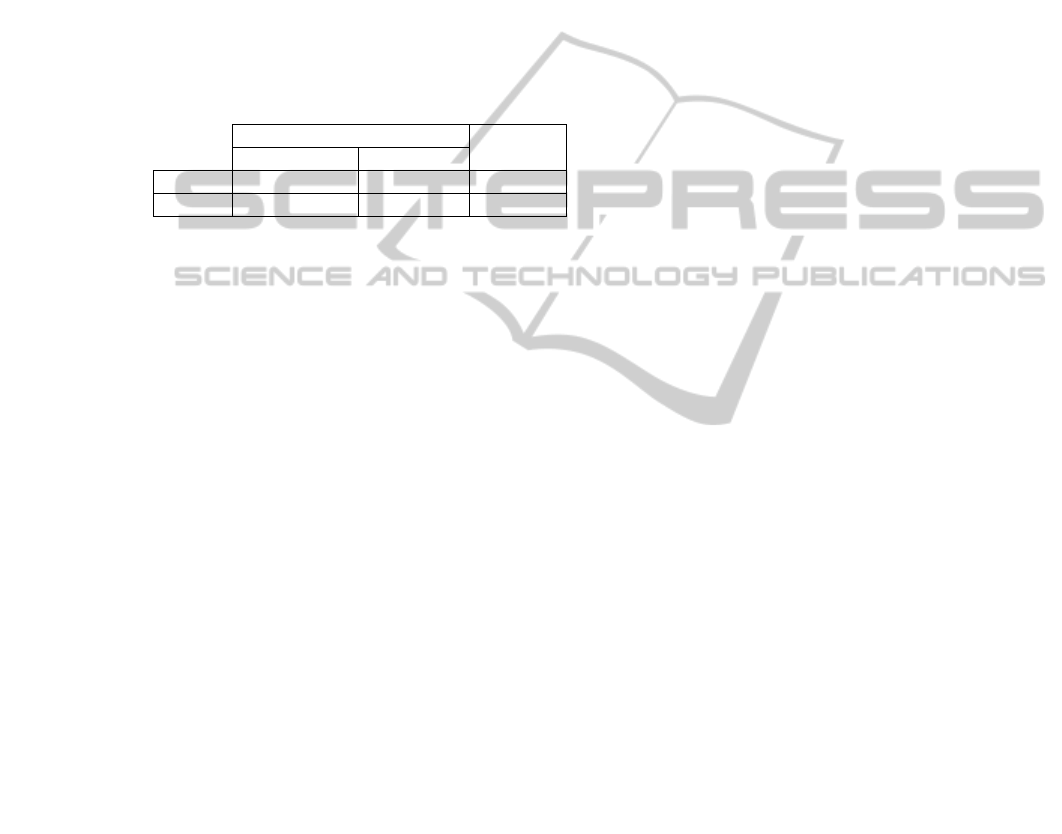
accuracy while the ANN classifier only exhibit
around 30% to 60% accuracy. This is due to the fact
that the fuzzy classifier has rules that can easily
distinguish between states. As for ANN classifier, it
needs to build the algorithm based on the training
data. This means that if the training data is lacking in
some aspects, then the accuracy of study data will
also be affected. In this project, the study data size is
small thus producing vulgar and incomplete patterns.
This affects the accuracy of the result due to the
difficulty of the algorithm to recognize the pattern
from the data. This can be solved by introducing
larger training data set.
Table 1: Classification accuracy.
FL
ANN
without Solver with solver
FCU 83.0% 97.8% 62.0%
ECR 82.9% 97.1% 37.1%
In term of automation, ease of use and capability
to adapt to various samples, the ANN classifier is a
better choice than fuzzy classifier. This is due to the
fact that the user only needs to introduce the input
and the target and the ANN will automatically create
an algorithm and network to recognize the study
data. On top of that, an accurate network that was
produce by ANN can be used on various samples
due to its learning capability. As for fuzzy classifier,
the user need to determine by them the membership
function, rules and need to calculate the accuracy
manually thus consuming a lot of time. However, it
is not a drawback to FL as automatic tuning is
possible (G. Panoutsos, 2010). The main advantage
of FL is the data doesn’t need to be trained like
ANN.
4 CONCLUSIONS
The study is to compare two classification methods
namely FL and ANN to determine the final output
from the extracted SEMG signals of the forearm.
The signals were recorded from FCU and ECR
during wrist flexion and extension respectively.
From the classification accuracy, it shows that
FL gave higher accuracy (>80%) compared to ANN
classifier (60%). This is due to small data size that
leads to the difficulty of the ANN model
development to recognize the pattern. The
performance can be improved by introducing larger
training data set.
REFERENCES
Ajiboye, A. W., R. (2005). "A Heuristic Fuzzy Logic
Approach to EMG Pattern recognition for
Multifunction Prosthesis Control." IEEE Transactions
on Biomedical Engineering 52(11): 280-291.
Chan, F. Y.-S., Y., Lam, F.; Yuan-Ting, Z; Parker, P.
(2000). "Fuzzy EMG Classification for Prosthesis
Control." IEEE Transactions on Rehabilittaion
Engineering 8(3).
Chappell, S. A. A. a. P. H. (2009). "Surface EMG Pattern
Analysis of the Wrist Muscles at Different Speeds of
Contraction." Journal of Medical Engineering and
Technology 33( 5,): 376-385.
Fukuda, O., and Tsuji, T. (2004). Control of an Externally
Powered Prosthetic Forearm Using Raw-EMG
Signals. SICE.
G. Panoutsos, M. M. (2010). "Neural-Fuzzy Modelling
Framework based on Granular Computing: Concepts
and Application." Fuzzy Sets and system 161(21):
2802-2830.
Hardeep S. Ryait; Arora, A. S. R. A. (2010).
"Interpretations of Wrist/grip Operations From SEMG
Signals at Different Locations on Arm." IEEE
Transactions on Biomedical Circuits and Systems
4(2): 101 - 111.
Hermans, H. F., B.; Merletti, R.; Stegeman, D.; Blok, J.;
Rau, G.; Disselhorst-Klug, C.; Hagg, G. (1999).
SENIAM 8, European Recommendations for Surface
Electromyography, results of the SENIAM Project,
Rossingh Research and Development.
Huang, H., and Chen, C. (1999). Development of a
Myoelectric Discrimination System for a Multi Degree
Prosthetic Hand. IEEE International Conference on
Robotics & Automation, Detroit, Michigan.
Hudgins, B. P., P.; Scott, R. N. (1999). "A New Strategy
for Multifunction Myoelectric Control." IEEE
Transactions on Biomedical Engineering 40(1): 82-94.
Kumar, V. P. S. a. D. K. (2008). Classification of Low-
level Finger Contraction from Single Channel Surface
EMG. 30th Annual International IEEE EMBS
Conference, Canada.
Mitchell, W. R. M. (2008). Development of a Clinically
Viable Multifunctional Hand Prosthesis. Myoelectric
Controls/Powered Prosthetics Sympossium, New
Brunswick, Canada.
Nagata, K., and Magatani, K. (2004). Development of the
Assist System to Operate a Computer for the Disabled
Using Multichannel Surface EMG. Proceedings of
26th Annual International conference of the IEEE
Engineering in Medicine and Biology Society.
Plettenburg, D. H. (2006). Upper Extremity Prosthetics,
Current Status & Evaluation, VSSD.
Tsuji, T. a. K., K. (2000). An EMG Controlled Pointing
Device Using a Neural Network. SICE.
Weir, R. A., A. (2003). A Multifunction Prosthesis
Controller Based on Fuzzy Logic Technique.
Proceedings of the 25th Annual International
Conference of the IEEE/EMBS.
BIOSIGNALS 2012 - International Conference on Bio-inspired Systems and Signal Processing
320