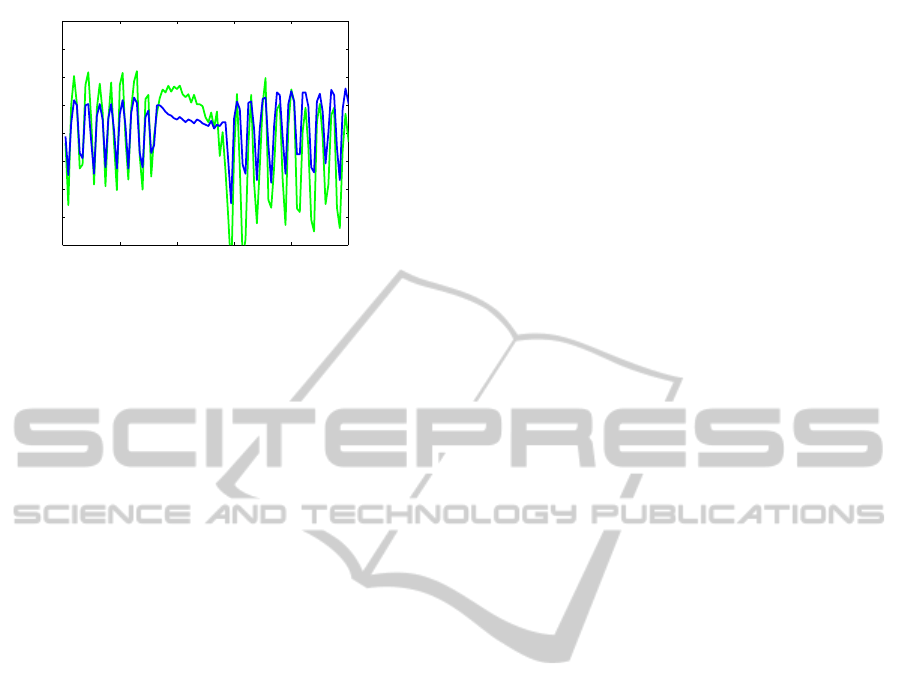
0 20 40 60 80 100
−2
−1.5
−1
−0.5
0
0.5
1
1.5
2
time [s]
amplitude [a.u.]
Figure 3: The graph shows the respiratory signal (blue) and
the EDR signal (green) for a subset of a timeseries contain-
ing periods of deep breathing and periods with bated respi-
ration.
demonstrate the robustness of this EDR approach the
study must be extended to a cohort with different gen-
der, age, etc. in different physical situations. Second,
the signal recording devices were chosen because of
availability rather than optimum superior signal qual-
ity. It is anticipated that better results could be ob-
tained by using an ECG device with a higher sampling
rate and full control of the amplification and filter-
ing of the signal. In particular, the asymmetry of the
T wave is suspiciously absent on our data generated
with the Zephyr Bioharness. Likewise, no informa-
tion from the P wave has been included.
Similarly, a more precise respiration sensor with
higher sampling rate and larger immunity to move-
ment artifacts would provide higher quality data to
feed into the evolutionary algorithm.
The goal of this work has been to investigate the
feasibility of optimizing EDR with an evolutionary
algorithm and provide a proof of concept. More re-
search remains to be done, but we have found that it is
indeed possible to optimize EDR with an evolutionary
algorithm and we believe that evolutionary algorithms
will provide a fruitful tool for further studies in EDR
and other biomedical signal processing problems.
ACKNOWLEDGEMENTS
This work is a part of the MIKAT project funded
by Region Midtjylland and the European Union via
Caretech Innovation.
REFERENCES
Clifford, G. D., Azuaje, F., and McSharry, P. E., editors
(2006a). Advanced methods and tools for ECG data
analysis. Artech House Publishing.
Clifford, G. D., Azuaje, F., and McSharry, P. E., edi-
tors (2006b). Advanced methods and tools for ECG
data analysis. Artech House Publishing, accompa-
nying website. Retrieved june 23, 2011 from http://
www.ecgtools.org.
Dobrev, D. and Daskolov, I. (1998). Two-electrode telemet-
ric instrument for infant heart rate and apnea monitor-
ing. Medical Engineering & Physics, 20:729–734.
Grossman, P. and Wientjes, K. (1986). Respiratory sinus
arrhytmia and parasympathetic cardiac control: some
basic issues concerning quantification, applications
and implications. In Grossman, P., Jansenn, K., and
Waitl, D., editors, Cardiorespiratory and cardioso-
matic psycophysiology, pages 117–138. Plenum Press,
NY.
Koza, J. R. (1992). Genetic Programming: On the Pro-
gramming of Computers by Means of Natural Selec-
tion. MIT Press, Cambridge, MA, USA.
MATLAB (2010). version 7.11.0.584 (R2010b). The Math-
Works Inc., Natick, Massachusetts.
Mazzanti, B., Lamberti, C., and de Bie, J. (2003). Val-
idation of an ECG-derived respiration monitoring
method. Computers in Cardiology, 30:613–616.
Moody, G., Mark, R., Zoccola, A., and Mantero, S.
(1985). Derivation of respiratory signals from multi-
lead ECGs. Computers in Cardiology, 12:113–116.
O’Brien, C. and Heneghan, C. (2007). A comparison of
algorithms for estimation of a respiratory signal from
the surface electrocardiogram. Computers in Biology
and Medicine, 37:305–314.
Raymond, B., Cayton, R. M., Bates, R. A., and Chappell,
M. J. (2000). Screening for obstructive sleep apnoea
based on the electrocardiogram - the computers in car-
diology challenge. Computers in Cardiology, 27:267–
270.
Schmidt, M. and Lipson, H. (2009). Distilling free-
form natural laws from experimental data. Science,
324(5923):81–85.
Sobron, A., Romero, I., and Lopetegi, T. (2010). Evaluation
of methods for estimation of respiratory frequency
from the ECG. Computers in Cardiology, 37:513–
516.
Zephyr Bioharness (n.d.). Retrieved june 23, 2011, from.
http://www.zephyr-technology.com.
BIOSIGNALS 2012 - International Conference on Bio-inspired Systems and Signal Processing
152