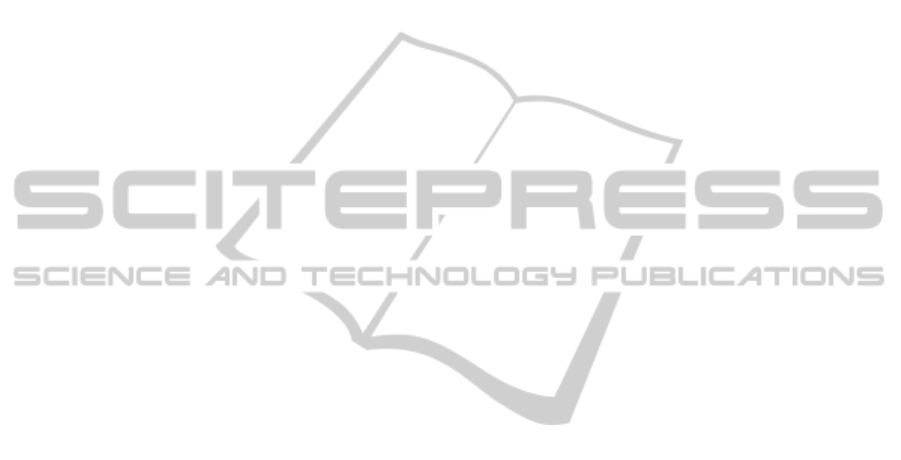
music experience instead of simply listening to what
the station chooses to webcast.
Last.fm (http://www.last.fm/) uses collaborative
filtering to recommend music you might like.
Collaborative filtering works by keeping track of
songs the user likes and listens to and is based on the
assumption that the musical taste of the specific
listener can be matched against the community.
Last.fm uses what they refer to as Scrobbling to
keep track of the music you listen to for use with
collaborative filtering techniques and to chart
popular music. One of the drawbacks of
collaborative filtering is that it only works well with
popular songs because songs lesser known might not
have substantial data. Another problem with this
approach is that it does not take into account the
structure of the song.
Musicovery (http://musicovery.com/) allows
users to intuitively find music based on their mood
and preferences for different genres and time
periods. This is accomplished through a graphical
interface based on Liveplasma technology and the
"mood pad". Forty musical parameters are used to
describe each song and place it on the mood pad
according to the likeliness that the song will please
the user according to their selected mood. Mood is
selected on a two dimensional scale with the y axis
ranging from calm to energetic and the x axis
ranging from dark to positive.
Pandora (http://www.pandora.com/) bases its
music recommendation on the Music Genome
Project. The Music Genome is a database of songs
where each song is described by a set of
characteristics. Each characteristic is referred to as a
gene and different songs have different numbers of
genes. For example, a rock song may have 150
genes while a classical song may have 500 genes.
These genes are collected into logical groups called
chromosomes and a set of chromosomes make up a
genome. Musicologists carefully classify each song
in the database by this method so that songs can be
compared on the basis of their genes taking into
account that some genes are more important than
others.
Listeners can tune into a currently playing station
or create their own personalized station. Stations are
started by entering the name of a song, artist, or
album which is referred to as the seed of the station.
This seed is then examined to determine which
distinguishing aspects of a song are present in its
genome and populate the station with songs with
similar features. While listening to music users can
provide feedback regarding whether or not they like
a selected song. Future music selections use this
feedback to refine the importance of each gene to an
individual listener.
Users can listen to Pandora online or on the go
with Pandora mobile. In the last two years Pandora
has been integrated into many telematics systems
and constitutes a popular vehicle infotainment
option.
In general Internet music stations provide
listeners with music they like based on feedback
from the listener. They usually allow users to create
multiple stations based on specific music features,
such as an artist or genre. Users can switch between
multiple stations or allow the system to switch for
them through a random shuffle mode, such as
Pandora mix mode. Since this shuffle is random,
these systems do not take into account the listeners
environment.
More recently products are being developed
which include music selection based on the user's
current environment and personal context.
Nike+ is one example, which is currently widely
available to consumers. This system has users place
a sensor in the bottom of their running shoe that
keeps track of each step taken. A user can then
choose between using the Nike+ Sportband or their
iPod. Either option provides runners with
information about their pace, distance, calories
burned, and more. After a run, users can log on to
the Nike+ webpage and upload information from
their run to track their progress, set goals, and
connect with other runners.
If paired with an iPod nano, users can create their
own personalized playlists to accompany their
workouts or download popular workout playlists
(referred to as Sport iMixes) from iTunes. US patent
20060107822 A1 filed by Apple claims the
capability of affecting a user's mood and behaviour
during an activity, such as exercise, by controlling
the speed of the music being listened to. The speed
of the music can either be selected to match the pace
of the activity or to drive the pace of the activity.
In a research context, several universities have
projects underway which aim to use Bio feedback to
select the music being played. The hypothesis
behind these applications is that a user's context
affects what they want to listen to. The University of
Maryland Baltimore County has developed a
concept called XPod which is intended to reside on a
PDA (Dornbush, 2007). Once XPod is initiated, a
BodyMedia SenseWear device is used to wirelessly
transmit data to a server to determine the user's level
of activity and emotion. Data collected by the
BodyMedia device includes transversal and
longitudinal acceleration, galvanic skin response,
INTELLIGENT MUSIC SELECTION TO INFLUENCE DRIVER BEHAVIOUR - An Empirical Study
85