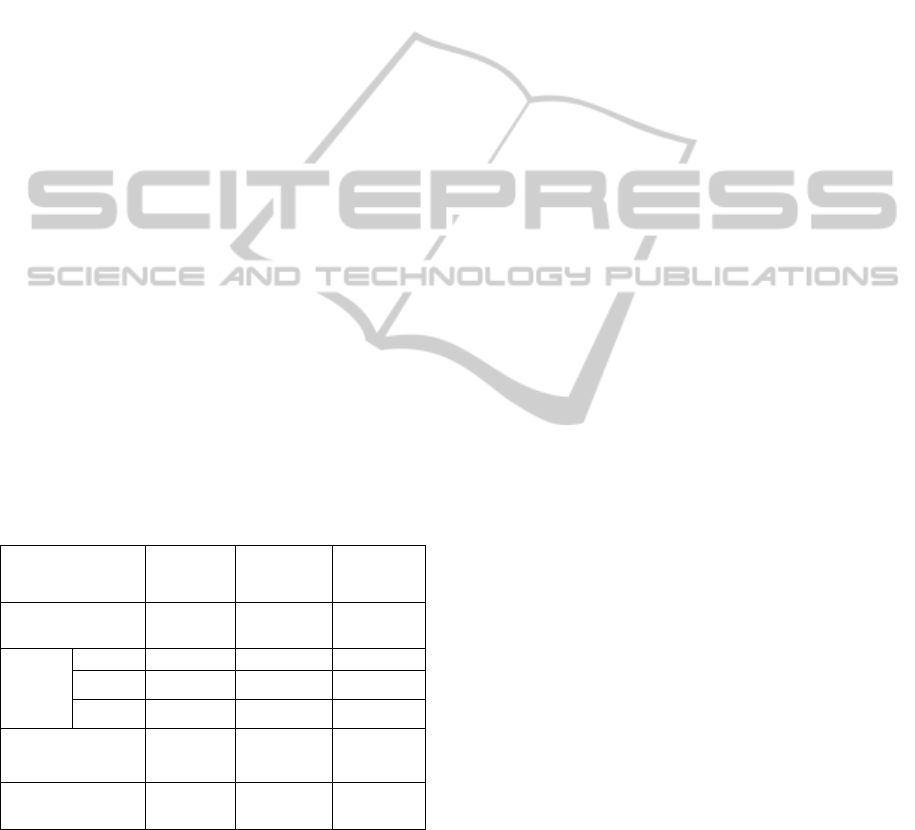
pattern is to open the switch of the branch that has
minimal current value in loop, with the optimal flow
pattern were to compute load flow of initial radial
network, it will follow by the closing of all normal
switches to produce loop networks, this will
continue by computing the equivalent injection
current at all nodes in loops through injecting
current method, and the replacement of the branch
impedance by corresponding branch resistance in the
loop and then compute the optimal flow, the network
will have a new reconfiguration by opening a switch
branch that has a minimal current value in loop. And
can again recomputed the load flow for the remained
network, this will end by opening the next branch
switch, and repeat the previous step discussed above,
until we have the radial network.
4 RESULTS AND DISCUSSION
The following case illustrates the loss reduction
through network reconfiguration of the tested system
of a 6 bus radial distribution. To evaluate the
proposed techniques, it has been applied to 6 bus
radial distribution system loss reduction. The results
obtained from the combination PSO, and as been
validated by Neural Network with the control of the
tie switch, it shows that it succeed in finding the
global solution with a high probability in the system
as represented in table 1
Table 1: Results of 6 bus radial distribution network
reconfiguration.
Description Initial State PSO
Neural
Network
Tie Switch open Switch 1-2 Switch 3-2
Switch 3-5
Switch 3-2
Switch 3-5
Total
Power
Loss
(MW)
Best ------------- 0.0096525 0.009652
Average ---------- 0.00117044 0.00117044
Worst 0.0095452 0.0035428 0.0035428
Average Power Loss
Reduction (%)
62.23 62.23
Minimum Voltage
Magnitude (p.u.)
0.894 0.978 0.978
5 CONCLUSIONS
Loss reduction is very important complement to
network and feeder reconfiguration. In this paper the
network and feeder reconfiguration problem was
formulated as loss minimization problem with the
view for its solution to control different switches
placed in the network. Two MATLAB based
solution methods have been proposed and
demonstrated. First is the PSO and the other is
neural network-base technique. The proposed
methods were successfully tested. From the practical
point of view these method can be very effective as
several model based approaches usually take very
long running time. The PSO has been found more
suitable and faster compared to neural network. But
both methods gives good results on loss reduction in
the network.
REFERENCES
T. H. Chen and J. T. Cherng, "Optimal Phase
Arrangement of Distribution Transformers Connected
to a Primary Feeder for System Unbalance
Improvement and Loss Reduction Using Genetic
Algorithm," IEEE Trans. Power systems, vol. 15,
August, 2000.
C. C. Liu, S. J. Lee, and K. Vu, "Loss Minimization of
Distribution Feeders: Optimality and Algorithms,"
IEEE Trans. Power delivery, vol. 4, April, 1989.
A. Ukil, M. Siti, and J. Jordaan, "Feeder Load Balancing
Using Neural Network," in Springer, Computer
Science, 2006.
J. Zhu, Optimization of Power System Operation.
Hoboken, New Jersey: John Wiley & Sons, Inc., 2009.
C. B. Alexandre, A. Carlos, and Newton, "Main Chain
Representation for Evolutionary Algorithms Applied
to Distribution System Reconfiguration," IEEE Trans.
Power systems, vol. 20, Febrary, 2005.
A. Auguglio, L. Dusonchet, and M. Giuseppe, "Minimum
Losses Reconfiguration of MV distribution Networks
through Local Control of Tieswitches," IEEE Trans.
Power delivery, vol. 18, July, 2003.
M. E. Baran and F. F. Wu, "Network Reconfiguration in
Distribution Systems for Loss Reduction and Load
Balancing," IEEE Trans. Power delivery, vol. 7, 1989.
M. Siti, D. V. Nicolae, A. A. Jimoh, and A. Ukil,
"Reconfiguration and Load Balancing in LV and MV
Distribution Networks for Optimal Performance,"
IEEE Trans. Power delivery, vol. 22, pp. 2534-2540,
2007.
X. Yang, S. P. Carull, and K. Miu, "Reconfiguration,
Distribution, Automation and Control Laboratory:
Multiphase Radial Power Flow Experiment," IEEE
Trans. Power systems, vol. 20, August, 2005.
W. YU, HE, H. & ZHANG, N., "Optimal Reactive Power
Dispatch Using Particle Swarms Optimization
Algorithm Based Pareto Optimal Set," Berlin:
Springer-Verlag Berlin Heidelberg 2009, 2009.
A. Zaraki and M. F. Bin Othman, "Implementing Particle
Swarm Optimization to Solve Economic Load
Dispatch Problem," in Soft Computing and Pattern
Recognition, 2009. SOCPAR '09. International
NCTA 2011 - International Conference on Neural Computation Theory and Applications
222