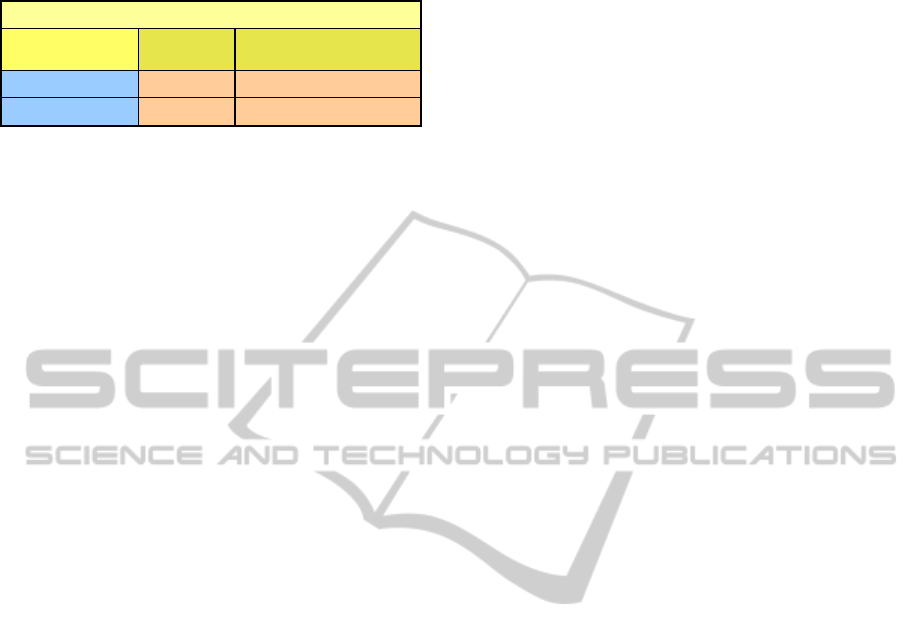
Table 1: Mean times of head and face images verification
during the experiment.
MEAN TIMES HEAD AND FACE IMAGES
Concept
Head
Time (sec.)
Face
Time (sec.)
Model Building 121.56 102.55
Test Verification 0.26 0.26
The best result obtained in the experiments with
thermal head images is 97.60% in relation to 88.20%
in thermal face verification. It can be observed that
the success rate with head images is higher in
comparison with facial images.
5 DISCUSSIONS AND
CONCLUSIONS
The main contribution of this work is the use, for the
first time, of a head/facial verification system based
on SIFT descriptors with a vocabulary tree. This
work is a preliminary step in the development of
face verification systems using SIFT descriptors in
thermal images of subjects.
The two variants compared in this work have
different performance for verification. The cause is
the amount of information provided by each format.
On the one hand, head images preserve important
discriminative characteristics about the original
thermal images for identifying a subject that facial
images do not include. On the other hand, it
becomes clear that in case of head images more
SIFT descriptors are produced and therefore, more
essential data for the verification process is
extracted. Additionally, faces of different subjects
have often common features that provide no
discriminant information.
As discriminative SIFT parameters are being
widely used, specialised methods can be developed
in future works for increasing dramatically the face
verification rates using thermal imaging systems.
As future work we would like to increase
considerably the size of database, and to include
outdoor images. The proposed approach will be
validated in this extended database.
ACKNOWLEDGEMENTS
This work was partially supported by “Cátedra
Telefónica - ULPGC 2010/11”, and partially
supported by research Project TEC2009-13141-
C033-01/TCM from Ministry of Science and
Innovation from Spanish Government.
Special thanks to Jaime Roberto Ticay Rivas for
their valuable help.
REFERENCES
Heo, J., Savvides, M., Vijayakumar, B. V. K., 2005.
Performance Evaluation of Face Recognition using
Visual and Thermal Imagery with Advanced
Correlation Filters. In CVPR’05, Proceedings of the
2005 IEEE Computer Society Conference on
Computer Vision and Pattern Recognition. pp.9-15.
ISSN: 1063-6919/05.
Huang, H., He, H., 2011. Super-Resolution Method for
Face Recognition Using Nonlinear Mappings on
Coherent Features. In Neural Networks, IEEE
Transactions. vol.22-1. pp.121–130. ISSN:1045-9227.
Imtiaz, H., Fattah, S.A., 2011. A wavelet-domain local
feature selection scheme for face recognition. In
ICCSP’11, 2011 International Conference on
Communications and Signal Processing. pp.448.
Kerala, India.
Jiang, L., Yeo, A., Nursalim, J., Wu, S., Jiang, X., Lu, Z.,
2004. Frontal Infrared Human Face Detection by
Distance From Centroide Method. In Proceedings of
2004 International Symposium on Intelligent
Multimedia, Video and Speech Processing. pp.41-44.
Hong Kong.
Jian-Gang, W., Jun, L., Wei-Yun, Y., Sung, E., 2010.
Boosting dense SIFT descriptors and shape contexts of
face images for gender recognition. In CVPRW’10,
2010 IEEE Computer Society Conference on
Computer Vision and Pattern Recognition Workshops.
pp.96-102.
Lowe, D. G., 1999. Object recognition from local scale-
invariant features. In ICIP’99, Proceedings of the
Seventh IEEE International Conference on Computer
Vision. vol.2. pp.1150-1157.
Lowe, D. G., 2004. Distinctive image features from scale-
invariant keypoints. In International Journal of
Computer Vision. vol.60-2 (2004). pp.91-110.
Majumdar, A., Ward, R.K., 2009. Discriminative SIFT
features for face recognition. In CCECE '09, 2009
Canadian Conference on Electrical and Computer
Engineering. pp.27-30.
Mikolajczyk, K., 2002. Detection of local features
invariant to affine transformations, Ph.D. thesis.
Institut National Polytechnique de Grenoble, France.
Nister, D., Stewenius, H., 2006. Scalable Recognition with
a Vocabulary Tree. In CVPR’06, 2006 IEEE Computer
Society Conference on Computer Vision and Pattern
Recognition. vol.2. pp.2161- 2168.
Ober, S., Winter, M., Arth, C., Bischof, H., 2007. Dual-
Layer Visual Vocabulary Tree Hypotheses for Object
Recognition. In ICIP’07, 2007 IEEE International
Conference on Image Processing. vol.6. pp.VI-345-
VI-348.
BIOSIGNALS 2012 - International Conference on Bio-inspired Systems and Signal Processing
480