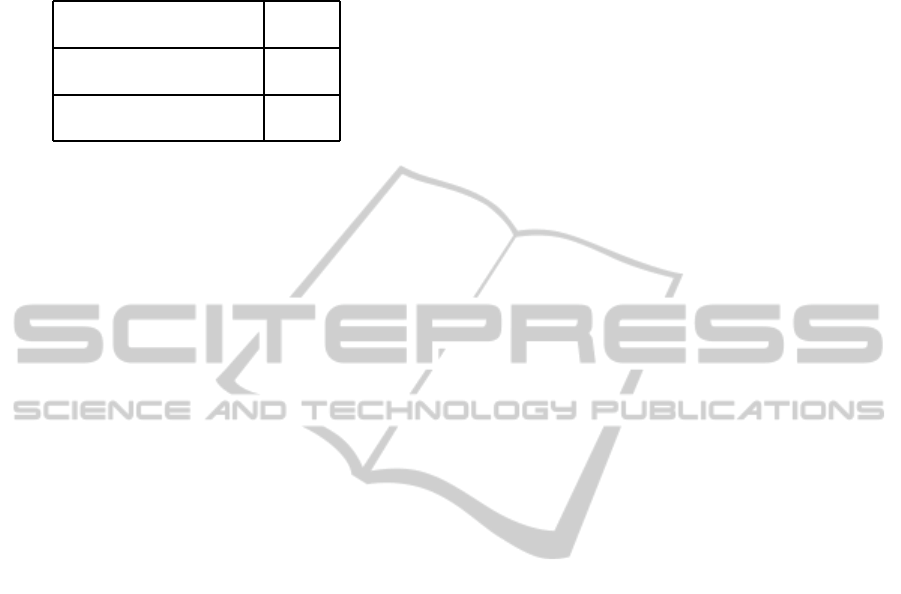
set (90%) and a test set (10%). The results in terms
of classification accuracy are presented in Table 1.
Table 1: Classification Accuracy.
Ensemble of TSVM 67.7%
CB features
TSVM 62.5%
Rating features
Ensemble of TSVM 68.3%
CB and Rating features
As presented in Table 1, the ensemble of TSVM
classifiers based on content-based features yielded to
higher performance than the performance of classi-
fiers based on feature vectors constructed by the rat-
ings of other users. In other words, the content-
based semantic information can describe more effi-
ciently the preferences of users than the opinion of
other users for a specific item. Finally, the ensemble
of TSVM classifiers based on the aggregation of all
available features, improves slightly the accuracy of
the ensemble with only content-based feature vectors.
5 CONCLUSIONS AND FUTURE
WORK
In this paper, we addressed the movie recommen-
dation process as a classification problem. Specifi-
cally, we followed an approach based on an ensem-
ble of classifiers, each of which was fed with differ-
ent feature vectors extracted from different sematic
information about movie. Each classifier was based
on Transductive Support Vector Machines which en-
hances their ability to embed unlabeled data in the
decision making process and results in better per-
formance when the available datasets are highly un-
balanced. Our recommendation method has been
evaluated on the MovieLens dataset. We found that
the content-based semantic information can describe
more efficiently the preferences of users rather than
the opinion of other users, represented as ratings of
items.
Currently, we are in the process of conducting fur-
ther experiments and improvements to our system by
extending the proposed method into a hybrid cascade
recommender system (Lampropouloset al., 2011) and
by applying differenttypes of classifiers (Lampropou-
los et al., 2010). This and other related research work
is currently in progress and will be reported elsewhere
in the near future.
REFERENCES
Billsus, D. and Pazzani, M. J. (2000). User modeling
for adaptive news access. User Modeling and User-
Adapted Interaction, 10(2-3):147–180.
Burke, R. (2002). Hybrid recommender systems: Survey
and experiments. User Modeling and User-Adapted
Interaction, 12(4):331–370.
Burke, R. (2007). Hybrid web recommender systems. In
Brusilovsky, P., Kobsa, A., and Nejdl, W., editors,
The adaptive web, pages 377–408. Springer-Verlag,
Berlin, Heidelberg.
Chapelle, O., Sch¨olkopf, B., and Zien, A. (2006). Semi-
Supervised Learning. The MIT Press, Cambridge,
Massachusetts, London, England.
Cherkassky, V. and Mulier, F. M. (2007). Learning from
Data: Concepts, Theory, and Methods. Wiley-IEEE
Press.
Christakou, C. and Stafylopatis, A. (2005). A hybrid movie
recommender system based on neural networks. In
Proceedings of the 5th International Conference on
Intelligent Systems Design and Applications, ISDA
’05, pages 500–505, Washington, DC, USA. IEEE
Computer Society.
Claypool, M., Gokhale, A., Miranda, T., Murnikov, P.,
Netes, D., and Sartin, M. (1999). Combining content-
based and collaborative filters in an online newspaper.
In Proc. ACM SIGIR Workshop on Recommender Sys-
tems.
Herlocker, J. L., Konstan, J. A., and Riedl, J. (2000). Ex-
plaining collaborative filtering recommendations. In
Proceedings of the 2000 ACM conference on Com-
puter supported cooperative work, CSCW ’00, pages
241–250, New York, NY, USA. ACM.
Herlocker, J. L., Konstan, J. A., Terveen, L. G., and Riedl,
J. T. (2004). Evaluating collaborative filtering recom-
mender systems. ACM Trans. Inf. Syst., 22:5–53.
IMDB (2010). The internet movie database. Database avail-
able at http://www.imdb.com/interfaces#plain.
JMDB (2009). Java movie database. Software available at
http://www.jmdb.de/.
Joachims, T. (1999). Transductive inference for text classifi-
cation using support vector machines. In Proceedings
of the Sixteenth International Conference on Machine
Learning, ICML ’99, pages 200–209, San Francisco,
CA, USA. Morgan Kaufmann Publishers Inc.
Joachims, T. (2008). Svmlight: An implementation
of support vector machines. Software available at
http://svmlight.joachims.org/.
Kuncheva, L. I. (2004). Combining Pattern Classifiers
Methods and Algorithms. Wiley, New York, NY, USA.
Lampropoulos, A. S., Lampropoulou, P. S., and Tsihrintzis,
G. A. (2011). A cascade-hybrid music recommender
system based on musical genre classification and per-
sonality diagnosis for mobile services. Multimedia
Tools and Applications, pages 1–18. 10.1007/s11042-
011-0742-0.
Lampropoulos, A. S., Sotiropoulos, D. N., and Tsihrintzis,
G. A. (2010). A music recommender based on artifi-
cial immune systems. In Howlett, R. J., Jain, L. C.,
NCTA 2011 - International Conference on Neural Computation Theory and Applications
246