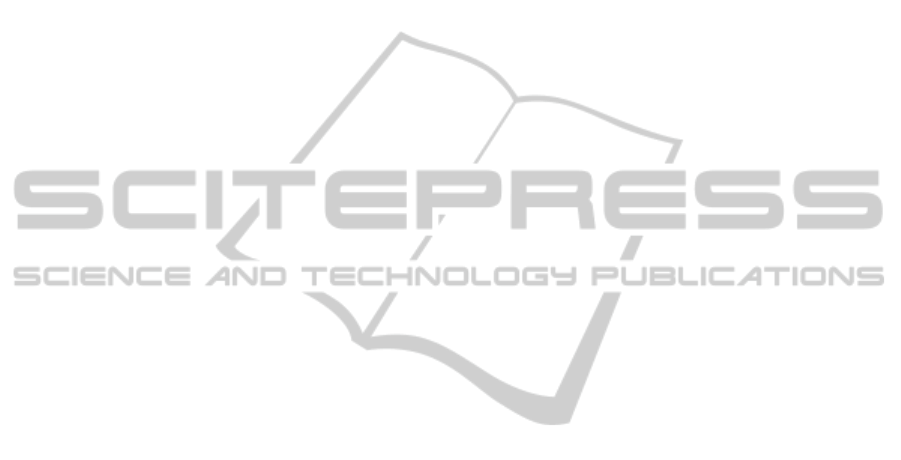
catenation feature ξ
5
to prove the effectiveness of the
concatenation method.
For comparison, SSVEP is classified with LDA
classification with only ξ
5
or only ξ
7
. There are two
kinds of stimulus frequencies as parameters (10 and
13.33 Hz). Fig. 7 shows learning error rate in those
cases. One can observe on this figure that error rates
with concatenation features are systematically lower
than those with averaged Fourier powers.
5 DISCUSSIONS
With the concatenation method, we can obtain not
only higher frequency resolution but also higher peak
of Fourier power at the stimulus frequency. There-
fore, it may work effectively in the situation where
high temporal resolution of SSVEP detection is re-
quired. This method only requires signals concatena-
tion, consequently, it is not computationally demand-
ing, and can be used in real time processing. It re-
duces the patients’ stress when using SSVEP-BCI.
The patients are not required to focus for more than
one second, as evidenced by the 0.86 classification
accuracy with 1 s epoch (Table 2).
In our future works, we intend to confirm effects
of concatenating points with artificial signals, and in
addition to EEG signals. Moreover, using a larger
number of EEG channels could allow us to go down
to even shorter EEG epochs. One of the unsolved
problems is that each EEG epoch has different phase
and amplitudes at their borders. Therefore, the cy-
cles in each epoch do not match perfectly, which most
probably reduces the SSVEP peaks in the Fourier
spectrum. Improved algorithms for windowing EEG
epochs, and for matching their phase differences, are
to be developed.
6 CONCLUSIONS
A concatenation method is proposed to improve the
frequency resolution of Fourier spectrum when using
short EEG epochs. It successfully detected SSVEP
response by concatenating EEG epochs in the time
domain, down to 1 s windows. Classification tests on
EEG data proved that concatenation method works
better than the averaging of Fourier spectrums com-
puted from the EEG epochs.
REFERENCES
Bashashati, A., Fatourechi, M., and Ward, R. K. (2007).
A survey of signal processing algorithms in brain-
computer interfaces based on electrical brain signals.
J. Neural Engineering, 4:R32–R57.
Bin, G., Gao, X., Yan, Z., Hong, B., and Gao, S. (2009). An
online multi-channel ssvep-based brain-computer in-
terface using a canonical correlation analysis method.
J. Neural Engineering, 6(4):046002.
Birbaumer, N. (2006). Breaking the silence: brain-
computer interfaces (bci) for communication and mo-
tor control. Psychophysiology, 43:517–532.
Dauwels, J., Vialatte, F.-B., Musha, T., and Cichocki, A.
(2010). A comparative study of synchrony measures
for the early diagnosis of alzheimer’s disease based on
eeg. NeuroImage, 49:668–693.
Dreyfus, G. (2005). Neural networks: methodology and
applications. Springer-Verlag.
Huang, N. E., Shen, Z., Long, S. R., Wu, M. C., Shih,
H. H., Zheng, Q., Yen, N.-C., Tung, C. C., and Liu,
H. H. (1998). The empirical mode decomposition and
hilbert spectrum from nonlinear and non-stationary
time series analysis. In R. Soc. Lond. A, volume 454,
pages 903–995.
Kawabata, N. (1973). A non-stationary analysis of the elec-
troencephalogram. IEEE Trans Biomed Eng, 20:444–
452.
Lotte, F., Cogedo, M., Lecuyer, A., Lamarche, F., and Ar-
naldi, B. (2007). A review of classification algorithms
for eeg-based brain-computer interfaces. J. Neural
Engineering, 4:R1–R13.
Nunez, P. L., Srinivasan, R., Westdorp, A. F., Wijesinghe,
R. S., Tucker, D. M., Silberstein, R. B., and Cadusch,
P. J. (1997). Eeg coherency: l: statistics, refer-
ence electrode, volume conduction, laplacians, cor-
tical imaging, and interpretation at multiple scales.
Electroencephalography and Clinical Neurophysiol-
ogy, 103:499–515.
Quiroga, R., Sakowitz, O., Bas¸ar, E., and Sch¨urmann, M.
(2001). Wavelet transform in the analysis of the fre-
quency composition of evoked potentials. Brain Re-
search Protocols, 8:16–24.
Sanei, S. and Chambers, J. A. (2007). EEG signal process-
ing. Wiley, Lodon.
Vialatte, F., Sol´e-Casals, J., and Cichocki, A. (2008). Eeg
windowed statistical wavelet scoring for evaluation
and discrimination of muscular artifacts. Physiolog-
ical Measurements, 29(12):1435–1452.
Vialatte, F.-B., Maurice, M., Dauwels, J., and Cichocki, A.
(2010). Steady-state visually evoked potentials: fo-
cus on essential paradigms and future perspectives.
Progress in Neurobiology, 90:418–438.
CONCATENATION METHOD FOR HIGH-TEMPORAL RESOLUTION SSVEP-BCI
451