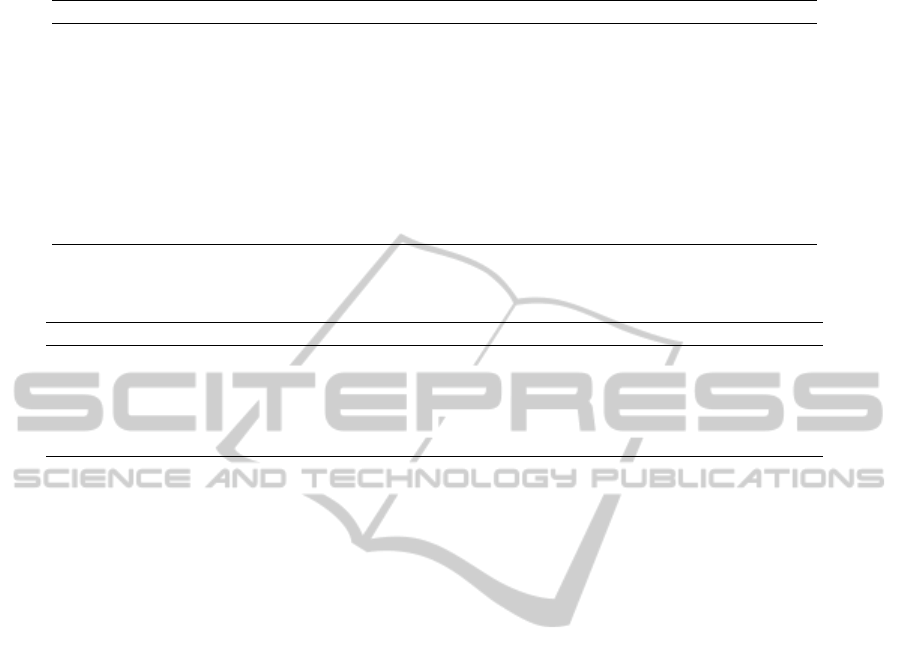
Table 5: Average success percentage by 5 users on each task using 3 mental tasks.
Tasks Rest Arm Song Math Object
Rest – Arm – Song 75.02 64.48 60.83 - -
Rest – Arm – Math 76.76 64.70 - 67.86 -
Rest – Arm – Object 80.44 62.28 - - 63.67
Rest – Song – Math 76.19 - 58.60 66.54 -
Rest – Song – Object 77.09 - 60.07 - 66.44
Rest – Math – Object 77.48 - - 68.53 67.35
Arm – Song – Math - 67.66 73.71 68.93 -
Arm –Song – Object - 65.69 77.35 - 65.09
Arm – Math – Object - 67.04 - 76.39 68.49
Song – Math – Object - - 73.86 70.05 66.86
Average 77.16 65.31 67.40 69.72 66.32
Table 6: Average success percentage by 5 users on each task using 4 mental tasks.
Tasks Rest Arm Song Math Object
Rest – Arm – Song – Math 71.83 60.10 53.46 62.02 -
Rest – Arm – Song – Object 73.03 58.24 54.28 - 57.70
Rest – Arm – Math – Object 73.24 58.27 - 63.01 58.31
Rest – Song – Math – Object 71.01 - 53.81 62.13 61.70
Arm – Song – Math – Object - 59.54 69.10 63.92 59.31
Average 72.28 59.04 57.66 62.77 59.26
indicates that the Emotiv Epoc is suitable to be used
in a Brain-Computer Interface. As a future work, the
implementation of an online application has been
proposed. Also, is expected to perform different tests
using volunteers with disabilities. In order to
compare results and verify if there is any
performance loss due to the Emotiv Epoc device, it
is expected to test the same experiments with a high-
quality research-oriented EEG system (gUSBamp
g.tec). Finally, the use of a new set of tasks (for
example, new motor tasks, tongue movement,
mental calculation or word formation) are suggested,
as well as the use of new classification algorithms.
ACKNOWLEDGEMENTS
This work has been supported by the Ministerio de
Ciencia e Innovación of the Spanish Government
through project DPI2008-06875-C03-03 and by the
Consellería d’Educació de la Generalitat Valenciana
through grant BEST/2010/047, grant
ACOMP/2011/066 and grant FPA/2011/058.
REFERENCES
American Electroencephalographic Society. (1991).
American Electroencephalographic Society Guidelines
for Standard Electrode Position Nomenclature.
Journal of Clinical Neurophysiology 8(2)
pp. 200-202.
AutoNOMOS project Freie Universität Berlin. (2011).
Brain Driver. [online] Available at: <http://
autonomos.inf.fu-berlin.de/news/brain-driver>
[Accessed 10 May 2011]
Babiloni, F., Cincotti, F., Bianchi, L., Pirri, G., Millán, J.
R., Mourio, J., Salinari, S. and Marciani, M.G. (2001).
Recognition of imagined hand movements with low
resolution surface laplacian and linear classifiers.
Medical Engineering Samp; Physics 23, pp. 323-328.
Bashashati, A., Fatourechi, M., Ward, R. K. and Birch, G.
E. (2007). A survey of signal processing algorithms in
brain–computer interfaces based on electrical brain
signals. Journal of Neural Engineering, 4(2), R32-57.
Bayliss, J. D. (2003). Use of the evoked potential P3
component for control in a virtual environment. IEEE
Transactions on Neural Systems and Rehabilitation
Engineering 11, pp. 113-116.
Campbell, A. T., Choudhury, T., Hu, S., Lu, H., Mukerjee,
M. K., Rabbi, M. and Raizada, R. D. S. (2010).
NeuroPhone: Brain-Mobile Phone Interface using a
Wireless EEG Headset. [online] Available at
<http://sensorlab.cs.dartmouth.edu/publications.html>
[Accessed 15 May 2011]
Carmena, J. M., Lebedev, M. A., Crist, R. E., O’Doherty,
J. E., Santucci, D. M., Dimitrov, D. F., Patil, P. G,
Henriquez, C. S. and Nicolelis, M. A. L. (2003).
Learning to control a brain-machine interface for
reaching and grasping by primates. PloS Biology 1, pp.
193-208.
Chang, C.-C. and Lin, C.-J. (2001). LIBSVM: A Library
for Support Vector Machines. [online] (Updated April
NCTA 2011 - International Conference on Neural Computation Theory and Applications
420