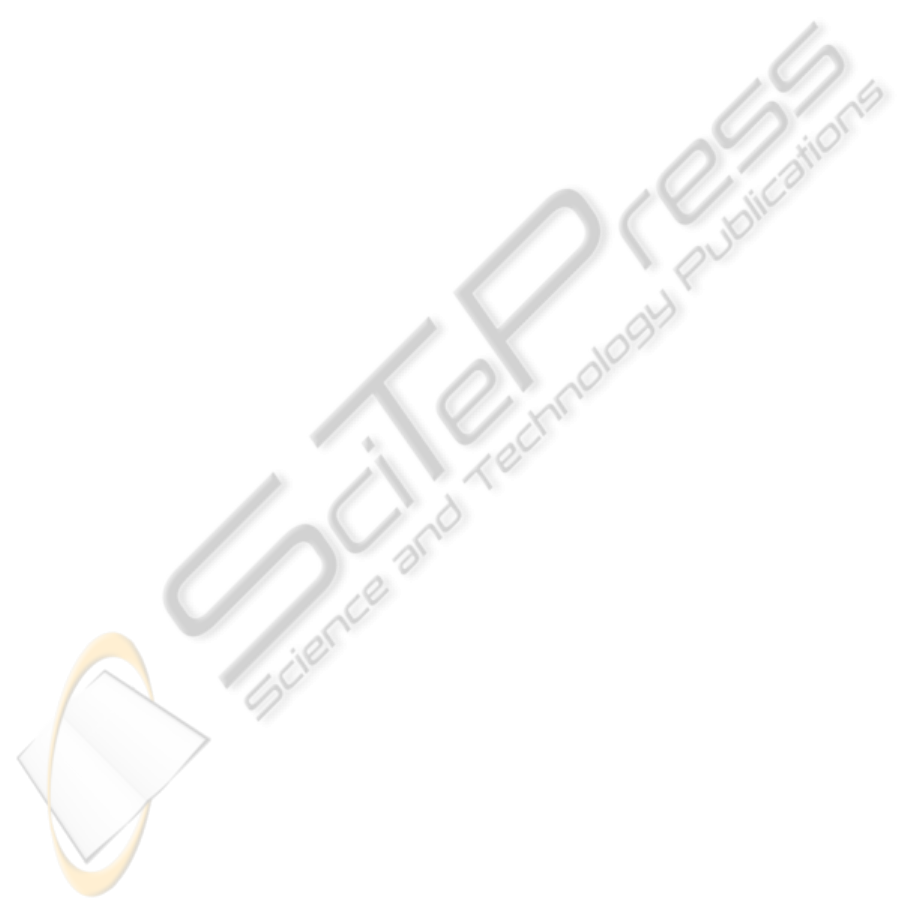
such an order does improve action recognition. On
the other hand, the significantly better performance
obtained by explicitly modeling human silhouette dy-
namics through HOF and HOG show that although
STIP-based representations are efficient, they may
fail to detect some feature points that are relevant for
recognition.
In the future, we are targeting the task of action
recognition in the context of daily human activities.
Here, the problem becomes more difficult as the in-
put will usually consist of a long video sequence
made up of a continuous sequence of actions (for in-
stance ”walk”, ”eat”, ”watching TV” and then ”laying
down”). Therefore, the purpose is to conjointly seg-
ment and recognize actions. One of the goals, in the
context of this application and according to the results
obtained in this study, is to select in an automatic way
the type of features (STIPs or HOG/HOF) to be ex-
tracted from the silhouette depending on such factors
as the complexity of the background, occlusion and
the presence or not of several moving shapes.
REFERENCES
Abdelkader, M. F., Roy-Chowdhury, A. K., Chellappa, R.,
and Akdemir, U. (2008). Activity representation using
3d shape models. J. Image Video Process., 2008:5:1–
5:16.
Atine, J.-C. (2004). People action recognition in image se-
quences using a 3d articulated object. In ICIAR (1),
pages 769–777.
Bay, H., Tuytelaars, T., and Gool, L. J. V. (2006). Surf:
Speeded up robust features. In ECCV (1)’06, pages
404–417.
Blank, M., Gorelick, L., Shechtman, E., Irani, M., and
Basri, R. (2005). Actions as space-time shapes. In
The Tenth IEEE International Conference on Com-
puter Vision (ICCV’05), pages 1395–1402.
Bobick, A. F. and Davis, J. W. (2001). The recognition
of human movement using temporal templates. IEEE
Trans. Pattern Anal. Mach. Intell., 23:257–267.
Dalal, N. and Triggs, B. (2005). Histograms of oriented
gradients for human detection. In Proceedings of the
2005 IEEE Computer Society Conference on Com-
puter Vision and Pattern Recognition (CVPR’05) -
Volume 1 - Volume 01, CVPR ’05, pages 886–893,
Washington, DC, USA. IEEE Computer Society.
Doll
´
ar, P. (2007). Piotr’s Image and Video Matlab Toolbox
(PMT).
Dollar, P., Rabaud, V., Cottrell, G., and Belongie, S. (2005).
Behavior recognition via sparse spatio-temporal fea-
tures. In Proceedings of the 14th International Con-
ference on Computer Communications and Networks,
pages 65–72, Washington, DC, USA. IEEE Computer
Society.
Elgammal, A. M., Harwood, D., and Davis, L. S. (2000).
Non-parametric model for background subtraction. In
Proceedings of the 6th European Conference on Com-
puter Vision-Part II, ECCV ’00, pages 751–767, Lon-
don, UK. Springer-Verlag.
Gorelick, L., Blank, M., Shechtman, E., Irani, M., and
Basri, R. (2007). Actions as space-time shapes. Trans-
actions on Pattern Analysis and Machine Intelligence,
29(12):2247–2253.
Harris, C. and Stephens, M. (1988). A Combined Corner
and Edge Detection. In Proceedings of The Fourth
Alvey Vision Conference, pages 147–151.
Huang, F. and Xu, G. (2007). Viewpoint insensitive action
recognition using envelop shape. In Proceedings of
the 8th Asian conference on Computer vision - Vol-
ume Part II, ACCV’07, pages 477–486, Berlin, Hei-
delberg. Springer-Verlag.
˙
Ikizler, N. and Duygulu, P. (2007). Human action recogni-
tion using distribution of oriented rectangular patches.
In Proceedings of the 2nd conference on Human mo-
tion: understanding, modeling, capture and anima-
tion, pages 271–284, Berlin, Heidelberg. Springer-
Verlag.
Kl
¨
aser, A. (2010). Learning human actions in video. PhD
thesis, Universit
´
e de Grenoble.
Kl
¨
aser, A., Marszałek, M., and Schmid, C. (2008). A spatio-
temporal descriptor based on 3d-gradients. In British
Machine Vision Conference, pages 995–1004.
Laptev, I. and Lindeberg, T. (2003). Space-time interest
points. In Proceedings of the Ninth IEEE Interna-
tional Conference on Computer Vision - Volume 2,
ICCV ’03, pages 432–, Washington, DC, USA. IEEE
Computer Society.
Laptev, I., Marszałek, M., Schmid, C., and Rozenfeld,
B. (2008). Learning realistic human actions from
movies. In Conference on Computer Vision & Pattern
Recognition.
Lindeberg, T. (1998). Feature detection with automatic
scale selection. Int. J. Comput. Vision, 30:79–116.
Lucas, B. D. and Kanade, T. (1981). An iterative image
registration technique with an application to stereo vi-
sion. In Proceedings of the 7th international joint
conference on Artificial intelligence - Volume 2, pages
674–679, San Francisco, CA, USA. Morgan Kauf-
mann Publishers Inc.
Moon, H. and Chellappa, R. (2008). 3d shape-encoded
particle filter for object tracking and its application
to human body tracking. J. Image Video Process.,
2008:12:1–12:16.
Niebles, J. C., Wang, H., and Fei-Fei, L. (2008). Un-
supervised learning of human action categories us-
ing spatial-temporal words. Int. J. Comput. Vision,
79:299–318.
Pehlivan, S. and Duygulu, P. (2009). 3d human pose search
using oriented cylinders. In S3DV09, pages 16–22.
Pnevmatikakis, A. and Polymenakos, L. (2007). 2d per-
son tracking using kalman filtering and adaptive back-
ground learning in a feedback loop. In Proceed-
ings of the 1st international evaluation conference
HUMAN ACTION RECOGNITION USING CONTINUOUS HMMS AND HOG/HOF SILHOUETTE
REPRESENTATION
507