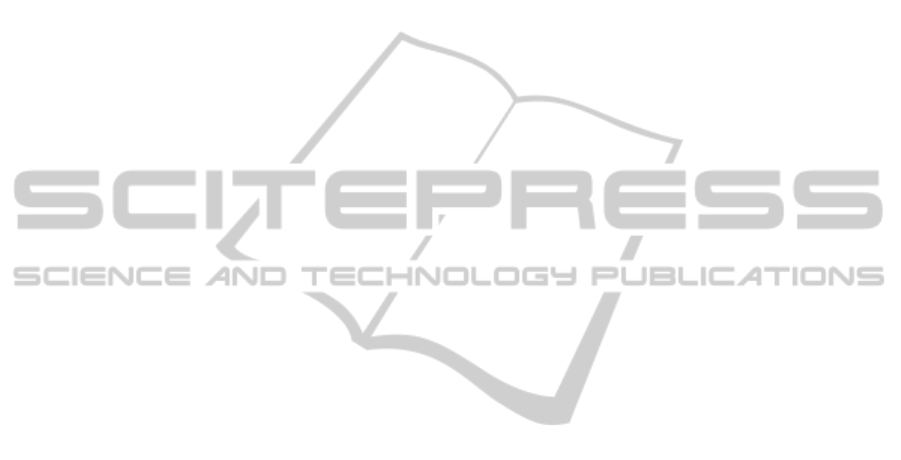
5 CONCLUSIONS
We have designed a 3D individual map able to
gather the features of the more classic genotype-
based risk maps with the more recent and accurate
haplotype-based risk maps. We have also developed
3DRiskMapper, a software application able to build a
3D risk map and provide the user with a virtual reality
interface so that they can get immerse in these usually
very large maps to explore them.
3D risk maps combine haplotype and genotype in-
formation in order to identify risk loci and therefore
the risk a individual has to a disease. They constitute a
a purelly visual tool provided to biomedical analysts.
We believe this tool is very important to reduce the
complexity in accessing, analysing and manipulating
result data from association studies.
As a future work, 3DRiskMapper may be en-
hanced with a more intuitive user interface, so that
users can interact in a very natural way, i.e. with their
own hands to unroll and rotate the helixes with the
information of risk loci. Maps enriched with infor-
mation from different annotation sources may fully
benefit of this 3D immersive feature.
6 WEB RESOURCES
The software is developed as open code under GNU
Public License 3.0 and can be downloaded from
http://bios.ugr.es/3DRiskMapper.
ACKNOWLEDGEMENTS
The authors were supported by the Spanish Research
Program under project TIN2010-20900-C04, the An-
dalusian Research Program under project P08-TIC-
03717 and the European Regional Development Fund
(ERDF).
REFERENCES
Abad-Grau, M., Medina-Medina, N., Masegosa, A., and
Moral, S. (2011a). Haplotype-based classifiers to pre-
dict individual susceptibility to complex diseases. In
Proceedings of BIOINFORMATICS 2012 - Interna-
tional Conference on bioinformatics Models, Methods
and Algorithms, volume 1.
Abad-Grau, M., Medina-Medina, N., Montes-Soldado, R.,
Matesanz, F., and Bafna, V. (2011b). Sample repro-
ducibility of genetic association using different multi-
marker tdts in genome-wide association studies: Char-
acterization and a new approach. PLoS ONE, ac-
cepted.
Evans, D., Visscher, P., and Wray, N. (2009). Harness-
ing the information contained within genome-wide as-
sociation studies to improve individual prediction of
complex disease risk. Human Molecular Genetics,
18:3525–31.
(IMSGC), I. M. S. G. C. (2010). Evidence for poly-
genic susceptibility to multiple sclerosis - the shape
of things to come. Am J Hum Genet, 86:621–5.
‘International Multiple Sclerosis Genetics Consortium’,
D. H., Compston, A., Sawcerand, S., Lander, E., Daly,
M., Jager, P. D., de Bakker, P., Gabriel, S., Mirel, D.,
Ivinsonand, A., Pericak-Vance, M., Gregory, S., Ri-
oux, J., McCauley, J., Haines, J., Barcellos, L., Cree,
B., Oksenberg, J., and Hauser, S. (2007). Risk alle-
les for multiple sclerosis identified by a genomewide
study. New England Journal of Medicine, 357(9):851–
62.
Jager, P. D., Chibnik, L., Cui, J., Reischl, J., Lehr, S., Si-
mon, K., Aubin, C., Bauer, D., Heubach, J., Sand-
brink, R., Tyblova, M., Lelkova, P., ’Steering com-
mittee of the BENEFIT study, committee of the BE-
YOND study’, S., committee of the LTF study’, S.,
committee of the CCR1 study’, S., E, E. H., Pohl, C.,
Horakova, D., Ascherio, A., Hafler, D., and Karlson.,
E. (2009). Integration of genetic risk factors into a
clinical algorithm for multiple sclerosis susceptibil-
ity: a weighted genetic risk score. Lancet Neurol.,
8(12):1111–9.
Sebastiani, P., Abad-Grau, M. M., and Ramoni, M. F.
(2010). Data mining and knowledge discovery hand-
book, Oded Maimon and Lior Rokach (eds.), chapter
Bayesian Networks, pages 175–208. Springer.
Sebastiani, P., Ramoni, M. F., Nolan, V., Baldwin, C. T., and
Steinberg, M. H. (2005). Genetic dissection and prog-
nostic modeling of overt stroke in sickle cell anemia.
Nature Genetics, 37:435–440.
Torres-S
´
anchez, S., Medina-Medina, N., Montes-Soldado,
R., Masegosa, A. R., and Abad-Grau, M. M. (2011).
Riskoweb: Web-based genetic profiling to complex
disease using genome-wide snp markers. In Rocha,
M. P., Corchado, J. M., Fdez-Riverola, F., and Va-
lencia, A., editors, Proceedings of the 5th Interna-
tional Conference on Practical Applications of Com-
putational Biology & Bioinformatics (PACBB 2011),
volume 1, pages 1–8.
Wang, J., D, D. P., Jager, P. D., Pelletier, D., de Bakker,
P., Kappos, L., Polman, C., ‘Australian, (ANZgene)’,
N. Z. M. S. G. C., Chibnik, L., Hafler, D., Matthews,
P., Hauser, S., Baranzini, S., and Oksenberg, J.
(2011). Modeling the cumulative genetic risk for
multiple sclerosis from genome-wide association data.
Genome Medicine, 3:3.
Wray, N., Goddard, M., and Visscher, P. (2003). Predic-
tion of individual genetic risk to disease from genome-
wide association studies. Genome Research, 17:1520–
28.
BIOINFORMATICS 2012 - International Conference on Bioinformatics Models, Methods and Algorithms
280