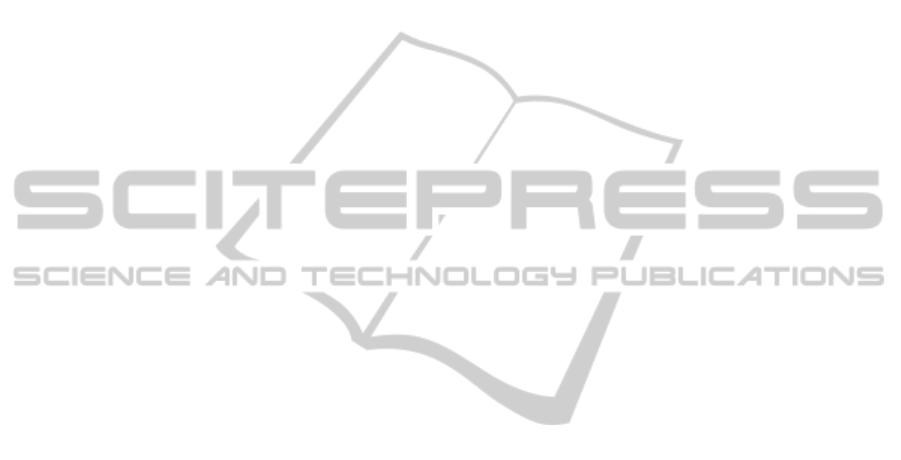
7 CONCLUSIONS AND
PERSPECTIVES
Based on both simulated and real data analyses, this
paper promotes the use of FLTMs as a simple and use-
ful framework for disease association detection in hu-
man genetics. Efficient capture of indirect genetic as-
sociation is achieved through two major reasons: (i)
the causal SNP ancestor nodes succeed in capturing
indirect associations with the phenotype; (ii) at the
opposite, the other latent nodes globally show very
weak associations. In other words, this property al-
lows to distinguish between true and false indirect ge-
netic associations.
The numbers of SNPs in the benchmarks were
limited. Nonetheless, this limitation is not a bias to
the sound characterization of the fading of informa-
tion in the FLTM hierarchies: bottom-up information
decays does concern the forest depth and does not in-
terfere with the forest width. It must be underlined
that our tests were not designed to meet the small
n, large p condition (many more variables (SNPs)
than subjects) as in genome-wide association studies
(GWASs). Again, this is not a bias to our study:
over thirty-six various scenarii, we have shown that
the overwhelming part (about three quarters) of false
positives confines in a unique tree, namely the one
harbouring the causal SNP (causal tree). In the con-
ditions of a GWAS, the forest width may well be far
larger than those observed in our tests, the false po-
sitives are expected to remain confined in the causal
tree, for the major part.
In a previous work, we have developped a scala-
ble FLTM learning algorithm, thus reaching orders of
magnitude consistent with GWAS demands (10
5
va-
riables, 2000 individuals). In addition to scalability,
data dimension reduction advocates the use of FLTM-
based modeling in GWASs: the issue of multiple hy-
pothesis testing in GWASs would be resolved by tes-
ting a low number of latent variables instead of a large
number of observed variables. However, before en-
visaging an FLTM-based GWAS, an inescapable pre-
requisite was testing whether the bottom-up informa-
tion fading through the forest would nevertheless al-
low reliable association detection. No less unavoida-
ble was the close examination of ratios of latent varia-
bles erroneously associated with the disease.
A precursory work to the GWAS concern, the
present contribution assets the soundness of the
FLTM model for association detection. Besides, we
have conceived a procedure to guarantee a given
family-wise (type I) error rate through the computa-
tion of layer-specific per-test error rates. The success-
ful test of our algorithm under a large spectrum of
conditions allows its integration in a GWAS tool.
REFERENCES
Ben-Dor, A., Shamir, R., and Yakhini, Z. (1999). Clustering
gene expression patterns. In Proc. of the 3rd annual
int. con. on Computational molecular biology, pages
33–42.
Chen, T., Zhang, N., Liu, T., Poon, K., and Wang, Y. (2011).
Model-based multidimensional clustering of categori-
cal data. In Artificial intelligence, in press.
Daly, M. J., Rioux, J. D., Schaffner, S. F., Hudson, T. J., and
Lander, E. S. (2001). High-resolution haplotype struc-
ture in the human genome. Nat. Genet., 29(2):229–
232.
Han, B., Park, M., and Chen, X. W. (2010). A Markov
blanket-based method for detecting causal SNPs in
GWAS. BMC Bioinformatics, 11(Suppl 3):S5+.
Harmeling, S. and Williams, C. K. I. (2011). Greedy learn-
ing of binary latent trees. IEEE Transactions on Pat-
tern Analysis and Machine Intelligence, 33(6):1087–
1097.
Hosking, L. K., Boyd, P. R., and Xu, C. F. e. a. (2002). Link-
age disequilibrium mapping identifies a 390 kb region
associated with CYP2D6 poor drug metabolising ac-
tivity. Pharmacogenomics J., 2(3):165–175.
Hwang, K.-B., Kim, B.-H., and Zhang, B.-T. (2006). Learn-
ing hierarchical bayesian networks for large-scale data
analysis. In ICONIP, pages 670–679.
Mourad, R., Sinoquet, C., and Leray, P. (2010). Learning
hierarchical Bayesian networks for genome-wide as-
sociation studies. In COMPSTAT, pages 549–556.
Mourad, R., Sinoquet, C., and Leray, P. (2011). A hierar-
chical Bayesian network approach for linkage dise-
quilibrium modeling and data-dimensionality reduc-
tion prior to genome-wide association studies. BMC
Bioinformatics, 12:16+.
Schwartz, G. (1978). Estimating the dimension of a model.
The Annals of Statistics, 6(2):461–464.
Spencer, C. C., Su, Z., Donnelly, P., and Marchini, J. (2009).
Designing genome-wide association studies: sample
size, power, imputation, and the choice of genotyping
chip. PLoS Genetics, 5(5):e1000477+.
Verzilli, C. J., Stallard, N., and Whittaker, J. C. (2006).
Bayesian graphical models for genome-wide associa-
tion studies. The American Journal of Human Gene-
tics, 79:100–112.
Wang, Y., Zhang, N. L., and Chen, T. (2008). Latent tree
models and approximate inference in Bayesian net-
works. Machine Learning, 32:879–900.
Zhang, N. L. (2004). Hierarchical latent class models for
cluster analysis. JMLR, 5:697–723.
Zhang, N. L. and Kocka, T. (2004). Efficient learning of
hierarchical latent class models. In ICTAI, pages 585–
593.
Zhang, Y. and Ji, L. (2009). Clustering of SNPs by a struc-
tural EM algorithm. In Int. Joint Conf. on Bioinfor-
matics, Systems Biology and Intelligent Computing,
pages 147–150.
BIOINFORMATICS 2012 - International Conference on Bioinformatics Models, Methods and Algorithms
14