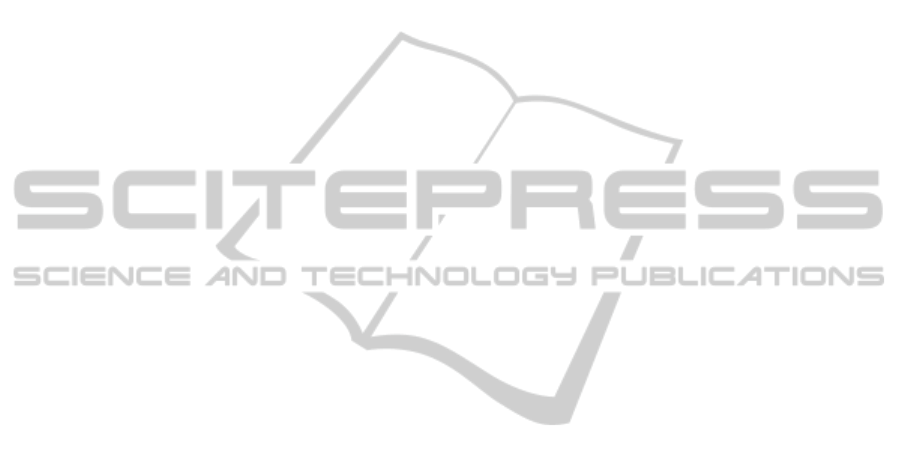
A short overview about the most innovative
solutions for each one of the key technologic aspects
featuring cognitive networks will be provided as
well as a short analysis about related business
models. Finally, in the last section, the impact of the
semantic technologies application is analyzed, as
key factor for the improving of the interoperability
level among heterogeneous networks, sub-networks
and data. Furthermore, the capabilities of knowledge
building and intelligent analysis of data can be
strongly improved.
2 COGNITIVE NETWORKS:
FROM SCIENCE TO REALITY
In this section, first the semantic ecosystems will be
defined and characterized both with their
relationships with real environments. Later, the
current approaches to concrete solutions and related
limitations are analyzed. Therefore, the main section
scope is the definition of a generic reference
scenario for semantic ecosystems in relation with
their technologic and economic sustainability. As it
will be discussed, there is, at the moment, a
significant gap between theoretical models and their
concrete application.
2.1 Semantic Ecosystems among
heterogeneous Cognitive Networks
In the context of this work, a metropolitan (or urban
(Wikipedia, Urban Ecosystem) ecosystem (Figure 1)
is defined as a large scale ecosystem composed of
the environment, humans and other living
organisms, and any structure/infrastructure or object
physically located in the reference area.
An exhaustive analysis of environmental and
social phenomena is out of paper scope. Just
considering that we are living in an increasingly
urbanized world. From recent studies, it appears that
this tendency will be probably followed also in the
next future. It is a commonly accepted assumption
that further increases in size and rates of growth of
cities will no doubt stress already impacted
environments as well as the social aspect of the
problem.
Considering this tendency is hard to be
controlled or modified, there are a great number of
interdisciplinary initiatives, studies and researches
aimed to understand the current impact of the
phenomena as well as to foresee the evolution of it.
These studies have, evidently, a scientific focus,
but they also could be of interest in the context of
the everyday life. In fact, modern cities change their
structure and physiology in function of human
activities that constantly act as inputs for the
feedback system. It is easy to imagine the great
number of services that could improve the quality of
life of citizens (or collectives) with a deep
knowledge of the environment.
As mentioned, the study of the human activities,
of the environmental and climatic phenomena is
object of interest in the context of several disciplines
and applications. All these studies are normally
independent initiatives, logically separated
researches and, in the majority of the cases, results
are hard to be directly related. This could appear a
paradox: interest phenomena happen in the same
physical ecosystem, involving the same actors but
the definition of the dependencies/relationships
among atomic results are omitted even if they are
probably the most relevant results.
The common point is the need of great amounts
of heterogeneous data, normally generated on large
scale (Akyildiz, 2002). They can be “simple”
measurements or complex phenomena, sometimes
hard to be detected. This overall approach according
heterogeneous model has a strong impact especially
in the representation and processing of the
information.
Summarizing, at now urban ecosystems have a
directly equivalent logic concept by a knowledge
perspective but its realizations are mainly
knowledge environments than effective knowledge
ecosystems.
2.2 Current Approaches and
Limitations
The normal technologic support for enabling
knowledge environment is the cognitive network
(Thomas, 2005) that assumes a physical
infrastructure (sensors) able to detect interest
information or phenomena and a logic infrastructure
able to process the sensor data (knowledge building)
eventually performing actions, responses or complex
analysis.
The parameters that can potentially affect the
“quality” of the applications or studies are mainly
the sensor technology (constantly increasing in
terms of reliability, precision and capabilities), the
coverage area, the amount of data and, finally, the
process capabilities.
Current solutions are hard to be proposed on
large scale due to the current limitations of the
KEOD 2011 - International Conference on Knowledge Engineering and Ontology Development
488