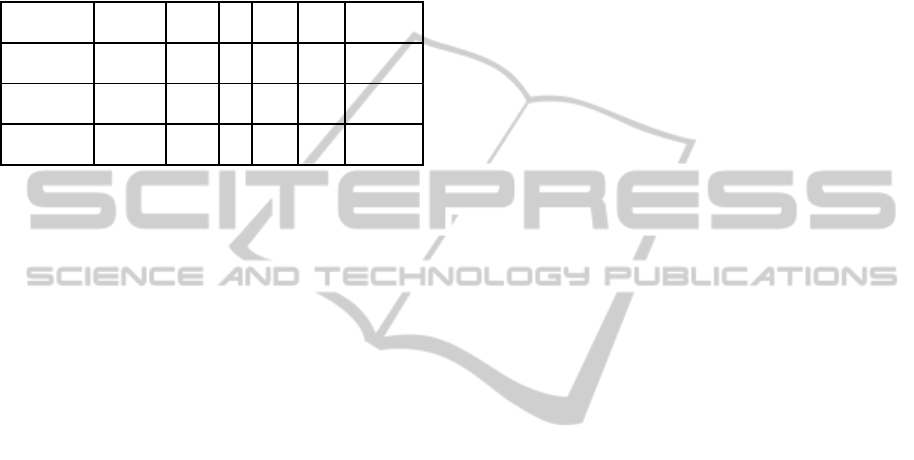
various Stock Market Indexes in Europe(V´ehel and
Walter, 2002). Here, γ is the scale factor and the
threshold is 1% (confidence level of 99%). As shown
by the data, all these indexes clearly have a L´evy dis-
tribution and the value of the stability exponent is typ-
ically around 1.7, which is not in the Gaussian regime.
Table 2: Estimation of the parameters of the L´evy distri-
butions associated with various Stock Exchange Indexes in
Europe (V´ehel and Walter, 2002).
Index Currency Period N α γ
Threshold
(1%)
FTA W
GBP
86.01
93 1.716 2.690 1.1408
Europe -93.09
MSCI
USD
80.01
165 1.713 2.936 0.1057
Europe -93.09
MSCI
USD
80.01
165 1.719 2.951 0.1057
EUR ex UK -93.09
Stock market data is not the only type of data that
are suspect to such large data fluctuation that does
not have a Gaussian distribution. As previously men-
tioned, the sales of ketchup are another example of
such data. Also, the damages caused by natural dis-
asters such as hurricanes, tornados and earthquakes,
fall within this domain. Using the standard data pre-
processing techniques, and incorrectly assuming that
the standard limit theorem holds in such cases, has
grave impact on the validity of the resultant models
constructed. This is especially true in domains where
the data are aggregated prior to model building. As
mentioned earlier, the vast size of massive data min-
ing repositories necessitates aggregation, due to the
sheer size and complexity of the data being mined.
4 CONCLUSIONS
This position paper challenges the implicit assump-
tion, which is often made during numerous data min-
ing exercises, that the standard limit theorem holds
and that the data distribution is Gaussian. We dis-
cuss the implications of this assumption, especially
in terms of aggregated data that is characterised with
large fluctuations. We show the nature of the differ-
ences between the Gaussian and L´evy distributions,
on synthetic data and show an example from the real-
world financial stock market data. We observe that the
two sets of distributions are vastly different, and that
it follows that, during any data mining exercise, that
data with a Levy distribution should be treated with
caution, especially during data pre-processing and ag-
gregation.
The implications and applications of this observa-
tion are far-reaching in many domains. It has been
shown that the value of a share is usually dominated
by a few large fluctuations. Damages associated with
earthquakes and tsunamis, such as those caused by
the recent events in Japan, are also characterized by
such large fluctuations. The same observation holds,
e.g., when observing the sizes of solar flares or craters
on the moon, as well as for the data obtained from
many climate change studies. This fact needs to be
taken into account, when aiming to create valid data
mining models for these types of domains, which are
becoming increasingly important for socio-economic
reasons.
REFERENCES
Groot, R. D. (2005). L´evy distribution and long correlation
times in supermarket sales. Lvy distribution and long
correlation times in supermarket sales, 353:501–514.
Han, J., Kamber, M., and Pei, J. (2006). Data Mining: Con-
cepts and Techniques (2nd edition). Morgan Kauff-
man.
Samoradnitsky, G. and Taqqu, M. (1994). Stable Non-
Gaussian Random Processes: Stochastic Models with
Infinite Variance. Chapman & Hall, New York.
V´ehel, J. L. and Walter, C. (2002). Les march´es fractals
(The fractal markets). Universitaires de France, Paris.
Walter, C. (1999). L´evy-stability-under-addition and fractal
structure of markets: implications for the investment
management industry and emphasized examination of
matif notional contract. Mathematical and Computer
Modelling, 29(10-12):37–56.
TO AGGREGATE OR NOT TO AGGREGATE: THAT IS THE QUESTION
357