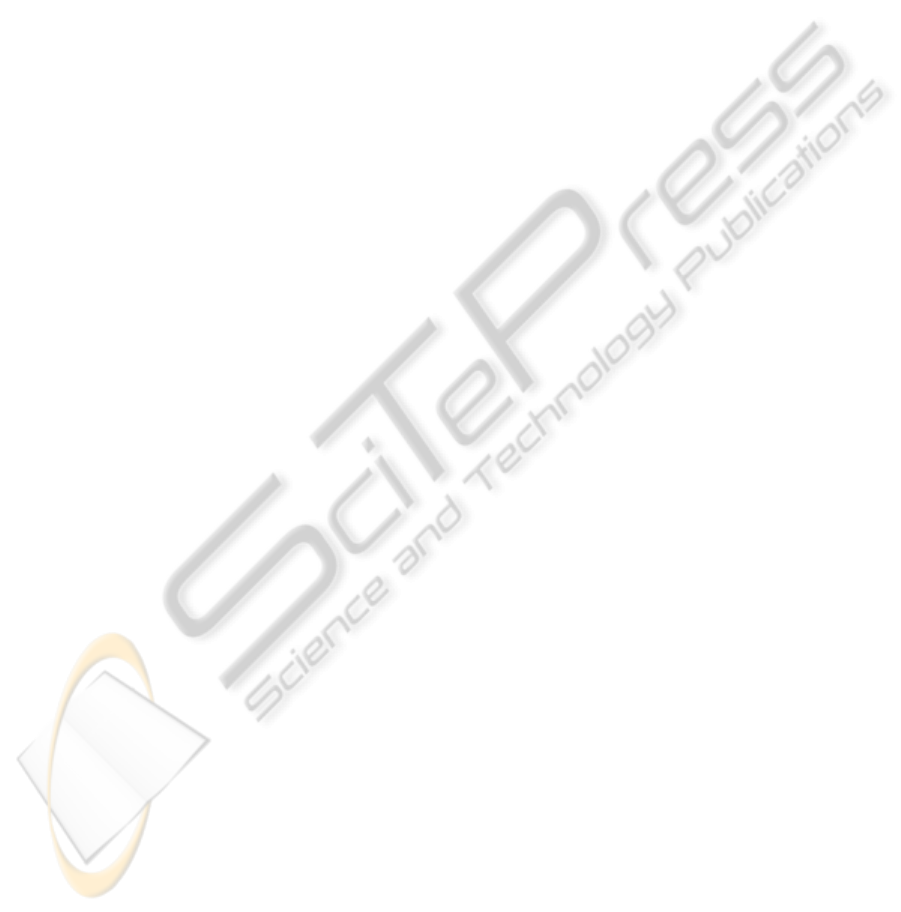
should be possible to determine whether an
individual learner is developing diagnostic skills
consistent with expectations and/or whether a
particular level of performance has been achieved
following exposure to specialist training. By
assessing four components of expert pattern
recognition, EXPERTise can also be used to identify
those component skills of pattern recognition that
experienced competent practitioners are struggling
to acquire. This information can then guide remedial
training efforts. Such cue-based approaches to
training have already met with some success in other
domains, including aviation (Wiggins & O’Hare,
2003) and mining (Blignaut, 1979).
The nature of the assessment tasks’ is such that
they assess independent skills, each of which
contribute to expert pattern recognition and
diagnosis. Therefore, if performance is weaker on
one or more of the tasks, it will be possible to
identify the specific area of deficiency and thereby
better target interventions. The application of this
strategy can be used to improve the efficiency and
the effectiveness of remedial medical training and,
as a consequence, minimize the costs associated
with training interventions.
5 CONCLUSIONS
The present study was designed to determine
whether four independent assessments of expert
pattern recognition could, collectively, distinguish
competent from expert practitioners within a
qualified sample of healthcare practitioners. Overall,
performance on all four assessment tasks
successfully differentiated the two groups, whereby
qualified staff could be divided into competent and
expert practitioners based on their capacity for
pattern recogniton, and cue extraction and
utilisation.
The successful replication of the results of
Loveday, et al. (submitted) in a dissimilar domain
demonstrates the utility of the EXPERTise tasks,
and the importance of pattern recognition in expert
performance generally. In time, it may also provide
a method for determining whether experienced
practitioners are developing expertise at a rate that is
consistent with their peers. Individuals’ who perform
at an unsatisfactory level may benefit from remedial
medical training. It is expected that this combination
of progressive assessment and remedial training may
reduce the rate of error in medicine through the
increased diagnostic expertise of practitioners.
ACKNOWLEDGEMENTS
This research was supported in part by grants from
the ‘Australian Research Council’ and TransGrid
Pty Ltd under the former’s Linkage Program (Grant
Number LP0884006).
REFERENCES
Blignaut, C. J. (1979). The perception of hazard: I. Hazard
analysis and the contribution of visual search to hazard
perception. Ergonomics, 22, 991 - 999.
Coderre, S., Mandin, H., Harasym, P. H., & Fick, G. H.
(2003). Diagnostic reasoning strategies and diagnostic
success. Medical Education, 37, 695 - 703.
Croskerry, P. (2009). A universal model of diagnostic
reasoning. Academic Medicine, 84(8), 1022 - 1028.
Gray, R. (2004). Attending to the execution of a complex
sensorimotor skill: Expertise, differences, choking,
and slumps. Journal of Experimental Psychology:
Applied, 10, 42 - 54.
Groves, M., O’Rourke, P., & Alexander, H. (2003). The
clinical reasoning characteristics of diagnostic experts.
Medical Teacher, 25, 308 - 313.
Jones, M. A. (1992). Clinical reasoning in manual therapy.
Physical therapy, 72, 875 - 884.
Lipshitz, R., Klein, G., Orasanu, J., & Salas, E. (2001).
Taking stock of naturalistic decision making. Journal
of behavioural decision making. Journal of
behavioural decision making, 14, 331 - 352.
Loveday, T., Wiggins, M., Harris, J., Smith, N., & O'Hare,
D. (submitted). An Objective Approach to Identifying
Diagnostic Expertise Amongst Power System
Controllers.
Morrison, B. W., Wiggins, M. W., Bond, N. W., & Tyler,
M. D. (2009). Examining cue recognition across
expertise using a computer-based task. Paper
presented at the NDM9, the 9th International
Conference on Naturalistic Decision Making, London.
Norman, G. R., Young, M., & Brooks, L. (2007). Non-
analytical models of clinical reasoning: the role of
experience. Medical education, 41(12), 1140 - 1145.
O’Hare, D., Mullen, N., Wiggins, M., & Molesworth, B.
(2008). Finding the Right Case: The Role of Predictive
Features in Memory for Aviation Accidents. Applied
cognitive psychology, 22, 1163 - 1180.
Rasmussen, J. (1983). Skills, rules, and knowledge:
signals, signs, and symbols, and other distinctions in
human performance models. IEEE Transactions on
Systems, Man, and Cybernetics, SMC-13, 257-266.
Ratcliff, R., & McKoon, G. (1995). Sequential effects in
lexical decision: Tests of compound cue retrieval
theory. Journal of Experimental Psychology:
Learning, Memory, and Cognition, 21, 1380 - 1388.
Schimdt, H. G., & Boshuizen, H. P. A. (1993). Acquiring
expertise in medicine. Educational psychology review,
3, 205 - 221.
IDENTIFYING DIAGNOSTIC EXPERTS - Measuring the Antecedents to Pattern Recognition
273