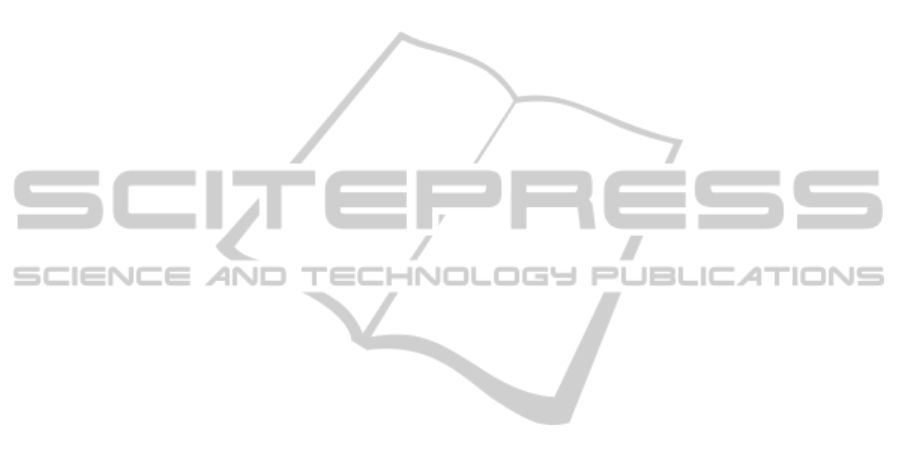
5 DISCUSSION AND FUTURE
WORK
The spiking neural network presented in this paper is
constructed using a hierarchical structure that is
composed of spiking neurons with various receptive
fields. The input image is converted to retinal
ganglion cell output spike trains by convolving with
DoG filters. The spike trains are presented to the
network and the various receptive fields process the
image, performing edge detection and corner
detection. The spiking neuron models provide
powerful functionality for integration of inputs and
generation of spikes. Synapses are able to perform
different complicated computations. This paper
demonstrates how a spiking neural network can
detect edge and corners in an image. The
performance illustrates that the proposed detector is
currently only capable of detecting simple edges at
specific orientations and similarly only particular
corner types. However, the current results appear
promising when compared with the standard Harris
approach to corner detection. Future work will
involve the incorporation of biologically plausible
unsupervised learning algorithms (STDP) to set the
synaptic weights, automatic development of
receptive fields to deal with different edge and
corner types.
ACKNOWLEDGEMENTS
This work was supported by the Centre of
Excellence in Intelligent Systems project, funded by
InvestNI and the Integrated Development Fund.
REFERENCES
Chevallier, S., Tarroux, P. and Paugam-Moisy, H., (2006).
Saliency extraction with a distributed spiking neural
network. ESANN'06.
Escobar M. J., Masson G. S., Vieville T. and Kornprobst
P., (2009). Action Recognition Using a Bio-Inspired
Feedforward Spiking Network. International Journal
of Computer Vision. 82, 3, 284-301.
Gerstner, W. and Kistler, W., (2002). Spiking Neuron
Models: Single Neurons, Populations, Plasticity,
Cambridge University Press, 2002.
Goodman D. F. and Brette R., (2009). The Brian
simulator, Front. Neurosci. 3, 2, 192-197.
Harris, C. and Stephens, M., (1988). A Combined Corner
and Edge Detector. Proceedings 4th Alvey Vision
Conf. 147-151.
Hodgkin, A. and Huxley, A. (1952). A quantitative
description of membrane current and its application to
conduction and excitation in nerve. Journal of
Physiology, London, 117, 500-544.
Hugues, E., Guilleux, F. and Rochel, O., (2002). Contour
Detection by synchronization of Integrate and Fire
Neurons. BMVC, 2002.
Izhikevich, E.M., Which model to use for cortical spiking
neurons? IEEE Trans. on Neural Networks. 15, 5.
Masquelier, T. and Thorpe, S. J. (2010). Learning to
recognize objects using waves of spikes and Spike
Timing-Dependent Plasticity. The 2010 International
Joint Conference on Neural Networks (IJCNN). 1-8.
Meftah, B., Lezoray, O. and Benyettou, A. (2010).
Segmentation and Edge Detection based on Spiking
Neural Network Model. Neural Processing Letters,
32, 2, 2010.
Moravec, H. P., (1977) Towards Automatic Visual
Obstacle Avoidance. Proceedings 5th Int. Joint
Conference. Artificial Intelligence. Cambridge, MA,
USA. 584.
O’Connor, D. H., Huber, D., and Svoboda, K. (2008).
Reverse engineering the mouse brain. Nature 461,
923-929.
Shapley, R. M. and Tolhurst, D. J., (1973). Edge detectors
in human vision. Journal of Physiology. 229, 1, 165-
183.
Shen, F. and Wang, H., (2001). A Local Edge Detector
Used for Finding Corners. Proceedings 3rd Int. Conf.
Inf, Comm. And Signal Processing. Singapore.
Smith, S. M. and Brady, J. M., (1997). SUSAN – A New
Approach to Low Level Image Processing. IJCV. 23,
1, 45-78.
van Rullen R. and Thorpe S., (2002). Surfing a spike wave
down the ventral stream. Vision Research. 42, 2593-
2615.
Wu, Q. X., McGinnity, T. M., Maguire, L. P., Glackin B.
and Belatreche, A., (2007a). Information Processing
Functionality of Spiking Neurons for Image Feature
Extraction. 7th Int. Workshop on Information
Processing in Cells & Tissue. Jesus College, Oxford,
UK.
Wu, Q. X., McGinnity, T. M., Maguire, L. P., Belatreche,
A., and Glackin, B., (2007b). Edge Detection Based
on Spiking Neural Network Model. Proc of ICIC.
SPIKING HIERARCHICAL NEURAL NETWORK FOR CORNER DETECTION
235