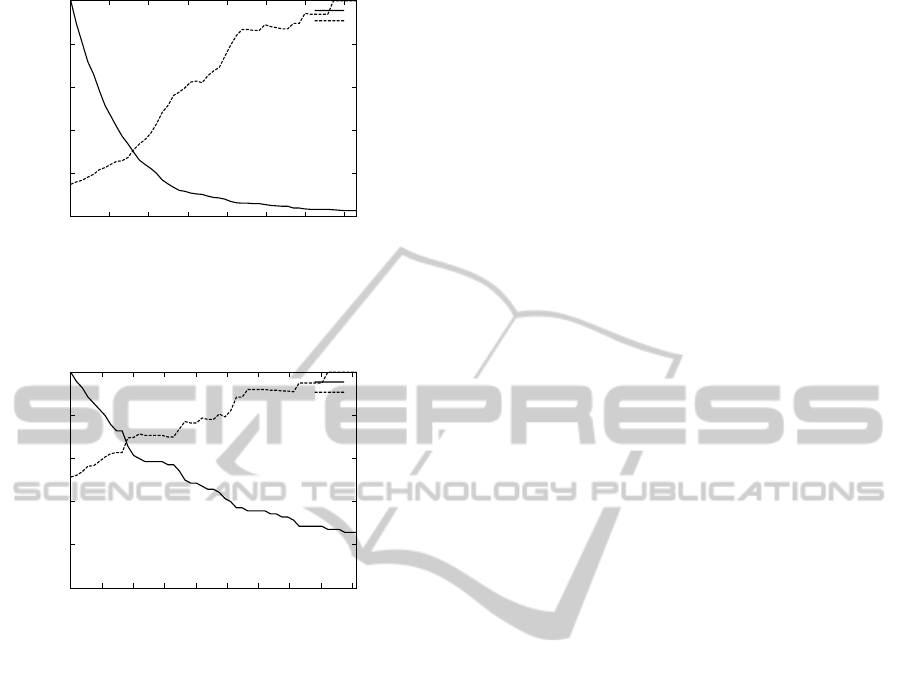
0
20
40
60
80
100
0 0.01 0.02 0.03 0.04 0.05 0.06 0.07
Number of classified faces [in %]
Recognition rate [in %]
Threshold
Classified
Recognition rate
Figure 4: Relative Confidence Value method: Face recog-
nition rates and numbers of the classified faces when one
training example is used (T ∈ [0;1]).
0
20
40
60
80
100
0 0.001 0.002 0.003 0.004 0.005 0.006 0.007 0.008 0.009
Number of classified faces [in %]
Recognition rate [in %]
Threshold
Classified
Recognition rate
Figure 5: Relative Confidence Value method: Face recogni-
tion rates and numbers of the classified faces when all seven
training examples are used (T ∈ [0;1]).
5 CONCLUSIONS AND
PERSPECTIVES
The main contribution of this work is the proposition
and evaluation of two confidence measure techniques
as a post-processing of the automatic face recognition
task. We suggest using a confidence measure due to
the relatively low face recognition rate on the
ˇ
CTK
corpus. The experiments show that around 10% of
the images is classified with nearly 100% accuracy
by the Absolute confidence value approach. We fur-
ther show, that the second proposed approach, Rel-
ative confidence value method, is more suitable for
the practical use. Around 30% of images is classified
with an accuracy close to 100% by this method.
The perspectives of this work are numerous, in-
cluding evaluation of the methods on the larger real-
word corpora (the faces are not well aligned and the
lighting conditions differ), the development of more
sophisticated confidence measures or in adding the
pre-processing step, i.e. eye localization and the sub-
sequent image rotation and aligning.
ACKNOWLEDGEMENTS
This work has been partly supported by
ˇ
CTK and by
the UWB grant SGS-2010-028 Advanced Computer
and InformationSystems. We also would like to thank
ˇ
CTK for providing the photographic data.
REFERENCES
Bledsoe, W. W. (1966). Man-machine facial recognition.
Technical report, Panoramic Research Inc., Palo Alto,
CA.
Bolme, D. S. (2003). Elastic Bunch Graph Matching. PhD
thesis, Colorado State University.
Jiang, H. (2005). Confidence measures for speech recog-
nition: a survey. Speech Communication, 45(4):455–
470.
Kanade, T. (1977). Computer recognition of human faces.
Birkhauser Verlag.
Kepenekci, B. (2001). Face Recognition Using Gabor
Wavelet Transform. PhD thesis, The Middle East
Technical University.
Lades, M., Vorbr¨uggen, J. C., Buhmann, J., Lange, J., and
von der Malsburg, C. (1993). Distortion invariant
object recognition in the dynamic link architecture.
IEEE Transactions On Computers.
Lawrence, S., Giles, S., Tsoi, A., and Back, A. (1997).
Face recognition: A convolutional neural network ap-
proach. IEEE Trans. on Neural Networks.
Lleida, E. and Rose, R. C. (1996). Likelihood Ratio Decod-
ing and Confidence Measures for Continuous Speech
Recognition. In ICSLP’96, volume 1, pages 478–481,
Philadelphia, USA.
Nefian, A. V. and Hayes, M. H. (1998). Hidden markov
models for face recognition. In IEEE International
Conference on Acoustics, Speech, and Signal Process-
ing.
Shen, L. (2005). Recognizing Faces - An Approach Based
on Gabor Wavelets. PhD thesis, University of Not-
tingham.
Shen, L. and Bai, L. (2006). A review on gabor wavelets
for face recognition. Pattern Analysis & Applications.
Sirovich, L. and Kirby, M. (1987). Low-dimensional proce-
dure for the characterization of human faces. Journal
of the Optical Society of America, 4.
Turk, M. A. and Pentland, A. P. (1991). Face recognition
using eigenfaces. In IEEE Computer Society Confer-
ence on In Computer Vision and Pattern Recognition.
Computer Vision and Pattern Recognition.
Wiskott, L., Fellous, J.-M., Kr¨uger, N., and von der Mals-
burg, C. (1999). Face recognition by elastic bunch
graph matching. Intelligent Biometric Techniques in
Fingerprint and Face Recognition.
KDIR 2011 - International Conference on Knowledge Discovery and Information Retrieval
368