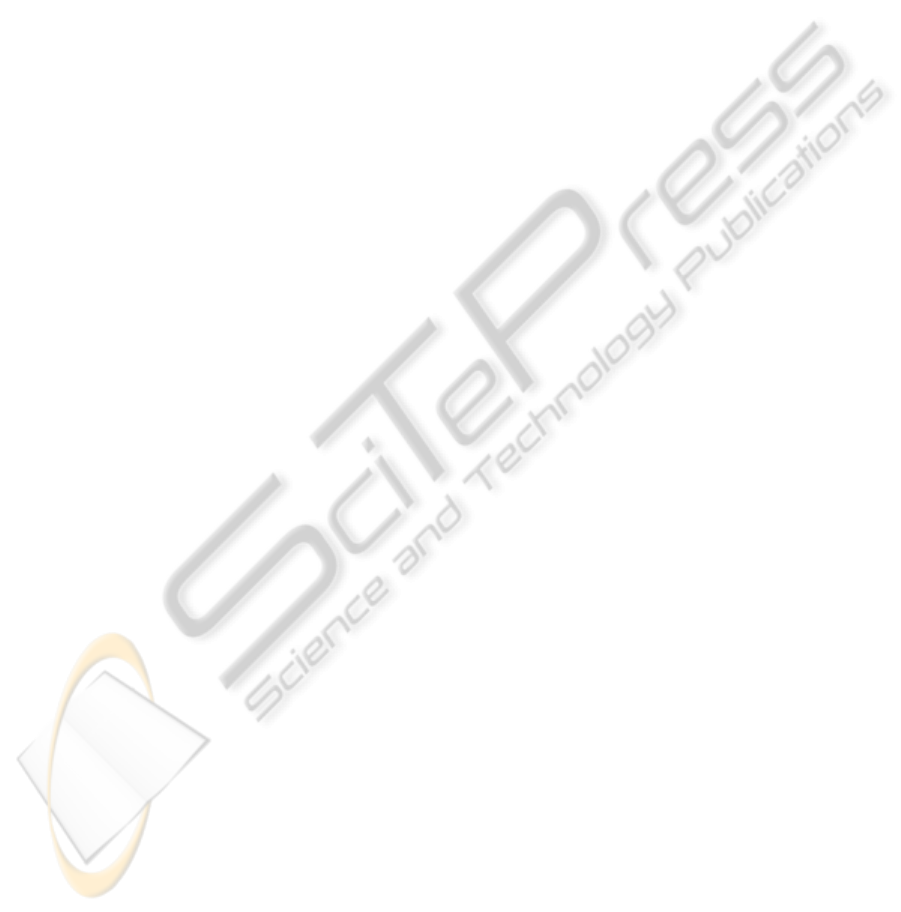
several reference images. Remaining outliers were re-
moved according to the local density (accumulator)
function (3). Further integration of color and con-
fidence information will concede an additional sta-
bility in the approach. The second application con-
cerns building modeling. The three-step procedure
of (Gross et al., 2005), with the two modifications
of DTM modeling by means of a robust cost func-
tion (L
1
-splines) and k-means based normal vector
clustering, also automatically processes dense point
clouds obtained by passive sensors from light UAVs
in nadir view. Therefore it is shown, that methods for
large scale range data with homegenously distributed
samples can be adapted to relatively low quality, se-
quentially obtained data of theoretical infinite length.
In the majority of cases, urban structures are recon-
structed well, as one can see from Fig. 5. To per-
form an accurate quantitative evaluation of complete-
ness and correctness of the procedure in comparison
with other procedures, such as (Rottensteiner, 2010),
reconstruction of either several high-resolution aerial
images or a larger video sequence must be performed.
These goals are currently being met, but they are be-
yond the scope of our work. Further consideration
of image information (e.g. segmentation) will be a
topic of future work. One can additionally filter out
vegetation: analyzing the reference image by means
of trained data is the only interactive part of the ap-
proach. The trees can then be found in larger regions
of the image (sequence); their height is given by the
depth map. Also here efforts must be made in future
by using color and gradient information in input im-
ages as well as confidence maps for better building
contour extraction and roof analysis.
REFERENCES
Baillard, C. and Zisserman, A. (2000). A plane-sweep strat-
egy for the 3D reconstruction of buildings from mul-
tiple images. ISPRS Congress and Exhibition in Ams-
terdam (Netherlands).
Bodensteiner, C., Hebel, M., and Arens, M. (2010). Ac-
curate single image multi-modal camera pose esti-
mation. Workshop on Reconstruction and Modeling
of Large-Scale 3D Virtual Environments. European
Conference on Computer Vision (ECCV).
Bulatov, D. (2008). Towards Euclidean reconstruction from
video sequences. Int. Conf.Computer Vision Theory
and Applications (2), pages 476–483.
Bulatov, D. and Lavery, J. (2010). Reconstruction and tex-
turing of 3D urban terrain from uncalibrated monocu-
lar images using L
1
Splines. Photogrammetric Engi-
neering and Remote Sensing, 75(10):439–450.
Bulatov, D., Wernerus, P., and Heipke, C. (2011). Multi-
view dense matching supported by triangular meshes.
ISPRS Journal of Photogrammetry and Remote Sens-
ing, accepted for publication.
Curless, B. and Levoy, M. (1996). A volumetric method for
building complex models from range images. Proc.
ACM SIGGRAPH, 30:303–312.
Fischer, A., Kolbe, T., Lang, F., Cremers, A., F¨orstner,
W., Pl¨umer, L., and Steinhage, V. (1998). Extracting
buildings from aerial images using hierarchical aggre-
gation in 2D and 3D. Computer Vision and Image
Understanding, 72(2):185–203.
Geibel, R. and Stilla, U. (2000). Segmentation of Laser-
altimeter data for building reconstruction: Compari-
son of different procedures. Int. Arch. of Photogram-
metry and Remote Sensing, 33 part B3:326–334.
Gross, H., Th¨onnessen, U., and v. Hansen, W. (2005). 3D-
Modeling of urban structures. Joint Workshop of IS-
PRS/DAGM Object Extraction for 3D City Models,
Road Databases, and Traffic Monitoring CMRT05,
Int. Arch. of Photogrammetry and Remote Sensing, 36,
Part 3W24:137–142.
Haala, N. (2005). Multi-Sensor-Photogrammetrie – Vi-
sion oder Wirklichkeit? Habilitation, Deutsche Geo-
d¨atische Kommission, M¨unchen, C589.
Harris, C. G. and Stevens, M. J. (1998). A combined corner
and edge detector. Proc. of 4th Alvey Vision Confer-
ence, pages 147–151.
Hartley, R. and Zisserman, A. (2000). Multiple view geom-
etry in computer vision. Cambridge University Press.
Hirschm¨uller, H. (2008). Stereo processing by semi-global
matching and mutual information. IEEE Transac-
tions on Pattern Analysis and Machine Intelligence,
30(2):328–341.
Kobbelt, L. and Botsch, M. (2004). A survey of point-
based techniques in computer graphics. Computers
& Graphics, 28(6):801–814.
Lin, Y.-M., Zhang, W., Wang, Y., Fang, S.-C., and Lavery, J.
E. (2006). Computationally efficient models of urban
and natural terrain by non-iterative domain decompo-
sition with l
1
-smoothing splines. Proc. 25th Army Sci-
ence Conf., Department of the Army, Washington DC,
USA.
Mayer, H. (1999). Automatic object extraction from aerial
imagery – A Survey focusing on buildings. Computer
Vision and Image Understanding, 74(2):139–149.
Mayer, H. and Bartelsen, J. (2008). Automated 3D re-
construction of urban areas from networks of wide-
baseline image sequences. The Int. Arch. of the Pho-
togrammetry, Remote Sensing and Spatial Informa-
tion Sciences, 37, Part B5:633–638.
Pock, T., Zebedin, L., and Bischof, H. (2011). Tgv-
fusion. Rain-bow of Computer Science. Springer-
Verlag, 6570/2011:245–258.
Rottensteiner, F. (2010). Roof plane segmentation by com-
bining multiple images and point clouds. Proc. of
Photogrammetric Computer Vision and Image Analy-
sis Conference, Int. Arch. of Photogrammetry and Re-
mote Sensing, 38, Part 3A:245–250.
Scharstein, D. and Szeliski, R. (2002). A Taxonomy
and Evaluation of Dense Two-frame Stereo Corres-
pondence Algorithms. International Journal of Com-
puter Vision, 47(1):7–42. Images and ground truth can
be downloaded at: http://bj.middlebury.edu/∼schar/
stereo/data/Tsukuba/.
ICPRAM 2012 - International Conference on Pattern Recognition Applications and Methods
280