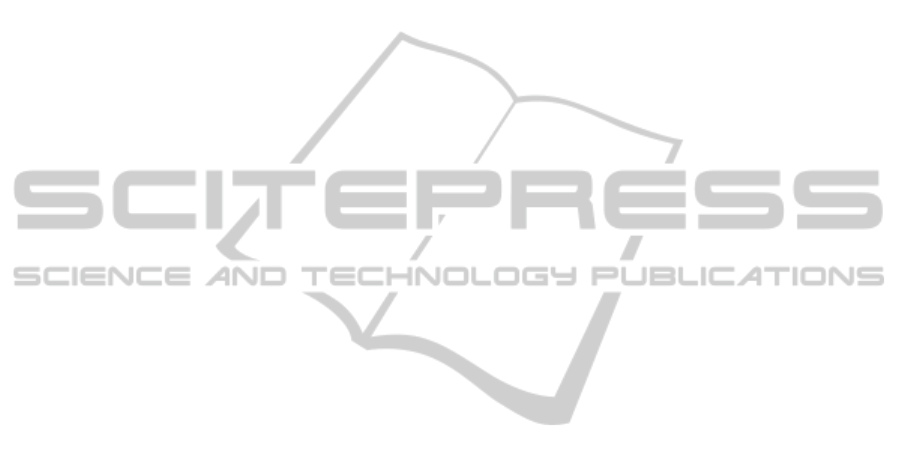
path. Not less important, it is necessary to consider
the time involved in each path and constraints such
as specific (and limited) time period in which the
garbage collection can be done. The last constraint
shows that we are solving a problem that can be clas-
sified as VRPTW.
VRPTW needs to satisfy a time window defined
for a customer i as [a
i
, b
i
], which expect to accom-
plish the job in a specific time interval. The starting
time cannot be previous to a
i
and the arrival time w
i
plus the time devoted to a particular customer i can-
not exceed b
i
. The central depot, represented by 0 is
also associated to a time window, which is defined as
[E, L], where E represents the time in which the trucks
begin to traverse their routes, and L the time in which
all trucks should be in the depot. If truck k arrives to a
customer i location in a time w
i
and satisfies w
i
< a
i
,
the truck k has to wait until w
i
≥ a
i
.
A good solution optimizes (minimizes) the sum of
costs involved in the K path considered for the vehi-
cles.
The cost associated to a path for a vehicle k is de-
termined by the sum of costs c
i j
corresponding to the
edges (i, j) that belong to the path. This solution must
satisfy the following constraints:
i) A customer is visited by, exactly, one vehicle,
ii) every path has only one edge from the depot, iii)
every node in the path has only one edge arriving to
it, and only one edge starting from it, iv) every path
has only one edge arriving to the depot, v) the sum
involving the customer’s attention time for customer i
and the time involved in going from i to j needs to be
less or equal to the time in which attention for a new
customer j begins, vi) the arrival time to a customer i
location, for one vehicle, needs to be associated to the
time interval defined for customer i, vii) starting time
from the depot, and arrival time to the depot need to
be considered in the interval associated to the depot
and viii) the sum of customer’s demands, for a partic-
ular path cannot exceed the vehicle capacity.
3 THE PROPOSAL
We propose to combine ACS with Tabu Search as fol-
lows: For each iteration in ACS, once the best route is
found by an ant colony, this route is improved through
2-opt. The route obtained in this way is used as input
data for applying the meta-heuristic Tabu Search. If
Tabu Search effectively improves the solution, it is re-
introduced into the colony, just before the process of
global pheromone updating.
Ant Colony System (ACS) was proposed by
Dorigo in (Dorigo and Stuetzle, 2004). As in other
ACO algorithms, ACS is a meta-heuristics inspired
in ants’ behavior when trying to find food. In the
collaborative behavior, communication among ants is
accomplished through a pheromone trail, that is se-
creted by the ants when moving from one point to
another, and that is used to influence the ants behav-
ior that contact this chemical factor. In this particular
work, we use ACS, that is characterized for having
a local and a global mechanism for updating the se-
creted artificial pheromone, allowing a search process
in which random aspects are more relevant.
Tabu Search is a meta-heuristic algorithm that can
be used for solving combinatorial optimization prob-
lems. Tabu search uses a local or neighborhood search
procedure to iteratively move from a solution x to a
solution x
0
in the neighborhood of x, until some stop-
ping criterion has been satisfied. To explore regions
of the search space that would be left unexplored by
the global search procedure, ACS in this case, Tabu
Search modifies the neighborhood structure of each
solution as the search progresses (Glover and Laguna,
1997).
When building a solution to the VRPTW, each ant
begins their path from the depot and visits different
customers, until the capacity of the vehicle is com-
pleted. Then the ant goes back to the depot forming
a path. This process is repeated until all customers
have been visited. In this way, the solution for one ant
builds as many path as times has started a search from
the depot.
ACS features are: i) Each time an ant choose a
customer, they have the possibility of doing it in a
probabilistic or deterministic way; this possibility is
given by a parameter called q
o
. ii) Pheromone up-
dating is accomplished through a global process that
considers the best solution that has been reached until
this moment. iii) Each ant diminishes a certain quan-
tity of pheromone each time it goes through an edge,
allowing this way that future ants can explore differ-
ent paths.
In ACS, when an ant that is in node i has to select
a new node, not previously visited, from a set N(i), it
considers the quantity of pheromone and the cost of
traversing an edge (i, j), for each j ∈ N(i). Besides
that, in this proposal two additional variables as pro-
posed in (Bullnheimer et al., 1997) were considered;
the first one is the time to arrive to the customer j
location, with respect to their time window (∆v) de-
scribed in equation (1), and the second is the wasted
capacity of a vehicle (∆c) described in equation (2).
In this way equation (3) describes the mechanism an
ant uses for selecting a node j.
ICAART 2012 - International Conference on Agents and Artificial Intelligence
470