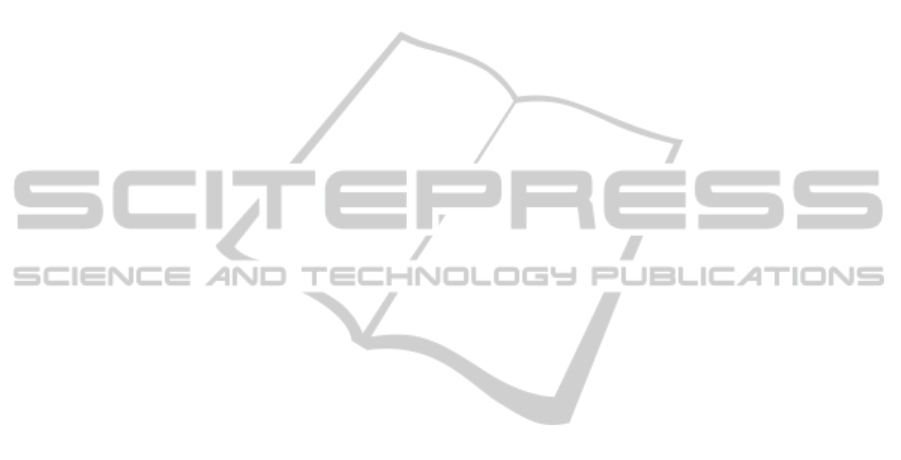
minProfit, maxRisk and minWinRate are 10, 30 and
30%, respectively. After mining the TDB of Table 1,
we found fourteen profit rules as shown in Table 3.
For Rule No. 8, the BP is equal to its SP meaning
that investors must sell their stocks first when they
hold no stock, or they will lose money. For the rules
No. 2 and No. 3, their BP, SP and trading results are
the same. It means that investors can adopt buy-first
or sell-first since their trading results are the same
either way.
5.2 Discussion
As a preliminary study on financial data mining, we
use a simple trading model of inter-day model for
trading simulation. With different trading models,
one can derive many different trading rules under
specific transaction databases.
In data mining research, one expects to mine the
knowledge of any kind that users might be interested
in. These useful results of knowledge can be
discovered and presented in the forms of rules,
patterns, or any other forms to meet users’
expectation.
Similarly in profit mining, we think more types
of profit rules can be defined and discovered in any
of the financial sectors. Since investors have their
own measurement criteria for mining the preferred
trading rules, there must be more useful models of
financial data mining to be investigated.
6 CONCLUSIONS
In this paper, we present a new data mining in the
financial market, called profit mining, with initial
results showing the feasibility and usefulness of our
proposed model. There still remains much research
to be investigated other than association rule mining
and inter-transaction mining. Currently we are
working on efficient algorithms for profit mining in
various trading models. Our future research also
includes solving the challenge of reducing the search
space and speeding up the mining process with
limited memory space.
ACKNOWLEDGEMENTS
This research was supported by the National Science
Council, Taiwan, under grant NSC 98-2221-E-035-
059-MY2.
REFERENCES
Agrawal, R., Imielinski, T., and Swami, A. 1993. Mining
association rules between sets of items in large
databases, In Proceedings of ACM SIGMOD, pp. 207–
216.
Boetticher, G.D., 2006. Teaching Financial Data Mining
using Stocks and Futures Contracts, Journal of
Systemics, Cybernetics and Informatics, Vol. 3, No. 3,
pp. 26-32.
Lu, H., Han, J. and Feng, L., 1998. Stock Movement and
n-Dimensional Intertransaction Association Rules, In
Proceedings of 1998 SIGMOD Workshop, Research
Issues on Data Mining and Knowledge Discovery, Vol.
12, pp. 1-7.
Magdon-Ismail, M. and Atiya, A., 2004. Maximum
drawdown, Risk, Vol. 17, No. 10, pp. 99-102.
Shen, L., Shen, H., and Cheng, L., 1999. New algorithms
for efficient mining of association rules, Information
Sciences, 118 (1–4), pp. 251–268.
Yao, H. and Hamilton H. J., 2006. Mining itemset utilities
from transaction databases, Data & Knowledge
Engineering, Vol. 59, pp. 603 – 626
Zhang D. and Zhaou L., 2004. Discovering golden
nuggets: data mining in financial application, IEEE
transactions on Systems, Man, and Cybernetics – Part
C: Application and Review, Vol. 34(4), pp. 513-522.
Trade Station, 2011. TradeStation Securities, Inc. for
simulated trading. http://www.tradestation.com/
default_2.shtm
KDIR 2011 - International Conference on Knowledge Discovery and Information Retrieval
514