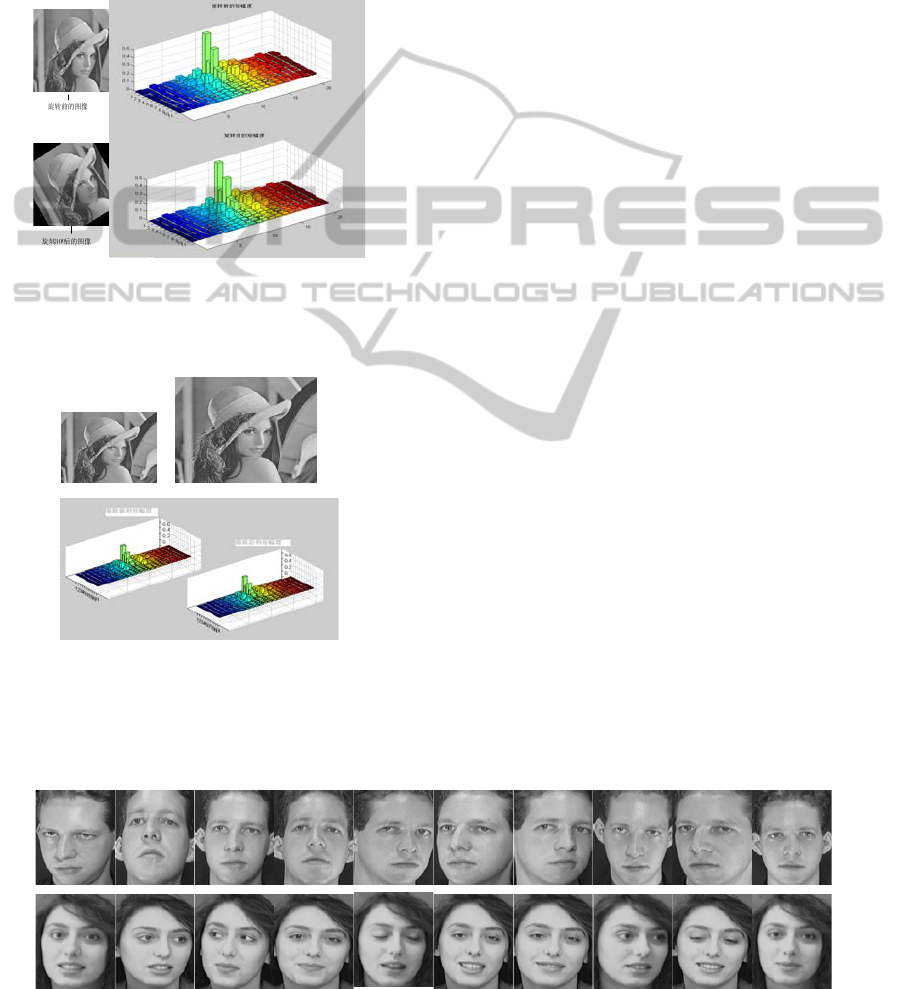
(Ping et al., 1748-1754). Fig.2 shows the
rotation-distorted invariant performance of Complex
Exponent Moments (CEMs): The line-up is original
image of Lena and its modular value distribution, the
line-down is rotated image of Lena and its modular
value distribution. From Fig.2 it can be seen that the
modular values of CEMs for rotated image are
invariant.
Figure 2: The rotated invariant performance of CEMs. The
line up is standart image and its CEMs modular value
distribution. The line down is rotated image and its CEMs
value distribution.
缩放前的图像 放大1.5倍后的图像
Figure 3: The scaling invariant performance of CEMs. The
left column is normal image and its CEMs modular value
distribution. The right column is scaled image and its
CEMs modular value distribution.
Fig.3 shows the scaling invariant performance of
modular value of CEMs
The left column is Lena normal image and
modular value distribution of its CEMs, the right
column is distorted image scaled 1.5 times and its
modular value distribution of its CEMs. From
Fig.3 it can be seen that the modular value of CEMs
of scaled image possesses the same modular value
distribution with the normal image.
5 APPLICATION OF CEMS FOR
PATTERN RECOGNITION
Using the CEMs of image to be feature vectors for
Suppot Vector Machine (SVM) algorithm pattern
recognition was performed for human face of 20
persons, for whom each one has 10 various visions and
different facial expression. Fig.4 shows the experiment
images of two persons of the recognized human faces.
Each face image was rotated for 10°、20°、30° and has
40 pieces of image for one person, and there are 800
pieces of variable image for 20 persons.
Take mass center of image to be original point of
the image coodinate system and calculate the CEMs
of the image via FFT algorithm. Using CEMs of the
image to be feature vectors of Support Vector
Machine (SVM), the human face recognition
experiment was performed. The 24 pieces of face
image for each person and 480 pieces of face image
for 20 persons were taken as trining sample image
set, and the other 320 pieces of face images were
taken as testing sample image set, and the one to
more SVM algorithm was applied in the experiment.
Table-1 shows the experiment result. From the data
of Table-1 it can be seen that taking 36 CEMs as
image feature vectors the recognition rate is the
highest, achieving 92.5%, as number of CEMs
increasing the recognition rate of image is
decreasing down, this is because of the over-learning
problem for Suport Vector Machine(SVM)
Figure 4: The various face expression for two persons in experiment, upper: face of male person; lower: face of female
person.
COMPLEX EXPONENT MOMENTS FFT ALGORITHM AND ITS APPLICATION
467