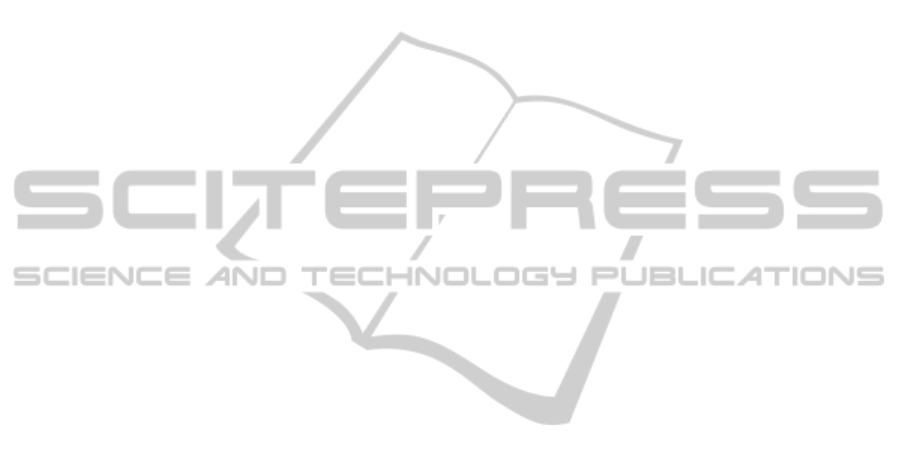
best, model II the second, and model III the worst
with regards to both predictions, width and cost.
This indicates that these estimators work together in
harmony and have been well chosen. These results
empirically establish their use as criteria for
selecting both the best ANN configuration for a
developed model and the best model that describes a
system or a problem.
5 CONCLUSIONS
DoE was used to design an arranged series of
information-gathering experiments to characterise
micro-channel formation using a Nd:YVO
4
laser.
The relationship between the main laser process
parameters and the developed micro-channel width
and corresponding micro-machining operating cost
was examined using feed-forward, back-propagation
ANN predictive models. The influence of changing
the number and the selection of training data on the
prediction capability of the developed ANN
predictive model was investigated. MSE (Mean
Squared Error), R2 (The coefficient of
determination), and MAPE (Mean Absolute
Percentage Error) were utilised as a basis for
comparison between the developed ANN predictive
models.
The comparison showed that model I (which has
the highest number of training data) was the best.
Moreover, model II is better than model III (both
have almost the same number of training data but
different training data set). This indicates that the
more training data employed the better model fit
acquired. However, when limited number of
experiments (training data) is allowed, the outcomes
of this work favoured using FCC Design over BB
design for the selection of training data. This result
indicates that using FCC design for training data
selection was found more efficient in predicting
width and micro-machining cost and highlighted the
importance of including all experimental data space
corner points in any training data set. Moreover, this
comparison showed that the ANN modelling
technique can be smoothly employed to predict the
laser machined micro-channel dimensions and
production cost precisely.
Automated systems control can allow the use of
the models presented in this paper in order to
produce optimised micro-channels with high
dimensional precision and least production cost.
It was established in this work that the developed
ANN predictive models were efficient at satisfying
these demands and were effective for the prediction
of the most appropriate laser micro-machining
parameters.
REFERENCES
Collins, M. and DeLucca, M. 2011. Neural networks
[Online]. Available from: www.academic.marist.edu/
~jzbv/architecture/
Projects/S2002/NeuralNet2/COA.PPT, [accessed 27 July
2011].
de-Deus, A. M. and Mazumder, J. 1996. Two-
Dimensional Thermo-Mechanical Finite Element
Model for Laser Cladding, Laser Materials
Processing; Detroit, Michigan; USA, B174-B183
Karazi, S. M., Issa, A. and Brabazon, D. 2009.
Comparison of ANN and DoE for the prediction of
laser-machined micro-channel dimensions. Optics and
Lasers in Engineering, 47 (9), p. 956-964.
Karazi, S. M. and Brabazon, D. 2010. Analysis and
prediction of dimensions and cost of laser micro-
machining internal channel fabrication
process, International Symposium on Experimental
Mechanics ICEM14, Poitiers, France, EPJ Web of
Conferences, V 6, p. 25002.
Lee, S. H., Park, W.S., Cho, H. S., Zhang, W. and Leu, M.
C. 2001. A neural network approach to the modelling
and analysis of stereolithography processes,
Proceedings of the Institution of Mechanical
Engineers, Journal of Engineering Manufacture, 215,
p. 1719-1733.
Shen, H., Shi, Y. J.., Yao, Z. .Q and Hu, J. 2006. Fuzzy
logic model for bending angle in laser forming,
Materials Science and Technology, 22, p. 981-986.
Wang, G. G. and Xie, S. Q. 2005. Optimal process
planning for a combined punch-and-laser cutting
machine using ant colony, International Journal of
Production Research, 43, p. 2195 – 2216.
Ye, J., Yuan, X. C. and Zhou, G. 2003. Genetic algorithm
for optimization design of diffractive optical elements
in laser beam shaping, Proceedings of SPIE, 4594, p.
118-127.
EVALUATION OF THE EFFECT OF ND:YVO4 LASER PARAMETERS ON INTERNAL MICRO-CHANNEL
FABRICATION IN POLYCARBONATE
259