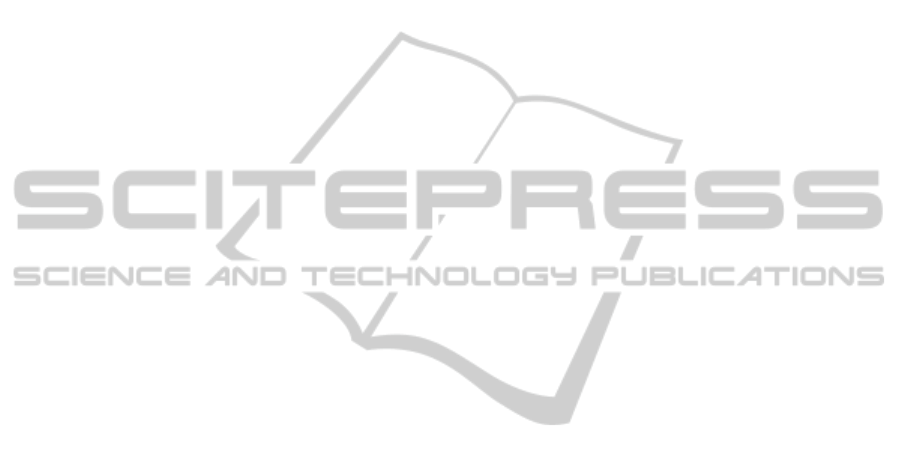
very high CPU time needed by the global research and
compared to the local research, comes with the size of
the task offers set used to find a solution.
Solution Quality. The quality of the solutions is
characterized by three different indicators: the total
postponement over agents initial schedule, the delay
of resolved freight deliveries and the additional travel
distance. Here we focus our study and observations
for a failure proportion varying from 1 to 30%. In-
deed, we consider that for any failure proportion su-
perior to 30% the simulation results concerns excep-
tional cases of disturbances in a transportation net-
work. Under this assumption and according to results
obtained, the mean total postponementinduced by ad-
ditional tasks in agents schedule is higher with the
global protocol than with the local protocol. In ad-
dition, the mean value of postponement is higher with
schedule type II than with type I, this observation rely
on the differences of road congestion characterizing
the two types of delivery schedules. Concerning the
mean postponement of resolved freight deliveries, we
observe higher mean postponement result values with
the global research procedure (from 17 to 27 min-
utes) than with the local research procedure(from 13
to 21 minutes). The mean additional travel distance
of agents obtained with the local research is higher
(from 1.5 km to 2,2 km) than with the global research
procedure (from 1,3 km to 1,5 km). In short, and with
regard to the global research, the local research max-
imizes the additional travel distances, but enables to
minimize the postponement over the delegated deliv-
eries and the agents task schedule.
5 CONCLUSIONS
In this paper, after the presentation of a task reallo-
cation problem and a state of the art, we proposed a
computational model that allows agents to delegate
the execution of tasks to others. This model aims to
capture all the the descriptors needed for task delega-
tion. It gives a description of the various constraints
for the performance of tasks under location and tem-
poral constraints by a set of situated agents. In or-
der to validate our model, we introduced a method
to enable the exploitation of residual resources in
a multi-agent system where agents have non share-
able resources to execute a defined task schedule. To
overcome disturbances during the execution of such
schedule, we propose to reallocate tasks to agents
over time periods where they can perform additional
tasks without modifying their initial schedule. Our
first experimental results show that the use of lo-
cal research is more efficient than global search and
gives solutions of equivalent quality, in term of addi-
tional distance and postpone delay. The main lines of
our future research will focus on optimizing the re-
search method by introducing a negotiation protocol.
We also aim to propose a task reallocation procedure
which enable the use of multiple task offers to solve
one failure. Another part of our work will concern
the influence of acceptance criteria on the method ef-
ficiency and the solution quality.
ACKNOWLEDGEMENTS
The present research work has been supported by the
Ile-de-France Region, the ”Minist`ere de l’
´
Economie,
de l’Industrie et de l’Emploi” and ADVANCITY.
REFERENCES
Barbucha, D. and Jedrzejowicz, P. (2009). Agent-based ap-
proach to the dynamic vehicle routing problem. In 7th
International Conference on PAAMS’09, pages 169–
178. Springer-Verlag.
Bellosta, M.-J., Kornman, S., and Vanderpooten, D. (2008).
A unified framework for multiple criteria auction
mechanisms. Web Intelligence and Agent Systems,
6(4):401–419.
Berbeglia, G., Cordeau, J.-F., and Laporte, G. (2010). Dy-
namic pickup and delivery problems. European Jour-
nal of Operational Research, 202(1):8–15.
Chevaleyre, Y., Dunne, P. E., Endriss, U., Lang, J.,
Lemaˆıtre, M., Maudet, N., Padget, J. A., Phelps, S.,
Rodr´ıguez-Aguilar, J. A., and Sousa, P. (2006). Is-
sues in multiagent resource allocation. Informatica,
30(1):3–31.
Cordeau, J.-F., Desaulniers, G., Desrosiers, J., Solomon,
M. M., and Soumis, F. (2001). VRP with Time Win-
dows, pages 157–193. Society for Industrial and Ap-
plied Mathematics, Philadelphia, PA, USA.
Davis, R. and Smith, R. G. (1983). Negotiation as a
metaphor for distributed problem solving. Artificial
Intelligence, 109:20–63.
Fischer, K., Chaib-draa, B., M¨uller, J. P., Pischel, M., and
Gerber, C. (1999). A simulation approach based on
negotiation and cooperation between agents: A case
study. IEEE Trans. on Systems, Man, and Cybernetics,
29:531–545.
Gorodetski, V. I., Karsaev, O., and Konushy, V. (2003).
Multi-agent system for resource allocation and
scheduling. In Mar´ık, V., M¨uller, J. P., and Pechoucek,
M., editors, CEEMAS, volume 2691 of Lecture Notes
in Computer Science, pages 236–246. Springer.
Laporte, G. and Osman, I. H. (1995). Routing prob-
lems: A bibliography. Annals of Operations Research,
61(1):227–262.
Solomon, M. M. (1987). Algorithms for the vehicle rout-
ing and scheduling problems with time window con-
straints. Operations Research, 35:254–265.
Vickrey, W. (1961). Counterspeculation, auctions and com-
petitive sealed bids. Journal of Finance, 16:8–37.
ICAART 2012 - International Conference on Agents and Artificial Intelligence
90