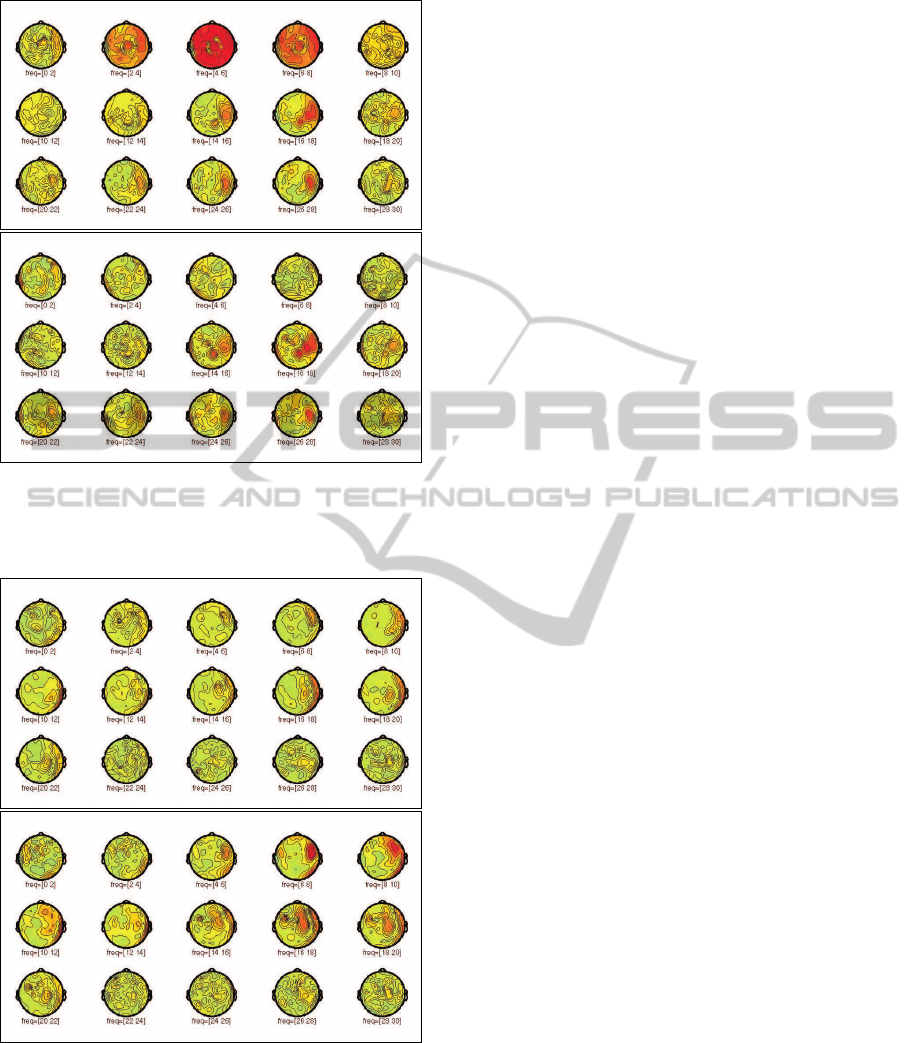
Figure 3: Coherence before (top plot) and after (bottom
plot) SSP in a PD patient between all magnetic channels
and contact 2 of the right electrode in the STN for frequency
bins of 2 Hz size from 0 to 40 Hz.
Figure 4: Coherence before (top plot) and after (bottom
plot) SSP in a dystonic patient between all magnetic chan-
nels and contact 3 of the right electrode in the GPi for fre-
quency bins of 2 Hz size from 0 to 40 Hz.
alternative to optimised beamforming (Litvak et al.,
2010) with regard to artefact suppression. In contrast
to beamforming, SSP does not require an electrical
source model. CCA removal not only suppresses co-
herences that are caused by the CCA, but also uncov-
ers coupling between the basal ganglia and cortical
brain regions, which were not visible before SSP. The
advantage of beamforming is that the location of the
coherent current in the brain is found. PCA on aCCA
followed by SSP could therefore be used as a prepro-
cessor for beamforming.
Future work has to investigate the reasons for the
CCA influencing coherences and statistical testing of
coherence differences before and after SSP (Maris
et al., 2007).
ACKNOWLEDGEMENTS
We would like to thank K. Obermayer for helpful dis-
cussions and support. A. B. is supported by a fellow-
ship from Dr. Robert Leven und Dr. Maria Leven-
Nievelstein-Stiftung.
REFERENCES
Jousm¨aki, V. and Hari, R. (1996). Cardiac artifacts in mag-
netoencephalogram. Journal of Clinical Neurophysi-
ology, 13:172–176.
K¨uhn, A. A., Williams, A., Kupsch, A., Limousin, P., Hariz,
M., Schneider, G. H., Yarrow, K., and Brown, P.
(2004). Event-related beta desynchronization in hu-
man subthalamic nucleus correlates with motor per-
formance. Brain, 127:735–746.
Litvak, V., Jha, A., Oostenveld, R., Barnes, G. R., Penny,
W. D., Zrinzo, L., Hariz, M. I., Limousin, P., Friston,
K. J., and Brown, P. (2010). Optimized beamforming
for simultaneous MEG and intracranial local field po-
tential recordings in deep brain stimulation patients.
Neuroimage, 50:1478–1588.
Maris, E., Schoffelen, J. M., and Fries, P. (2007). Nonpara-
metric statistical testing of coherence differences. J
Neurosci Methods, 163:161–175.
Silberstein, P., Kuehn, A. A., Kupsch, A., Trottenberg, T.,
Krauss, J. K., Woehrle, J. C., Mazzone, P., Insola, A.,
Lazzaro, V. D., Oliviero, A., Aziz, T., and Brown, P.
(2003). Patterning of globus pallidus local field poten-
tials differs between Parkinson’s disease and dystonia.
Brain, 126:2597–2608.
Uusitalo, M. A. and Ilmoniemi, R. J. (1997). Signal-space
projection method for separating MEG or EEG into
components. Medical and Biological Engineering and
Computing, 35:135–140.
BIOSIGNALS 2012 - International Conference on Bio-inspired Systems and Signal Processing
328