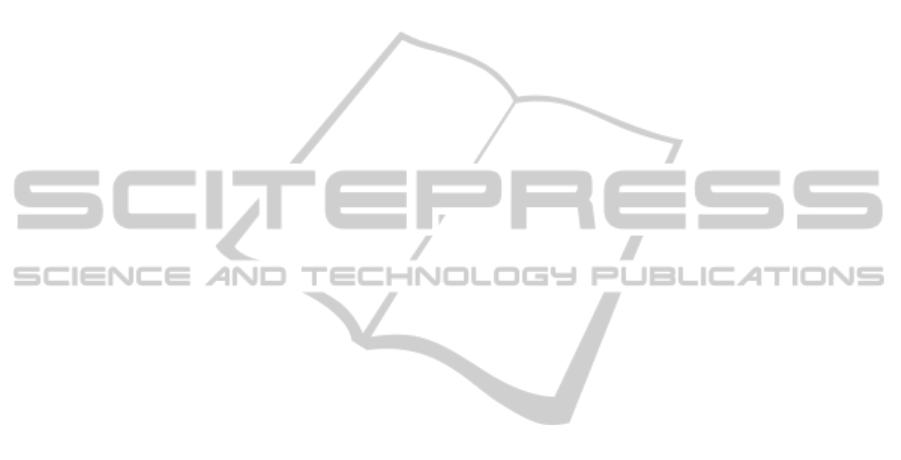
ws the association among the elements mentioned be-
fore, and the further identification of any information
resource if it is required. As a result, representation
of a chemical flexible process has been developed and
distributed inside an ontology.
The ANSI/ISA-88 defines a physical model
(equipment) and a procedural model (tasks). Both the
procedural and physical model are related each other
by means of the recipes: a recipe consists of the set of
information that uniquely defines the production re-
quirementsfor a specific product. The standard differ-
entiates between four types of recipes: general, site,
master and control. At planning and control level, the
information arrives detailed disposed in master and
control recipes. However when the strategic level is
to be modeled, the general and site recipes gather the
information related to this decision level.
The general recipe is handled at the company level
as the building block for lower-level recipes. It con-
tains the information about the required raw materi-
als, their quantities and processing stages for making
the product. Such recipe is further specified for the
manufacturing sites by the site recipes, which con-
tain the conditions and constraints related to the pro-
duction site for determining its scheduling. Master
recipes are derived from site recipes and are targeted
at the process cell including the following information
categories: header, formula, equipment requirements
and procedure. Control recipes are batches that are
created from master recipes. Specifically, they con-
tain the product-specific process information that is
required to manufacture a particular batch of product.
Regarding the strategic level, the supply chain
management decisions are related to the facility lo-
cation, production capacity and resources allocation,
distribution flows and inventory policies. Therefore,
the flow of materials, information and economic re-
sources along the wide enterprise structure are mod-
eled, as well as the restrictions regarding mass bal-
ances, capacity and technological constraints, such
as product recipes, product sequencing, unstable and
perishable materials, economic limitations, suppliers’
capacity and market demand among others.
3.2 Methodology
Various methodologies exist to guide the theoreti-
cal approach to the design of ontologies, and numer-
ous building tools are available. However, there is a
lack of consensus on a uniform approach to design-
ing and maintaining these ontologies. The method-
ology adopted in this paper is based on two on-
tology development methodologies "Methontology"
(López et al., 1999) and "On-To-Knowledge" (Sure
and Studer, 2002). On the one hand, by the use of
"Methontology", a support for the entire life-cycle
of ontology development is provided. On the other
hand, the analysis of usage scenarios of the "On-to-
Knowledge" methodology, allows to present knowl-
edge efficiently and effectively.
The phases that involve the characteristics of the
aforementioned methodologies are grouped inside the
PDCA cycle (Figure 2). Using the PDCA (Plan,
Do(study), Check and Act) cycle allows to coordi-
nate the continuous improvement efforts of the two
methodologies. As a result of the cycle, a good plan-
ning and effective actions come out. Moreover, the
base of quality management creates an easy manner
for improving the developed methodology about on-
tologies.
Plan Phase. This stage tries to make an arrangement
by first capturing the requirements and specifi-
cations, and next adequately documenting them.
The description of general information (e.g., date,
creators, and versions) is detailed. Besides the on-
tology motivations, the uses and applicability and
potential users are also described. The possible
knowledge sources are defined as well. Owing to
its expressive, declarative, portable, domain inde-
pendent and semantically definition, the language
used in the ontological approach is OWL (ontol-
ogy web language). One of the main benefits of
OWL is the support for automated reasoning, and
to this effect, it has a formal semantics based on
Description Logics (DL). The decidability, which
refers to the existence of an effective method for
determining membership in a set of formulas (the-
orems), of the logic ensures that sound and com-
plete DL reasoners can be built to check the con-
sistency of an OWL ontology. Furthermore, rea-
soners can be used to derive inferences from the
asserted information, e.g., infer whether a partic-
ular concept in an ontology is a subconcept of an-
other.
Do Phase. In this stage the principal components
of the conceptualization model are established.
Glossary of terms, concepts and properties, hier-
archies, the taxonomy, class and instant attributes
among others are described. Then a formaliza-
tion of all the content should be made in order
to agree with the knowledge sources. An iden-
tification of other ontologies that probably could
be reused is performed in order to determine if
they could be added to the model. In this stage the
translation of the model to an ontology language
must be done. The OWL ontology editors used
for the development of this model were Protégé
(Horridge et al., 2007) as main editor and Swoop
ONTOLOGICAL FRAMEWORK FOR THE ENTERPRISE FROM A PROCESS PERSPECTIVE - Operational, Tactical
and Strategic Integration for Improved Decision-making
541