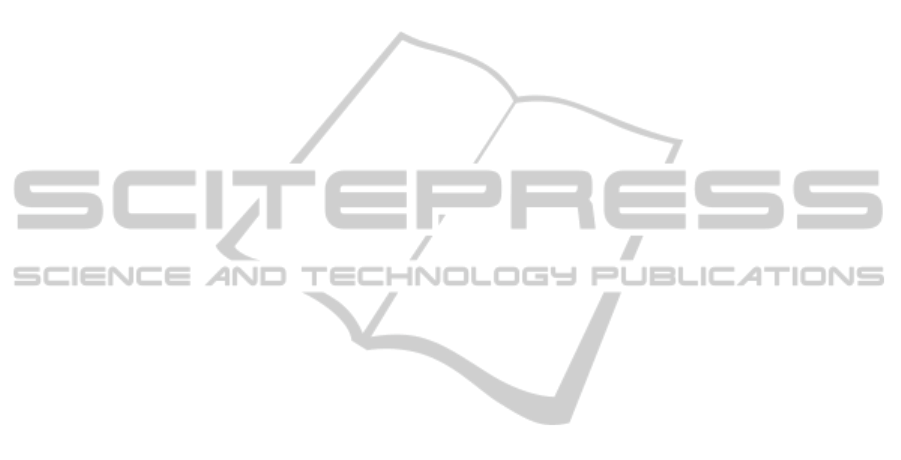
the single appearance of any sub-string in positive or
negative set to eliminate the data set bias.
The table demonstrates that new method can
outperform miRTif in all evaluation criteria except
specificity, where they perform equally. The
superiority of present method is quite significant in
terms of ROC statistics. This shows that new method
is not only successful in separation of positive and
negative examples but also able to successfully
quantize the level of certainty in its prediction. This
result is consistent with our intention to release this
model; enabling the sequence to be easily integrated
with other data.
4 CONCLUSIONS
We have introduced a probabilistic method to model
miRNA-target binding and evaluated its
performance on prediction of the interaction when
miRNA sequence and a putative binding site are
given. The accurate results that we obtained from the
experiments suggest that present model is able to
capture compositional properties of a duplex
sequence by additionally considering the effect of
different base pairings, mismatches and gaps with
their arrangements inside the duplex.
The model which we proposed may find
applications in several platforms. First, it can be
used as a post-processing filter for other miRNA
target prediction tools. Many of available algorithms
consider the seed match as a strong evidence for
target identification. Since it is possible to observe
random mRNA matches to seed region without any
interaction, this decision criterion can mislead the
algorithm to produce excessive number of false
positives. Present method is able to reject miRNA-
nontarget duplexes despite a high seed
complementarity, thus it may help to reduce the
number of false positives by reanalysing the binding
site predicted by former tool. Second, it may serve
complementary information which can be deployed
in target prediction algorithms. Conventional
methods perform a window-based linear scan over
the mRNA sequence to identify a putative binding
site which may attain a large binding score based on
a weighted sum of predefined criteria. Output of
proposed model is an obvious complement to other
determinants such as structure, site accessibility or
cross-spices conservation in this scoring scheme.
Third, the model enables the researchers to integrate
sequence data directly with other behavioural data
such as gene expression profiles over a probabilistic
framework. An integrated framework can provide a
comprehensive analysis of miRNA functions
associated with other entities, conditions or diseases.
Machine learning research has been competing in
two directions for intelligent analysis of
heterogeneous data: black-box kernel methods such
as Support Vector Machines and probabilistic
graphical models such as Bayesian Networks. Latter
requires a probabilistic representation of each
contributor in the model. Present scheme can fill a
gap in this respect.
It is anticipated that the participation of
computational models into miRNA research will
increasingly continue in coming years. We believe
that integration of multi-source heterogeneous data
will be a focal point in this research. Our study does
not yield a standalone tool in this context; however,
it provides a different view of miRNA-target
interactions from which future research can
definitely benefit. As a future work, we plan to
analyze the effects of seed and non-seed regions and
the types of different pairings of match and
mismatches in duplex analysis. Our final goal is to
come up with an integrative solution which
combines this sequence-based model with other
behavioural data in order to find functional maps of
miRNAs and their targets.
ACKNOWLEDGEMENTS
This study was supported by the Scientific and
Technological Research Council of Turkey
(TUBITAK) under the Project 110E160.
REFERENCES
Alexiou P., Maragkakis M., Papadopoulos G. L. et al.,
Lost in translation: an assessment and perspective for
computational microRNA target identification.
Bioinformatics 2009; 25:3049-3055.
Barbato C, Arisi I, Frizzo M. E. et al. Computational
Challenges in miRNATarget Predictions: To be or Not
to be a True Target? J Biomed Biotechnol 2009;
2009:803069.
Bartel DP. MicroRNAs: target recognition and regulatory
functions. Cell 2009; 136: 215-233.
Bartel D. P., MicroRNAs: genomics, biogenesis,
mechanism, and function. Cell 2004;116:281-297
Begleiter R., El-Yaniv R., Yona G., On prediction using
variable order Markov models, Journal of Artificial
Intelligence Research 2004, 22:385-421.
Bejerano G., Yona G., 2001. Variations on probabilistic
suffix trees: statistical modeling and prediction of
protein families. Bioinformatics 17, 23-43.
NCTA 2011 - International Conference on Neural Computation Theory and Applications
292