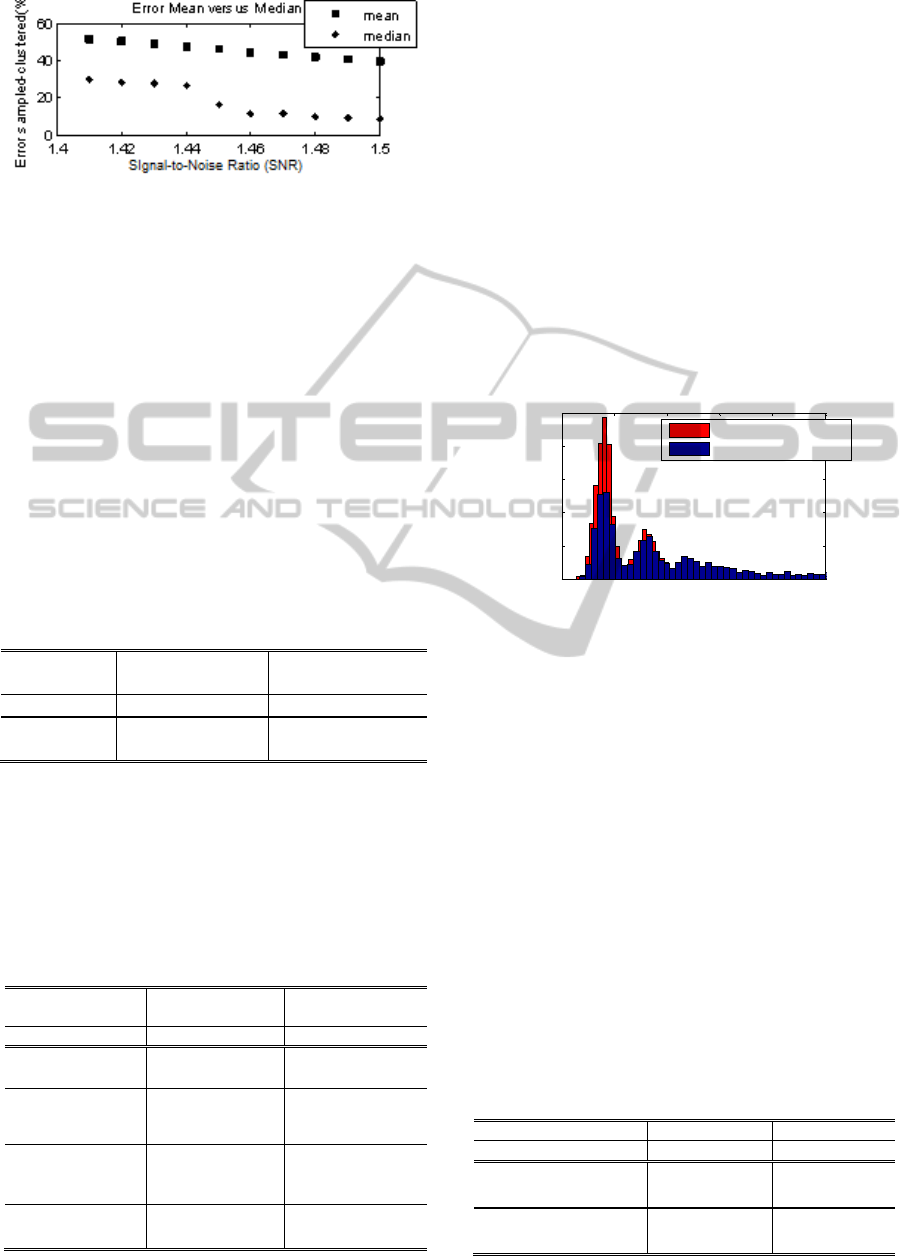
Figure 4: Error mean versus error median for signals with
different low SNRs.
C
V
>1 was reported as an indicator for bursting
activity (Kostal et al., 2007). In our simulations this
feature has higher values for signals with bursts,
approximating 1 or higher after clustering
substantiating this claim. As a consequence of low
SNR many spikes are not detected, hence the change
of the standard deviation and mean will lead to the
large deviations of the Cv. Nevertheless, it has
informative capacity indicating main features of
distribution (Table 1).
Table 1: Example of two normal distributions (with and
without bursts) corresponding to the two active neurons
recorded in one signal. C
V
is calculated for two models -
before and after clustering. The second column is a
repeated simulation with other values for the means of the
underlying distributions.
Mean (ms)
83,33
+bursts
125,00
75,00 +
bursts
133,33
Std (ms) 12,50 20,00 12,50 20,00
Cv,before_cl 0,60 0,17 0,45 0,15
Cv,after_cl 1,63 0,52 1,86 0,53
Spiking randomness indicates the variety of
spiking patterns. However, it showed large and
unpredictable errors, indicating little practical
usefulness. The mean error was 401,67 (±403,22) %.
Table 2: Examples of two normal timestamp distributions
(with and without bursts), selected for generating an
artificial signal. Bursting parameters are calculated for two
models - before (b_cl) and after clustering (a_cl).
Mean (ms)
100
+bursts
108,33
91,67 +
bursts
116,67
Std (ms) 12,50 20,00 12,50 20,00
Mod_burst, b_cl 0,31 0,00 0,22 0,00
Mod_burst, a_cl 0,29 0,02 0,22 0,02
Pause_index, b_cl 2,74 249,00 3,60
249,0
0
Pause_index_a_cl 2,22 13,93 2,57 10,79
Pause_ratio, b_cl 51,57 709,15 42,41
637,4
1
Pause_ratio, a_cl 27,04 79,18 21,88 55,57
Perc>5spikes, b_cl 3,96 0,00 3,30 0,00
Perc>5spikes, a_cl 3,30 0,00 2,82 0,00
Burst parameters can reveal the presence or
absence of bursts (Table 2). In this case both
features mod_burst and `percentage window > 5
spikes’ are larger than zero. On the other hand, the
values for pause_ratio and pause_index are smaller
than those values for signals without bursts. If all
these conditions are true, even modest bursting
activity of a neuron is always detected in our
simulations.
3.2 Overclustering
The mean and median proved to be significantly
different for the two clusters. Many missing
timestamps resulted in longer interspike intervals
(Figure 5), hence higher values for mean and median
compared to those of the underlying distribution.
Figure 5: Two overlaid ISIHs, originally assumed as
belonging to different clusters but having the same
underlying distribution; dominant peaks matching almost
perfectly.
Although not accurate in cases of individual
clusters, skewness and kurtosis proved to be good
indicators of overclustering. Values for the two
clusters are similar (example in Table 3), with
respectively differences of 15,61% and 18,98%. As
a comparison, these differences are at least twice as
large in cases of different distributions.
Fitting the ISIHs with analytical functions after
clustering could be another condition to decide if the
two clusters should be merged.
Table 3: To simulate overclustering, one original normal
distribution (orig_distr) is randomly split up into two
clusters. A certain percentage of timestamps is assigned to
the first cluster and the other timestamps to the second
cluster. Values for skewness and kurtosis are calculated
and compared. Two examples are given.
Mean (Std) (ms) 66,67 (12.5) 66.67 (12.5)
% cluster 1/ cluster 2 70 30 80 20
skewness, orig_distr 0.09 0.09
skewness, after_cl 2,17 1,81 2,24 2,02
kurtosis, orig_distr 3,19 3,19
kurtosis, after_cl 9,12 7,43 9,38 9,97
0 2000 4000 6000 8000 10000
0
50
100
150
200
250
samples
interspike interval histogram
ISIH 1 (60% of cluster)
ISIH 2 (40% of cluster)
BIOSIGNALS 2012 - International Conference on Bio-inspired Systems and Signal Processing
172