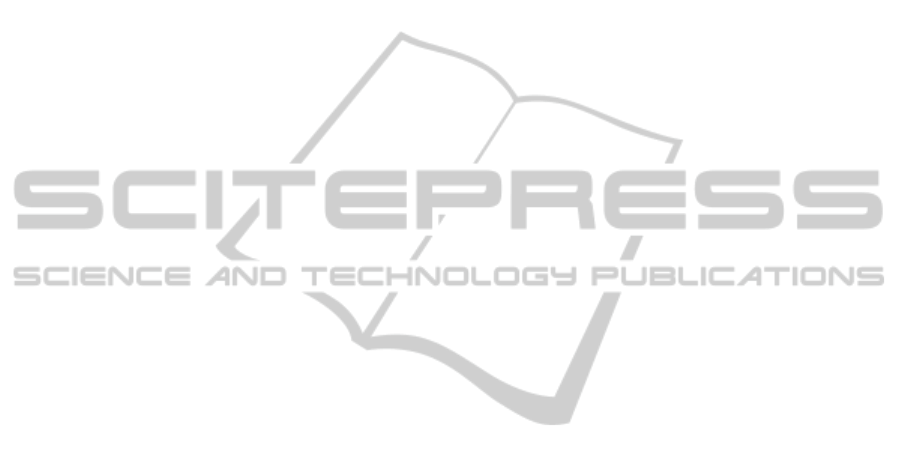
to be validated by comparing automatic contours
with highly detailed manual ones. Nevertheless, we
believe that a public database whose objective is to
be used in works with several different purposes,
should have as accurate ground truth as possible.
Notwithstanding the importance of the
digitalized databases, technological advances in
image acquisition devices for Radiology led to the
development of the Full Field Digital
Mammography (FFDM), where the digitalization-
related loss of information is absent. Thus, the
development of new databases that cover such
technological advances is a crucial step to develop
future CADs. Besides case selection and annotation
requirements, there are some authors (e.g. Oliver,
Freixenet et al., 2010) who referred that this issue
must also be taken into account when developing
new algorithms for CAD improvement. As noted in
this review, agreeing with previews works (Oliver et
al., 2010), there is no publicly available database
made with digital mammograms, all the images are
digitized.
We can conclude that researchers need a
database that fulfils all the mentioned requirements
in order to develop CAD systems. Having in
attention the actual state of the art on the breast
cancer research, databases with great variability of
cases, accurate annotations FFDM images are the
natural step in the evolution of mammographic
databases.
The requirements discussed at the Biomedical
Image Processing Meeting in 1993 need to be
reviewed and updated, as new paradigms and ideas
to increase algorithms performance are needed in
order to improve CAD schemes.
REFERENCES
American College of Radiology, 2003. American College
of Radiology Breast Imaging and Data System (BI-
RADS) 4th ed.
Eurostat, 2009. Health Statistics Atlas on Mortality in the
European Union.
Heath, M. et al., 1998. Current status of the Digital
Database for Screening Mammography. In Digital
Mammography. p. 457–460.
Jiang, L. et al., 2008. Automated Detection of Breast Mass
Spiculation Levels and Evaluation of Scheme
Performance. Academic Radiology, 15(12), p.1534-
1544.
Llobet, R., Paredes, R. and Pérez-Cortés, J.C., 2005.
Comparison of Feature Extraction Methods for Breast
Cancer Detection. In J. S. Marques, N. Pérez de la
Blanca, & P. Pina, orgs. Pattern Recognition and
Image Analysis. Lecture Notes in Computer Science.
Springer Berlin / Heidelberg, p. 495-502.
Matheus, B. R. N. and Schiabel, H., 2010. Online
Mammographic Images Database for Development
and Comparison of CAD Schemes. Journal of Digital
Imaging.
Nishikawa, R. M., 1997. Development of a Common
Database for Digital Mammography Research.
Nishikawa, R. M., 1998. Mammographic databases.
Breast Disease, 10(3-4), p.137-150.
Oliver, A., Freixenet, J., et al., 2010. A review of
automatic mass detection and segmentation in
mammographic images. Medical Image Analysis, 14,
p.87-110.
Oliver, A., Lladó, X., et al., 2010. A Statistical Approach
for Breast Density Segmentation. Journal of Digital
Imaging, 23, p.527-537.
Rangayyan, R. M., Mudigonda, N. and Desautels, J., 2000.
Boundary modelling and shape analysis methods for
classification of mammographic masses. Medical and
Biological Engineering and Computing, 38(5), p.487–
496.
Rojas Dominguez, A. and Nandi, A., 2007. Detection of
masses in mammograms using enhanced multilevel-
thresholding segmentation and region selection based
on rank. In Proceedings of the 5th IASTED
International Conference on Biomedical Engineering,
BioMED 2007. p. 370-375.
Song, Enmin et al., 2010. Hybrid Segmentation of Mass in
Mammograms Using Template Matching and
Dynamic Programming. Academic Radiology, 17(11),
p.1414-1424.
Suckling, J., 1994. The Mammographic Image Analysis
Society Digital Mammogram Database. In Exerpta
Medica. International Congress Series 1069. York,
England, p. 375–378.
Wang, D., Shi, L. and Ann Heng, P., 2009. Automatic
detection of breast cancers in mammograms using
structured support vector machines. Neurocomputing,
72(13-15), p.3296-3302.
World Health Organization, 2009. Fact sheet Nº 297:
Cancer.
Zheng, Bin et al., 2003. Mammography with Computer-
Aided Detection: Reproducibility Assessment - Initial
Experience. Radiology, 228, p.58-62.
Zheng, Bin et al., 2010. Computer-Aided Detection: The
Effect of Training Databases on Detection of Subtle
Breast Masses. Academic radiology, 17(11), p.1401-
1408.
HEALTHINF 2012 - International Conference on Health Informatics
340