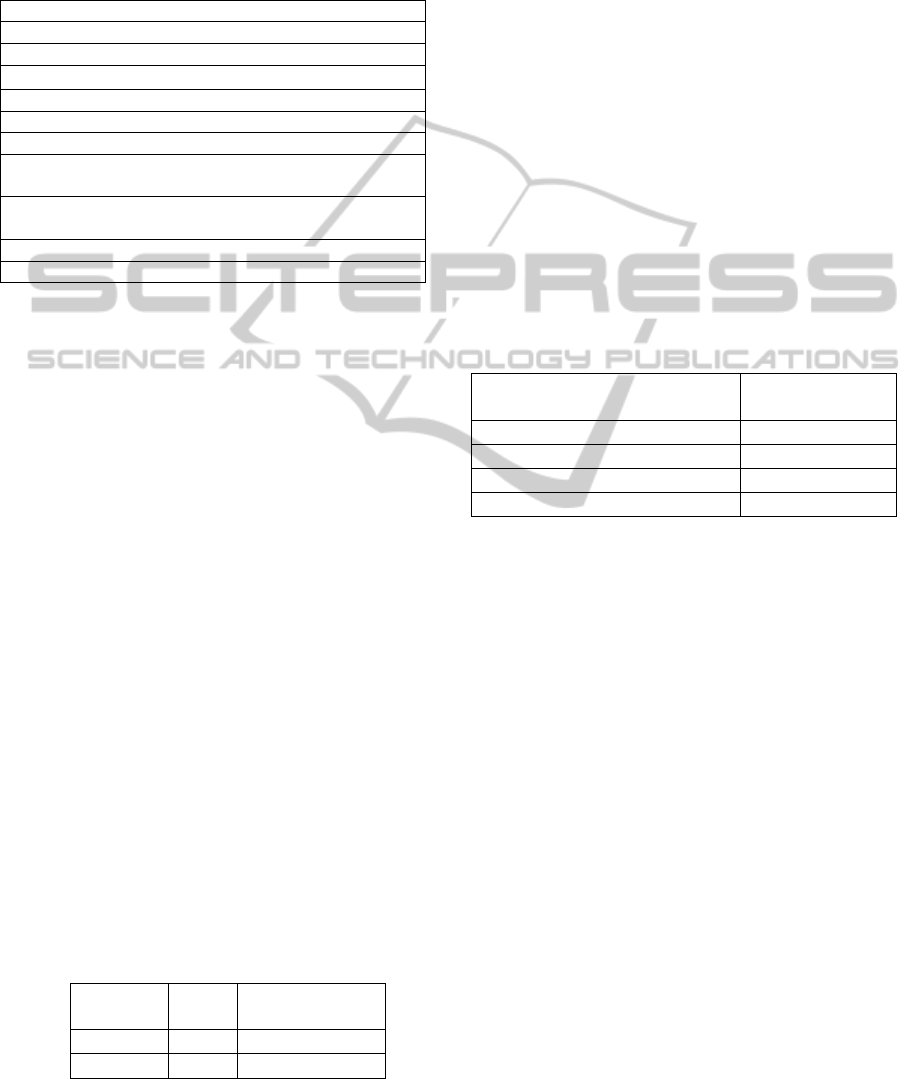
will be described in the next section,
Step 3: Next generation GA population is generated.
Step 4: Evaluation of performance of the classifier
for P300 classification.
Table 2: GA Parameters.
Coding of Genes Binary Coding
Population size 15
No. of genes 32 (numbers of WT features)
Reproduction Tournament selection
Crossover Two point crossover
Crossover rate 0.5
Mutation Random mutation
Convergence Max 800 generation of population
convergence
Population convergence if 80% of population one
are similar
Vigilance parameter Varied from 0 to 1 in steps of 0.1
Mutation rate 0.01
4.3 Performance Measure
The performance of the obtained PFC and GA based
feature selection was measured by a ten-fold cross
validation. Each individual was left out once, whilst
the other nine were applied for construction of the
classifier which was subsequently validated for the
unseen cases in the left-out sub-set. In each
generation during the running of GA, the maximum
of fitness function calculated. After producing 800
generation, the best chromosome was obtained and
its correspondence feature set determined. The
results of PFC and PFC by using GA as feature
selection algorithm (PFC+GA) for the ERP data
have been depicted in Table 3. The m parameter has
also been selected manually for finding the best
result. The best features selected using the GA
algorithm for the best accuracy.
For comparing the results we also applied LDA
as pure statistical classifier and fuzzy classifier
described in (Roubos, et all, 2001). and GA for
feature selection for both of these classifiers
(LDA+GA and Fuzzy+GA). The framework for
calculation of the results was the same as before, i.e.
based on ten-folds cross validation, and were
depicted in Table 4.
Table 3: Classification results for the PFC with and
without using GA measured by 10 fold cross validation.
Classifier
Type
m Classification
Accuracy
PFC 1.9 79.5%
PFC+GA 1.6 83.3%
5 CONCLUSIONS
In this paper time-frequency features, PFC, and GA
have been utilized for classification of P300 signals.
In order to generate the ERP, oddball paradigm has
been employed. The accuracy of classification for
the PFC together with wavelet features was 79%,
which was better than that of the LDA (purely
statistical classifier), 75%, and higher than that of
fuzzy classifier, which was 77.1%. Moreover, by
using GA to select the best set of wavelet features
the accuracy of classification increased. The
comparison between these classifiers when, GA is
used as feature selector also verified the superior
performance of the PFC with 83% accuracy. The
results justify that combination of the probabilistic
and fuzzy approaches is very useful for classification
of ERP and outperforms other classifiers solely
based on fuzzy or statistical properties.
Table 4: Performance of LDA and Fuzzy classifier
measured by 10 fold cross validation.
Classifier
Type
Classification
Accuracy
LDA 75%
LDA+GA 78.3%
Fuzzy (Roubos, et all, 2001). 77.1%
Fuzzy+GA 80.2%
REFERENCES
Kropotov, J., (2007). Quantitative EEG, Event-Related
Potentials and Neurotherapy, John Wiley.
Abootalebi, V., Moradi, M H., Khalilzadeh, M., (2009). A
new approach for EEG feature extraction in P300-
based lie detection, Computer Methods and Programs
in Biomedicine, vol. 94, pp. 48–5.
Abootalebi, V., Moradi, M H., Khalilzadeh, M., (2006). A
comparison of methods for ERP assessment in a P300-
based GKT, International Journal of
Psychophysiology, vol. 62, pp, 309–320.
Meyer, E., Lopes Da Silva, F., (2000). Electro-
encephalography Basic Principles, clinical
applications, and related fields, Lippincott Williams
and Wilkins, Baltimore, Maryland, USA. 4th Ed.
Meghdadi, AH., Akbarzadeh, M., (2001). Probabilistic
fuzzy logic and probabilistic fuzzy systems, The 10th
IEEE International Conference on Fuzzy Systems,
vol.3, pp.1127 –1130.
Meghdadi, AH., Akbarzadeh, M., (2003). Uncertainty
modelling through probabilistic fuzzy systems, Fourth
International Symposium on Uncertainty Modelling
and Analysis (ISUMA 2003), pp.56-61.
BIOSIGNALS 2012 - International Conference on Bio-inspired Systems and Signal Processing
178