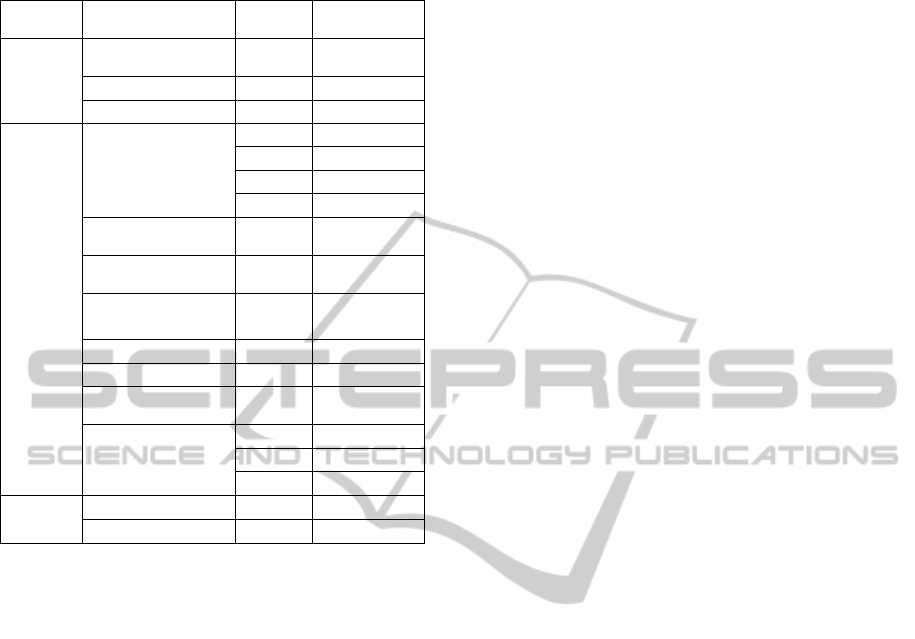
Table 2: MSE values obtained with the proposed hybrid
algorithm and other authors’ algorithms.
Function Algorithm
Number
of Rules
MSE
c
(Mitaim et al.,
1996)
12 1.426
(Lisin et al., 1999) 12 0.247
Hybrid algorithm 12 0.0169
d
(Rojas et al., 2000)
9 0.146
16 0.051
25 0.026
36 0.017
(Sugeno et al.,
1993)
6 0.079
(Nozaki et al.,
1997)
25 0.0085
(Teng et al., 2004) 4 0.016
(Lee, 2008) 3 0.0028
(Wang et al,. 2005) 3 0.0052
(Tsekouras et al.,
2005)
6 0.0108
Hybrid algorithm
9 0.0168
16 0,0018
25 0.0002
e
(Lee, 2008) 25 less than 0.001
Hybrid algorithm 25 0.00009
5 CONCLUSIONS
The results of the experiment allow to conclude that:
• The suggested hybrid algorithm based on genetic
algorithm and derivative based methods provides
better results than each method separately;
• The suggested hybrid algorithm for fuzzy models
tuning allows to achieve smaller error values in most
cases compared to existing analogues.
ACKNOWLEDGEMENTS
This paper is supported by Russian Foundation for
Basic Research (09-07-99008).
REFERENCES
Mitaim, S., Kosko, B., 1996. What is the best shape for a
fuzzy set in function approximation? In Proc. Fifth
IEEE Int. Conf Fuzzy Systems, vol. 2. New Orleans
Lee, Zne-Jung, 2008. A novel hybrid algorithm for
function approximation. Expert Systems with
Applications, vol. 34.
Lisin, D., Gennert M.A., 1999. Optimal Function
Approximation Using Fuzzy Rules. In Proc. Int. Conf.
North American Fuzzy Information Processing
Society.
Rojas, I., Pomares, H., Ortega, J., Prieto, A., 2000. Self-
organized fuzzy system generation from training
examples. In IEEE Transactions on Fuzzy Systems,
vol. 8 (1).
Nozaki, K., Ishibuchi, H., Tanaka H., 1997. A simple but
powerful method for generating fuzzy rules from
numerical data. In Fuzzy Sets and Systems, vol. 86.
Sugeno, M., Yasukawa, T., 1993. А fuzzy-logic-based
approach to qualitative modeling. In IEEE
Transactions on Fuzzy Systems. vol.1, no. 1.
Teng, Y., Wang, W., Chiu, C.H., 2004. Function
approximation via particular input space partition and
region-based exponential membership functions. In
Fuzzy Sets and Systems, vol. 142.
Tsekouras, G., Sarimveis, H., Kavakli, E., Bafas G., 2005.
A hierarchical fuzzy-clustering approach to fuzzy
modeling. In Fuzzy Sets and Systems, vol. 150.
Wang, H., Kwong, S., Jinb Y., Wei, W., Man, K.F., 2005.
Multi-objective hierarchical genetic algorithm for
interpretable fuzzy rule-based knowledge extraction.
In Fuzzy Sets and Systems, vol. 149.
HYBRID ALGORITHM FOR FUZZY MODEL PARAMETER ESTIMATION BASED ON GENETIC ALGORITHM
AND DERIVATIVE BASED METHODS
515